Let's go on a tour of cutting-edge tech that helps machines make their own art and stories!
AI can now craft realistic photos, write poems, and more - it's like magic!
In this beginner guide, we'll look at GANs, autoencoders, language models and other tools.
You'll see how each works and what types of content it makes best. We'll also check out real examples from art to science.
By the end, you'll understand how generative AI can imagine endless new things. What are you waiting for?!
Join us to discover the future of creation as we learn what it means to give computers the power of imagination.
First, let's start with what a generative model is.
What are Generative models?
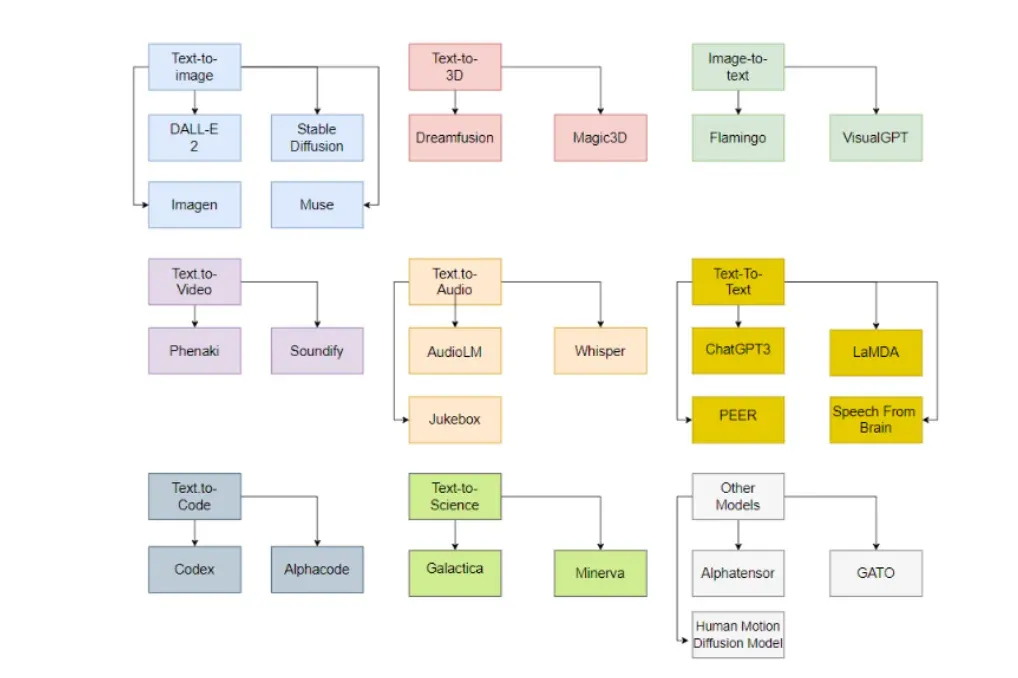
Generative models are a type of artificial intelligence that can create new images, text, audio, and more. Instead of just predicting things, they can generate brand-new creative outputs.
An example is image generators that can make realistic photos of people even though they were never shown those exact people before.
They learn patterns from huge amounts of data and use that to generate new things following the same patterns.
Things like text generators for writing, image creators, music makers, and more are all examples of generative AI.
They are changing things like art and design by automatically generating new creative works.
Now, let us see what are the types of generative AI models.
Types of Generative AI Models
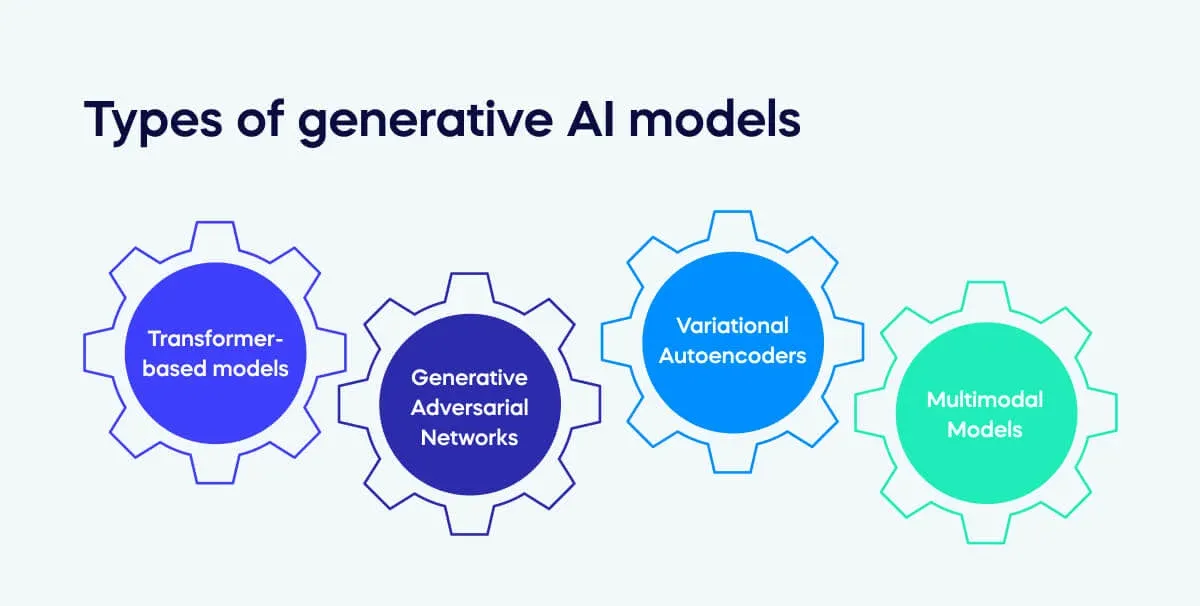
Each type of generative AI model has its strengths and applications. The choice of the model depends on the specific problem domain and the characteristics desired in the generated content.
Here are the types of generative models:
Variational Autoencoders (VAEs)
VAEs are generative AI models that utilize unsupervised learning to encode and decode data.
They consist of an encoder network that maps input data to a latent space and a decoder network that reconstructs the data from the latent space.
VAEs are designed to learn the underlying distribution of input data and generate new samples by sampling from the learned latent space.
They are widely used in tasks like image and text generation, as well as data synthesis for training other models.
Generative Adversarial Networks (GANs)
GANs are generative AI models that employ a game-theoretic approach.
They consist of a generator network that produces synthetic data and a discriminator network that distinguishes between real and generated samples.
The two networks are trained adversarially, with the generator trying to generate realistic data to fool the discriminator.
GANs have shown remarkable results in various domains, including image and video generation, transfer learning, and data augmentation.
Autoregressive Models
Autoregressive models are sequential generative AI models that predict the probability distribution of the next element in a sequence, conditioned on the previous elements.
These models generate data by sampling from the predicted distribution and iteratively generating the next element of the sequence.
Autoregressive models are commonly used for tasks like text generation, speech synthesis, and time series prediction.
Flow-based Models
Flow-based models are generative AI models that learn the data distribution by reparameterizing it as a series of invertible transformations.
These models determine a mapping from a simple distribution, such as a Gaussian, to the target distribution. By applying inverse transformations, flow-based models can generate new samples from the target distribution.
They have shown promise in domains like image generation, where they can generate high-quality images with controllable attributes.
After looking into the types let us see the capabilities and applications of generative models.
Suggested Reading:
Capabilities and Applications of Generative AI Models
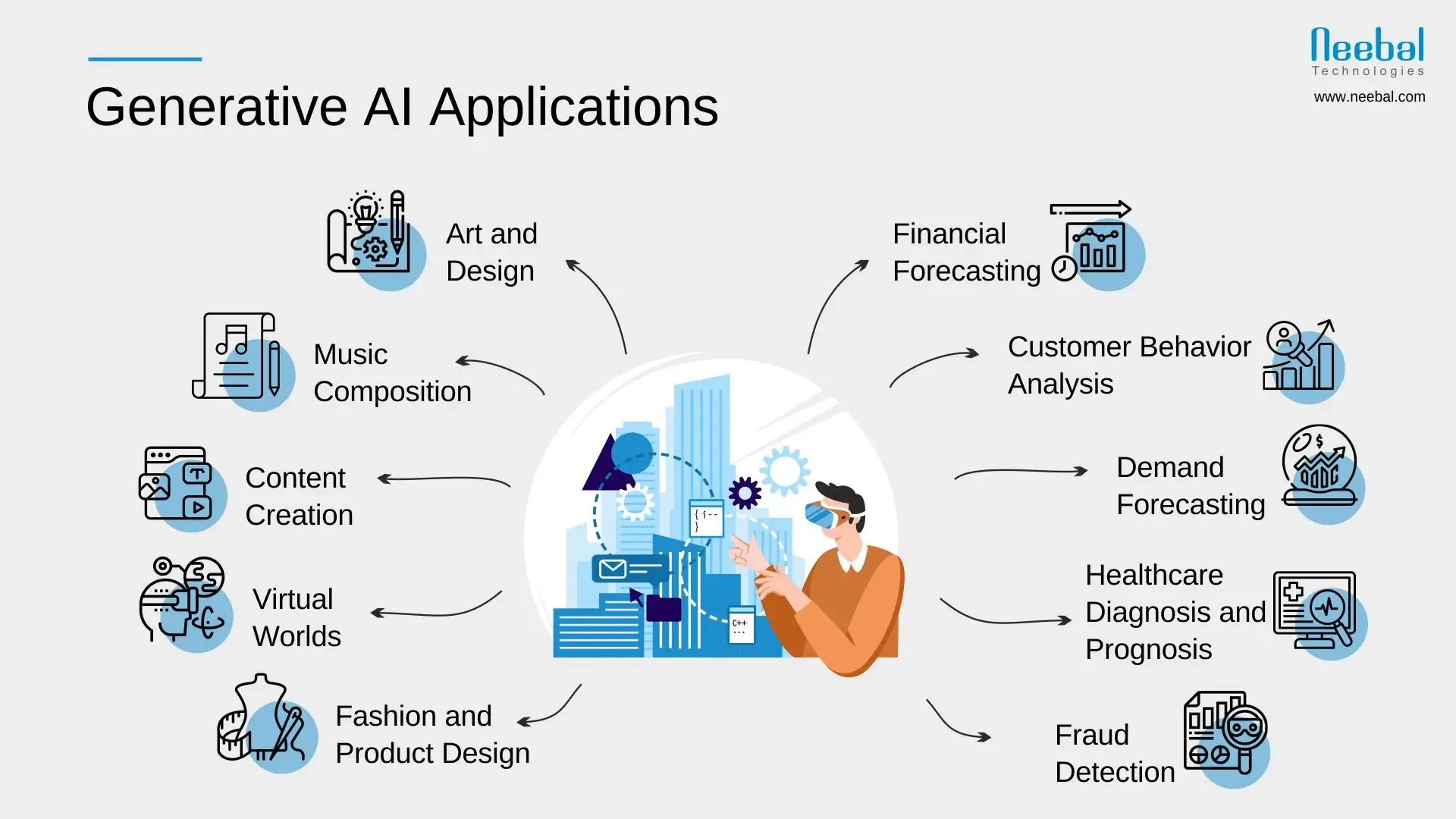
Generative AI models continue to make significant strides in their capabilities and applications.
From generating realistic images and coherent text to composing music and augmenting datasets, these models offer a wide range of creative and practical applications.
They have the potential to enhance various industries and domains, opening up new avenues for human-AI collaboration and innovation.
Image Generation
Generative AI models, such as GANs and VAEs, have significantly advanced in generating realistic and high-quality images.
These models can learn the underlying distribution of a dataset and generate novel images that closely resemble the original data.
Image generation has applications in various fields, from generating synthetic images for computer graphics and virtual reality to aiding artists and designers in generating new visual concepts.
Text Generation
Text generation using generative AI models enables machines to create coherent and contextually relevant text content.
Like the transformer-based models used in natural language processing, autoregressive models have successfully generated human-like text.
Text generation models have applications in chatbots, virtual assistants, content creation, and machine translation.
And, taking your first step towards Generative AI-powered chatbots isn't that tough. Meet BotPenguin- the home of chatbot solutions. With all the heavy work of chatbot development already done for you, move forward to setting up a top-notch AI chatbot for your business with features like:
- Marketing Automation
- WhatsApp Automation
- Customer Support
- Lead Generation
- Facebook Automation
- Appointment Booking
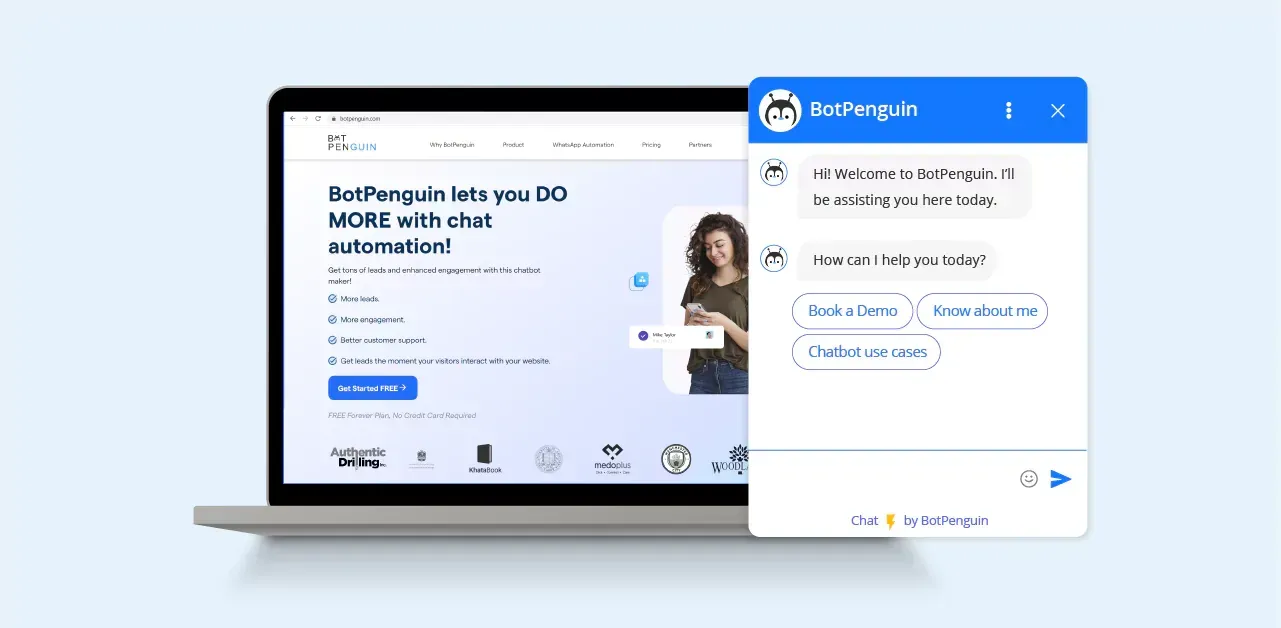
Music Generation
Generative AI models have also succeeded in generating music that mimics different styles and genres.
By training on a large dataset of musical compositions, models like recurrent neural networks (RNNs) or autoregressive models can generate original melodies, harmonies, and even lyrics.
Music generation has applications in entertainment, creative production, and music composition assistance tools.
Data Augmentation
Generative AI models can augment datasets by generating synthetic data similar to the original dataset.
This can help address challenges related to training data scarcity and imbalance. By generating additional samples, generative models can improve the generalization and robustness of machine learning models.
Data augmentation is particularly useful in computer vision, natural language processing, and medical imaging.
Challenges in Generative AI models
Addressing the challenges of training instability and mode collapse requires ongoing research and development of improved algorithms and techniques.
Ethical considerations must also be carefully addressed by adopting ethical guidelines, public awareness, and regulatory frameworks.
By overcoming these challenges and embracing responsible practices, generative AI models can continue to advance while serving as powerful tools for creativity, innovation, and problem-solving.
Training Instability
One of the primary challenges in generative AI is training instability.
Generative models, such as GANs, often require a delicate balance between the generator and discriminator networks to converge to an optimal solution.
However, finding this balance can take time and effort, resulting in training instability. Training instability can lead to difficulties in achieving convergence, slow training progress, and mode collapse, where the generator produces limited and repetitive outputs.
Various techniques, such as adjusting learning rates, network architectures, and regularization methods, have been developed to address training instability in generative AI.
Mode Collapse
Mode collapse is a specific manifestation of training instability in generative AI models.
It occurs when the generator of a GAN fails to explore the entire distribution of the training data and produces limited variations.
In other words, the generator collapses to generate a few representative samples, disregarding the full diversity of the target distribution.
Mode collapse hampers the model's ability to generate diverse and novel samples.
Researchers have proposed several techniques, such as adding diversity-promoting objectives or regularization terms, to mitigate mode collapse and encourage the generation of a more diverse range of outputs.
Ethical Considerations
As generative AI models become more powerful and capable, they raise important ethical considerations.
One of the key concerns is the potential for misuse and the creation of deepfakes or fake content that can spread misinformation or be used for malicious purposes.
These AI-generated fakes have the potential to deceive individuals and undermine trust.
Now after seeing the challenges it's time for future aspects of generative models.
Suggested Reading:
Understanding Generative and Discriminative Models: Which One Should You Choose?
Recent Advances and Future Prospects
As these models become more accessible and user-friendly, they can empower individuals and organizations to use the power of AI in various applications, positively impacting industries, innovation, and society as a whole.
OpenAI's GPT
OpenAI's GPT (Generative Pre-trained Transformer) is a language model that has garnered significant attention for its impressive capabilities in natural language understanding and generation.
With a staggering 175 billion parameters, GPT has demonstrated remarkable proficiency in tasks like text completion, language translation, and even creative writing.
This model utilizes unsupervised learning on a massive scale and has set new benchmarks for generative AI. GPT represents a culmination of recent advances in transformer-based architectures and has sparked enormous interest in natural language processing.
Potential Applications in Various Fields
The emergence of models like GPT-3 opens up various applications.
In industries such as customer support and chatbots, GPT-3 can provide more sophisticated and human-like interactions. It can also automate tasks like content generation, writing code, or even creating entire websites.
In healthcare, GPT-3 can assist with medical diagnosis and treatment recommendations based on analyzing patient data and medical literature.
Furthermore, GPT-3 can aid in language translation, sentiment analysis, and information retrieval, making it valuable in journalism, marketing, and research.
Impact on Industries and Society
The advancements in generative AI, exemplified by models like GPT-3, can potentially transform industries and society.
By automating tasks that previously required human effort, these models can enhance productivity and streamline workflows.
They can revolutionize content creation, freeing up time for creatives and enabling the generation of personalized and tailored content at scale. The impact extends to customer service, where GPT-3 can provide more efficient and satisfying interactions.
However, the widespread adoption of such models also raises concerns about job displacement and the need to reevaluate workforce skills and job market dynamics.
Conclusion
Generative AI models are transforming content creation through their ability to generate images, text, music, and more autonomously.
As these technologies continue advancing exponentially, the possibilities seem endless. Shortly, generative AI may assist with or automate many tasks across industries.
However, developing these systems responsibly and ensuring their fair, safe, and ethical use will be crucial.
With openness, oversight, and care for human values, generative AI has the potential to be a tool that empowers creativity for the benefit of all.
Suggested Reading:
Frequently Asked Questions (FAQs)
What are generative AI models?
Generative AI models are algorithms that generate new data, such as images, texts, or sounds, based on patterns learned from existing data. These models use techniques like neural networks to learn and mimic the underlying distribution of the training data.
How do generative AI models work?
Generative AI models, like GANs or VAEs, are trained on a large dataset and learn to generate new data by capturing the statistical patterns present in the training data. They use various techniques, such as transforming noise into meaningful output or encoding meaningful input into desired outputs.
What are the applications of generative AI models?
Generative AI models find applications in areas like image synthesis, text generation, music composition, and even drug discovery. They can be used to generate realistic images, create virtual characters, assist in content creation, and support creative tasks in multiple domains.
What is the difference between generative AI models and discriminative AI models?
Generative AI models focus on generating new data, while discriminative models focus on classification tasks by distinguishing among existing data. Generative models learn to capture the underlying distribution, whereas discriminative models learn to distinguish between different classes or categories.
What are the challenges faced in training generative AI models?
Training generative AI models can be challenging due to issues like training instability and mode collapse. These challenges can impact convergence, slow down training progress, and lead to the generation of limited and repetitive outputs.
How can generative AI models be used responsibly?
To ensure responsible use of generative AI models, ethical considerations should be taken into account. Guidelines and regulations can be implemented to address concerns related to deep fakes, privacy, bias, and content integrity, promoting transparency, fairness, and accountability in their development and deployment.