Introduction
Generative AI has revolutionized the landscape of AI chatbots and conversational AI, ushering in a new era of more natural and context-aware interactions.
According to a 2023 report by Gartner, by 2025, generative AI models will be a part of 30% of all AI software revenue, highlighting its growing importance in various applications, including chatbots.
This surge in generative AI applications has significantly enhanced the capabilities of language models used in conversational interfaces.
The integration of generative AI models into chatbots has led to remarkable improvements in their ability to understand and respond to user queries.
A study by Juniper Research predicts that by 2026, conversational AI powered by generative models will help banks save $7.3 billion globally, showcasing the technology's potential across industries.
These advanced AI chatbots can now generate human-like responses, adapt to context, and even engage in creative problem-solving.
Generative AI examples in chatbot technology are numerous and diverse. From customer service bots that can empathize and provide personalized solutions to virtual assistants capable of engaging in complex dialogues, the applications are transforming user experiences.
A 2023 survey by MIT Technology Review Insights found that 72% of business leaders believe generative AI will have a significant impact on their industry within the next two years.
As we delve deeper into how generative AI models enhance chatbot conversations, it's clear that this technology is not just improving existing systems but redefining the very nature of human-machine interactions.
Chatbots are everywhere these days, from customer service to online shopping assistants. They have changed how businesses interact with users. But not all chatbots are the same. Understanding the different types of chatbots and how Generative AI models improve them is key to grasping their full potential.
Understanding Chatbots
Chatbots are software applications designed to simulate human conversation. They interact with users through text or voice, aiming to provide information, solve problems, or perform tasks.
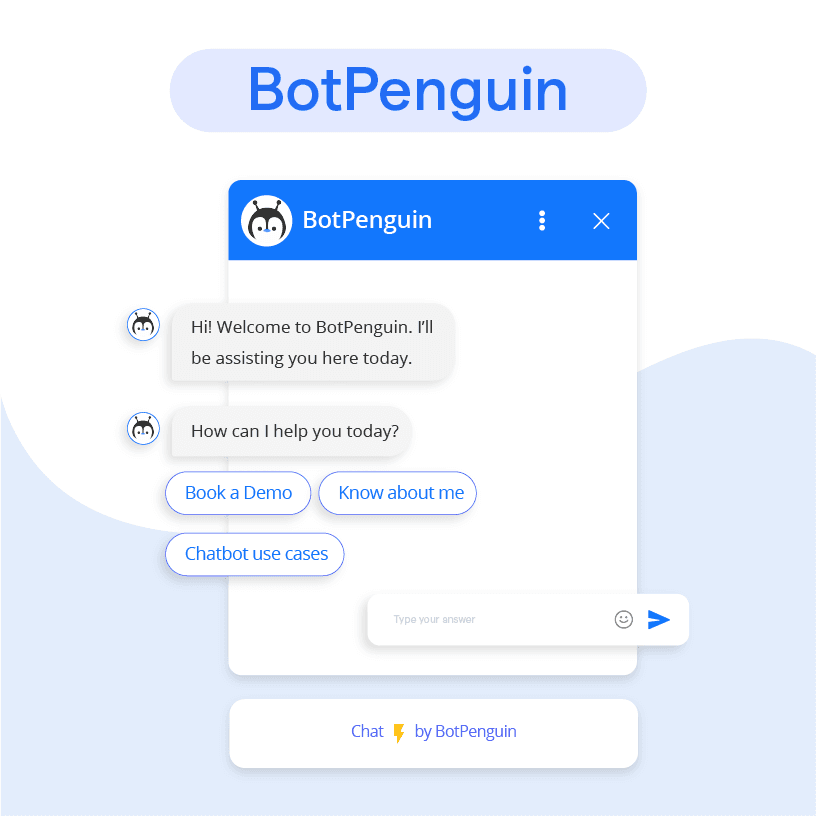
Chatbots can be simple or sophisticated, depending on how they are programmed.
Types of Chatbots
The different types of chatbots are the following:
- Rule-Based Chatbots: These chatbots follow a set of predefined rules. They respond to specific commands and keywords.
- AI Chatbots: These chatbots use artificial intelligence to understand and respond to more complex queries. They can recognize various ways of asking the same question and learn from interactions to improve over time.
What is Generative AI?
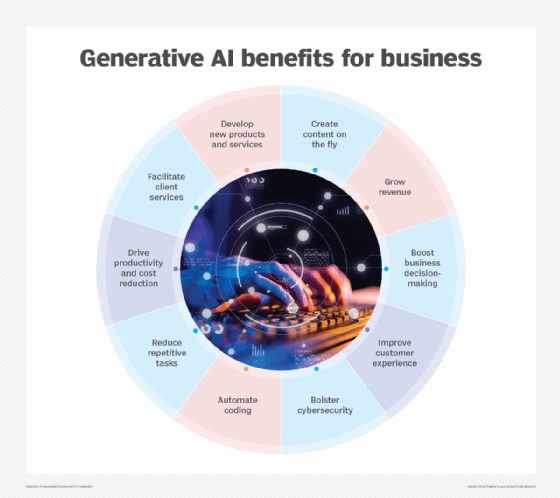
Generative AI is a type of artificial intelligence that can create new content. It learns from large datasets and generates text, images, or other media.
Generative AI models, like GPT-3, analyze patterns in data and produce outputs that are often indistinguishable from human-generated content.
Generative AI models can write articles, compose music, or even create art. In the context of chatbots, it means generating human-like responses that are contextually relevant and engaging.
Benefits of Generative AI in Chatbots
Given below are the benefits of generative AI models in chatbots :
Improved Response Accuracy
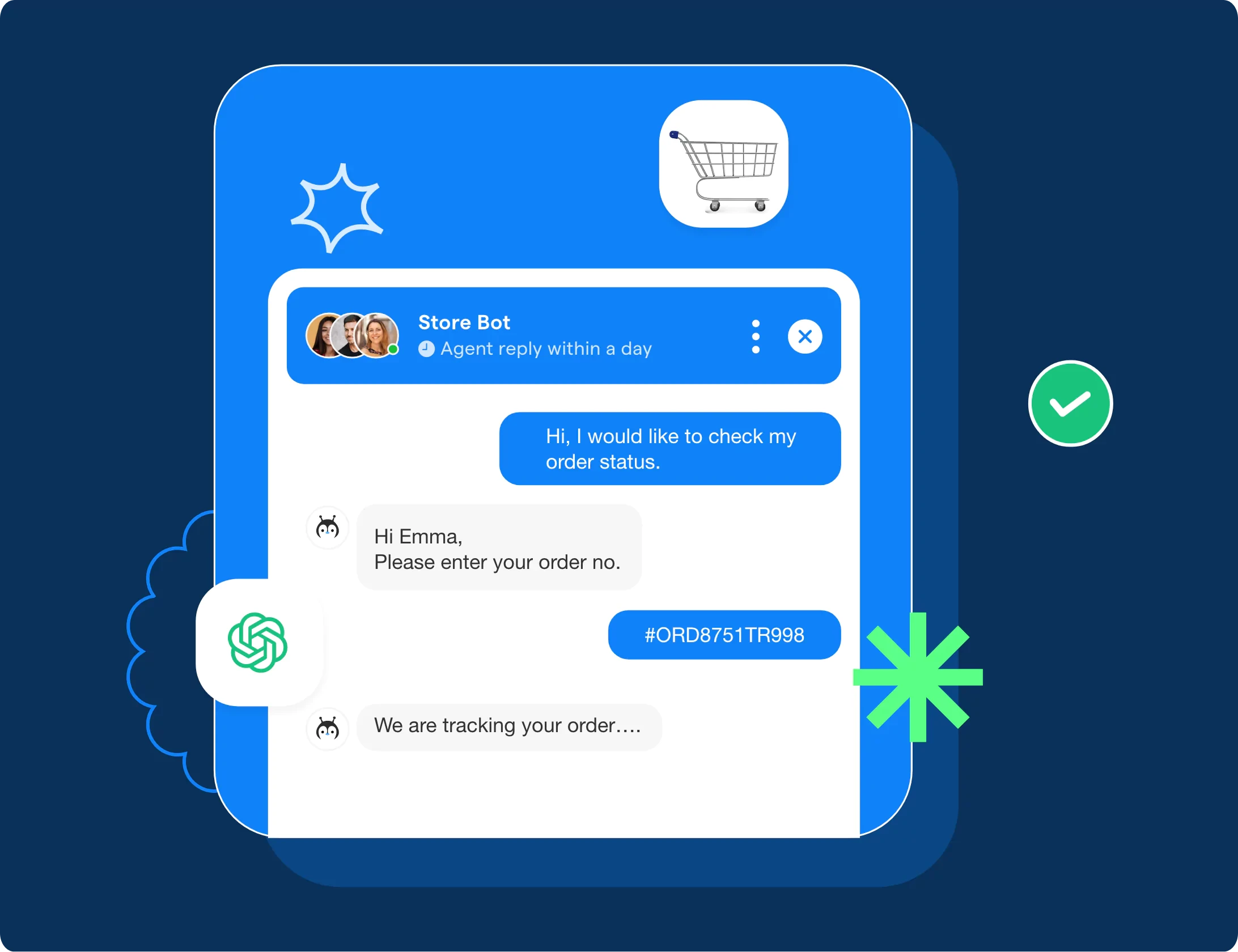
Generative AI models enhance the accuracy of chatbot responses. They understand the context and nuances of user queries better than traditional AI. This leads to more precise answers.
For example, a travel chatbot using Generative AI can understand and answer complex questions about the best time to visit a place, analyzing various factors like weather data and tourist trends to give an accurate response.
Enhanced Engagement
Generative AI creates more engaging interactions. It can produce varied and interesting responses, making the conversation feel natural.
A retail chatbot can not only answer questions about products but also suggest complementary items based on previous conversations. This keeps the interaction dynamic and engaging.
Natural Language Processing Capabilities
Generative AI excels at natural language processing (NLP). It understands and generates human language in a way that feels natural.
An educational chatbot, like BotPenguin, can help students with homework, providing clear, step-by-step explanations and adapting its answers to follow-up questions, much like a human tutor.
Generative AI models revolutionize how chatbots interact with users. They make conversations more accurate, engaging, and natural. As these technologies continue to evolve, we can expect even more sophisticated and human-like interactions from chatbots.
With its integration of generative AI, BotPenguin stands out as an exceptional choice for educational institutions looking to enhance student interactions.
Generative AI models are transforming chatbots, making them smarter and more responsive. Understanding how these models work is crucial to appreciate their impact.
We'll use BotPenguin, a popular chatbot, as an example to illustrate this process.
How Generative AI Models Work in Chatbots
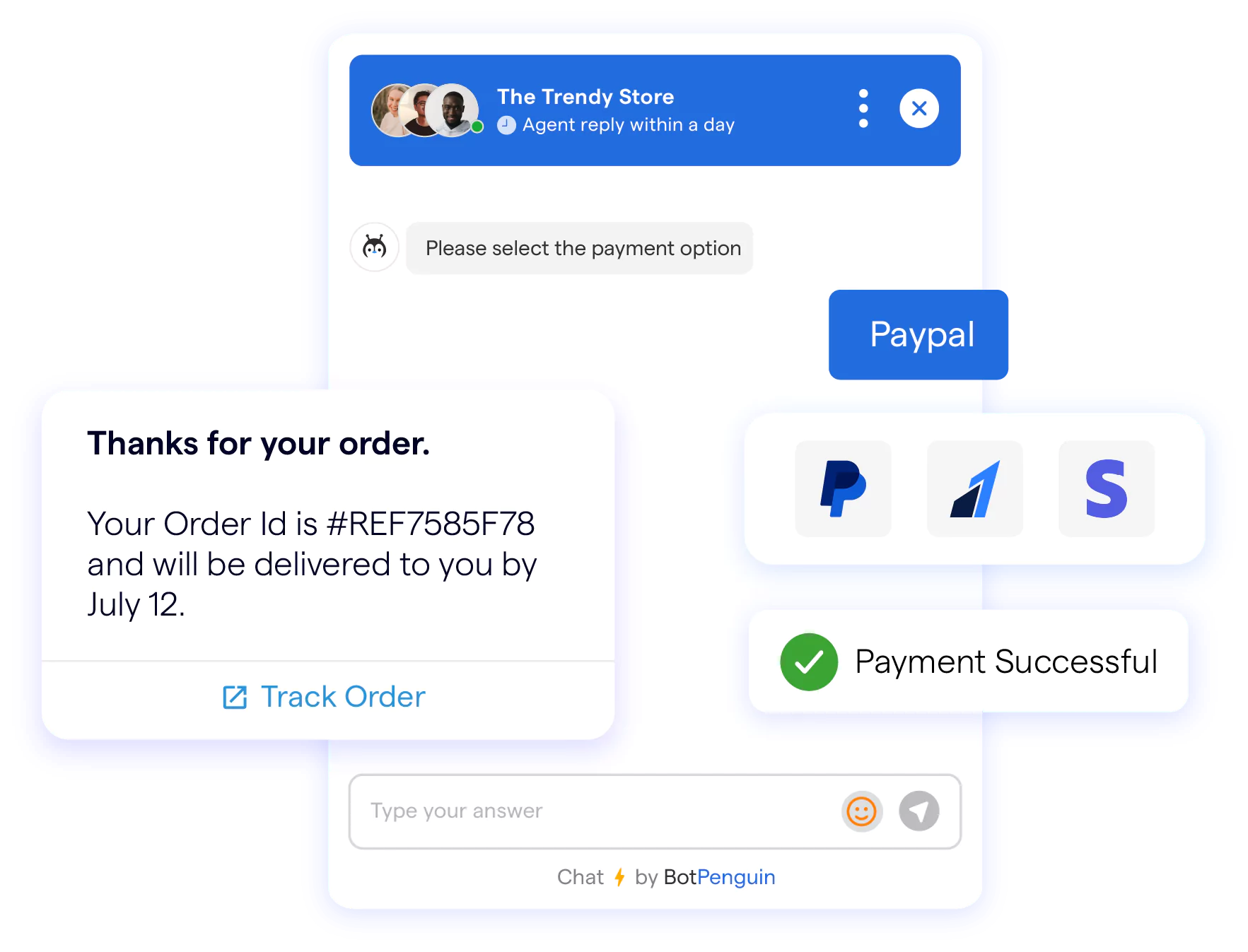
Generative AI has revolutionized how chatbots interact with users. These advanced models make conversations feel more natural and engaging.
By leveraging state-of-the-art language models, BotPenguin enables businesses to deploy AI chatbots that can understand complex queries, generate human-like responses, and adapt to diverse conversational contexts.
Understanding Generative AI Models
Generative AI models, like GPT-4, are designed to create human-like text. They learn patterns in language from vast amounts of data. This allows them to generate responses that are contextually relevant and engaging.
- Data Training: Generative AI models are trained on diverse text datasets from books, websites, and conversations.
For example, GPT-4 might learn how people talk about movies by analyzing thousands of movie reviews.
- Pattern Recognition: Generative AI models recognize patterns and relationships in the data. If someone asks about a good movie to watch, the model can suggest a popular film based on its understanding of what users usually like.
- Contextual Understanding: Generative AI models can understand context. If a user asks, "What's the weather like?" after saying, "I'm planning a picnic," the model knows to give a weather report.
Enhancing Conversations with Generative AI
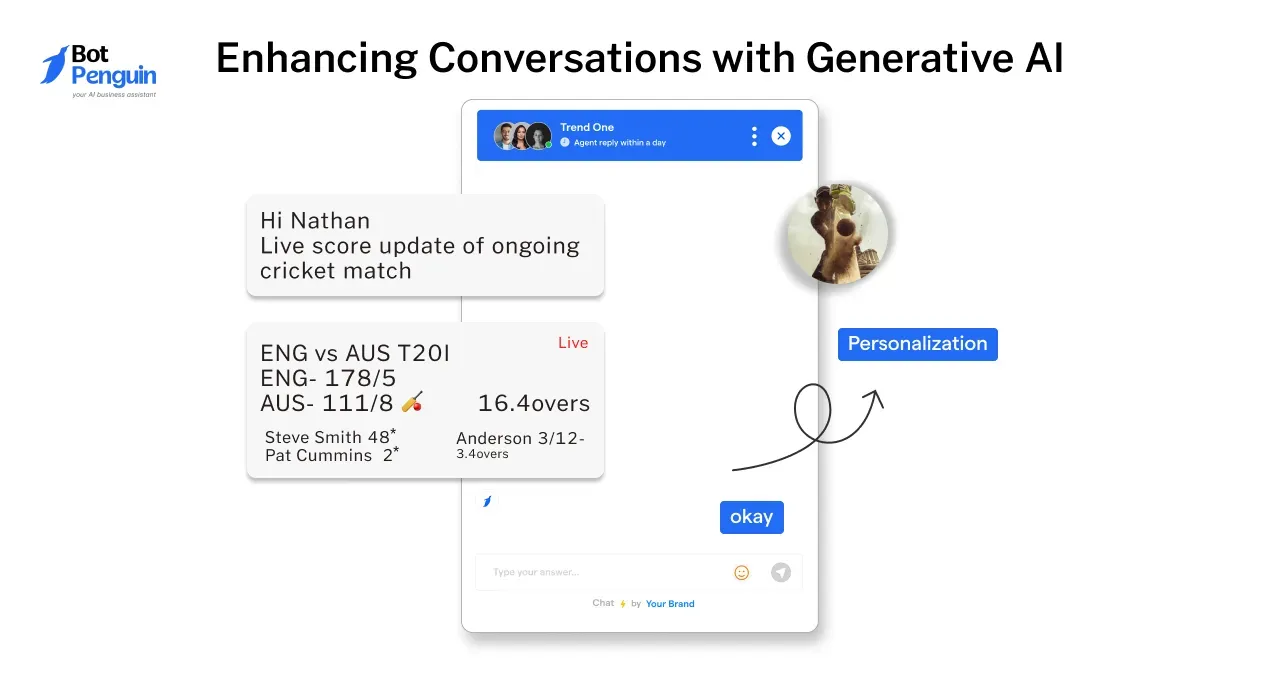
Generative AI models make chatbot conversations more dynamic and human-like.
- Natural Responses: Unlike traditional chatbots, generative models create responses on the fly. Imagine asking a chatbot for a joke.
Instead of giving a pre-written joke, it can come up with a new, funny response each time.
- Personalization: These models can remember previous interactions. If a user frequently asks about sports scores, the chatbot can tailor its responses to include the latest updates in their favorite sports.
- Handling Ambiguity: Generative AI can handle vague questions better. If a user says, "I'm feeling down," the chatbot can respond empathetically and ask if the user wants to talk about it or suggest something uplifting.
Suggested Reading:
How Generative AI models are used in building AI Chatbots
Practical Applications in Chatbots
Generative AI models have various real-world applications in chatbots, making them smarter and more versatile.
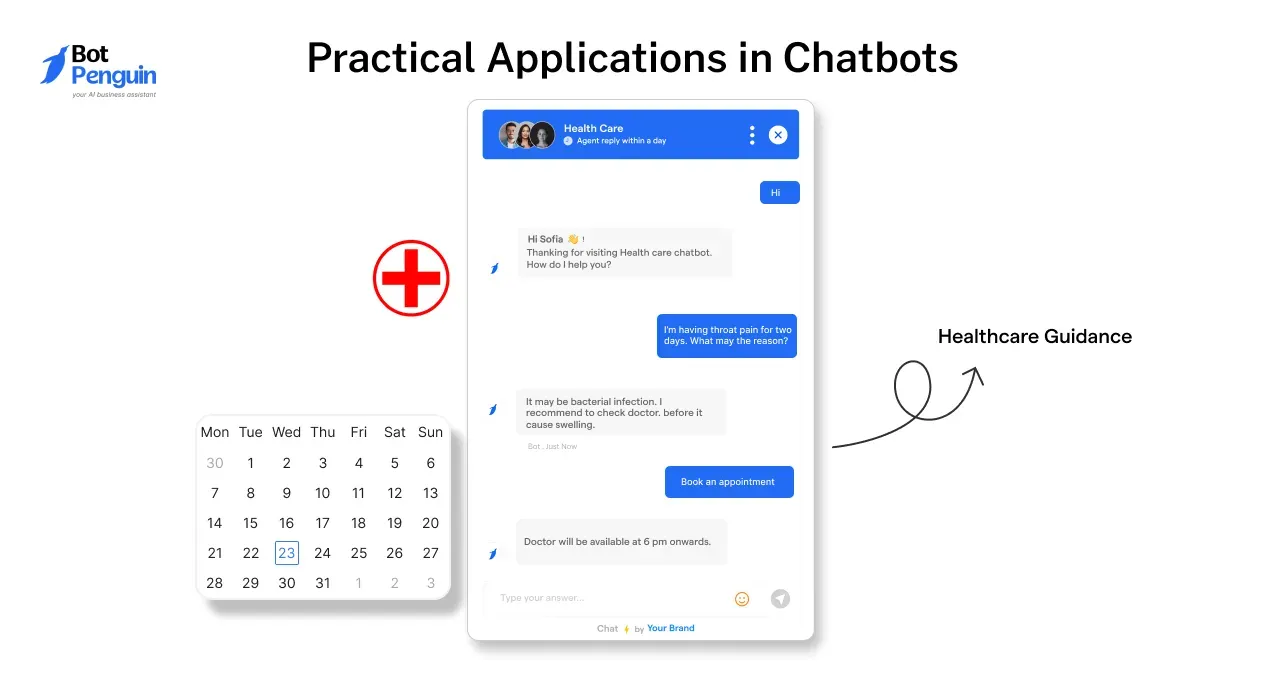
- Customer Support: They provide quick and accurate answers. For instance, if a customer asks about the return policy, the chatbot can give a detailed explanation or guide them through the process step-by-step.
- E-commerce Assistance: They help users find products. If someone is looking for a birthday gift, the chatbot can ask about the recipient's interests and suggest personalized options.
- Healthcare Guidance: In health apps, generative AI chatbots can provide information about symptoms. If a user mentions a sore throat and fever, the chatbot can suggest possible causes and recommend seeing a doctor.
Challenges and Limitations of Generative AI Models
While Generative AI models offer significant benefits, they also face several challenges that need to be addressed for optimal performance.
Data Privacy Concerns
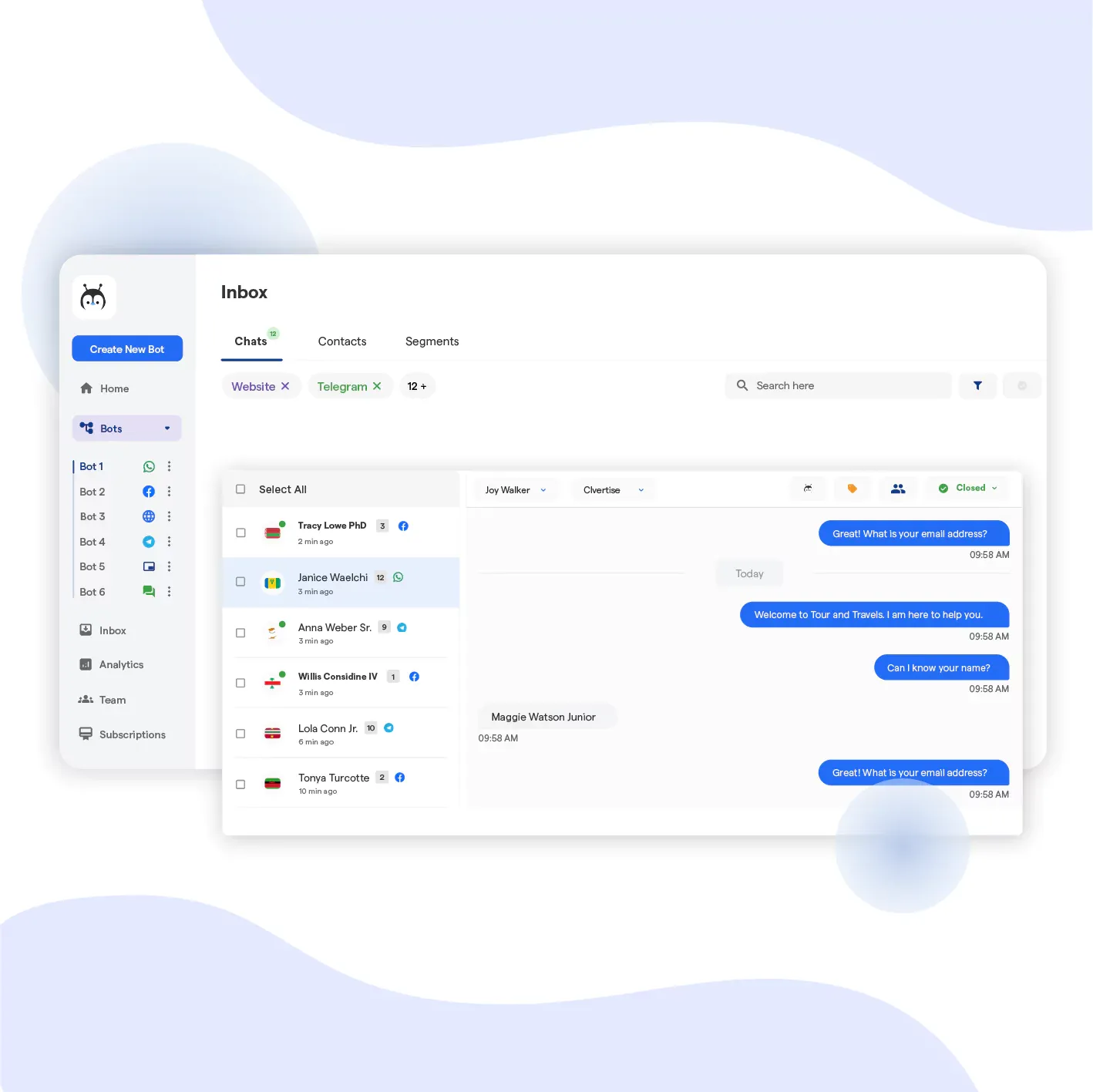
Generative AI models require large amounts of data for training and operation. This data often includes personal and sensitive information.
- Privacy Issues: Handling vast amounts of personal data raises privacy concerns. Ensuring that user data is collected, stored, and processed securely is critical.
Breaches or misuse of data can lead to serious privacy violations and loss of user trust.
- Regulations: Compliance with data protection regulations, such as GDPR or CCPA, is essential. These regulations impose strict guidelines on data usage and protection, making it challenging to balance data needs and privacy.
High Computational Resources
Running Generative AI models, especially large ones, requires substantial computational power.
- Resource Intensive: Training and deploying these models demand high-performance hardware, such as GPUs or TPUs, which are expensive and consume a lot of energy.
This can be a barrier for small and medium-sized enterprises (SMEs) looking to implement advanced AI chatbots.
- Scalability: Scaling Generative AI applications to handle a large number of users simultaneously requires significant infrastructure.
This increases operational costs and complexity, making it challenging to maintain efficiency and cost-effectiveness.
Potential for Bias
Generative AI models learn from the data they are trained on, which can include biases present in the data.
- Bias Propagation: If the training data contains biases, the model can perpetuate and even amplify these biases in its responses.
This can lead to unfair or inappropriate interactions, affecting user experience and trust.
- Detection and Mitigation: Identifying and mitigating bias in AI models is a complex task. It requires continuous monitoring and refinement of the training data and algorithms to ensure fairness and neutrality in the chatbot’s interactions.
Suggested Reading:
Use Generative AI Models to Improve Customer Service in Business
Maintaining Up-to-Date Information
Generative AI models need to stay current with new information and trends to remain relevant and accurate.
- Dynamic Updates: The information and knowledge base used by the chatbot must be regularly updated to reflect the latest data, trends, and user expectations.
This ensures that the chatbot provides accurate and timely responses.
- Training Frequency: Regularly retraining the AI models with new data is essential but resource-intensive. This requires ongoing access to fresh data and computational resources, adding to the operational burden.
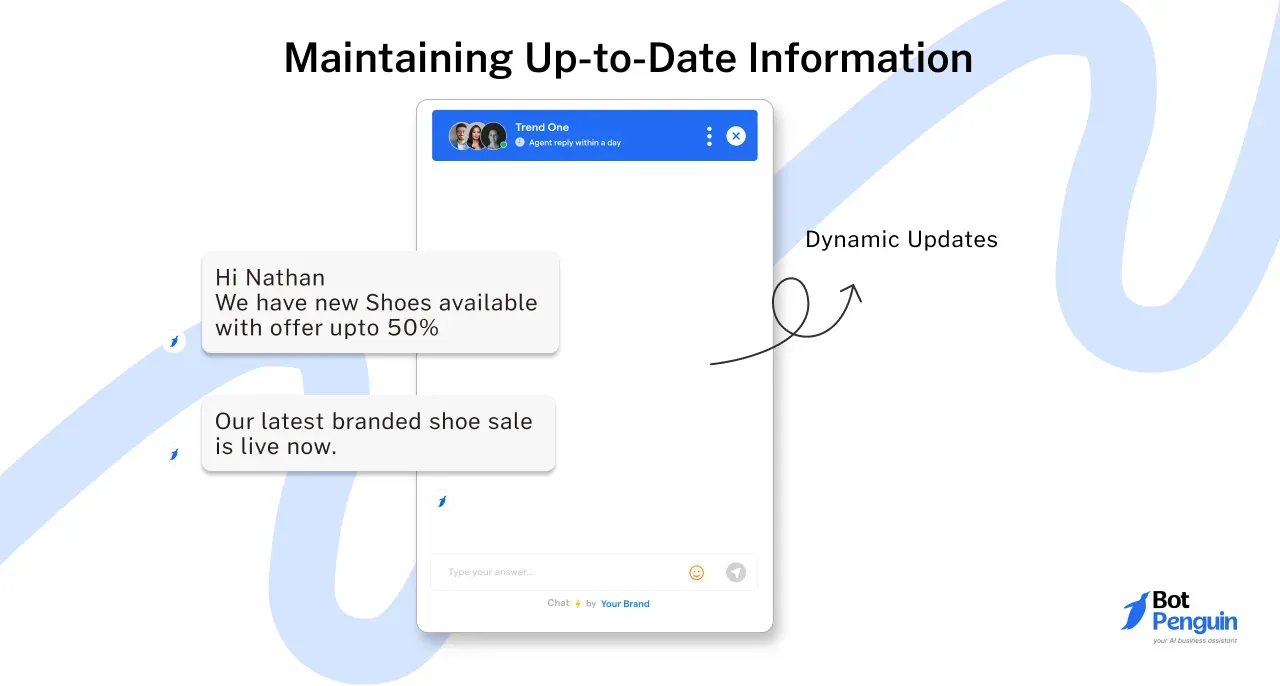
Despite these challenges, the potential of Generative AI models in enhancing AI chatbots is undeniable.
By addressing data privacy concerns, managing computational resources, mitigating bias, and maintaining up-to-date information, we can harness the full power of Generative AI.
This will lead to more effective, trustworthy, and dynamic conversational AI applications.
Generative AI models are revolutionizing the way chatbots interact with users, offering improved accuracy, engagement, and natural language capabilities.
While these advancements come with challenges such as data privacy, high computational resources, potential biases, and the need for constant updates, the benefits far outweigh the hurdles.
BotPenguin and Generative AI Chatbots
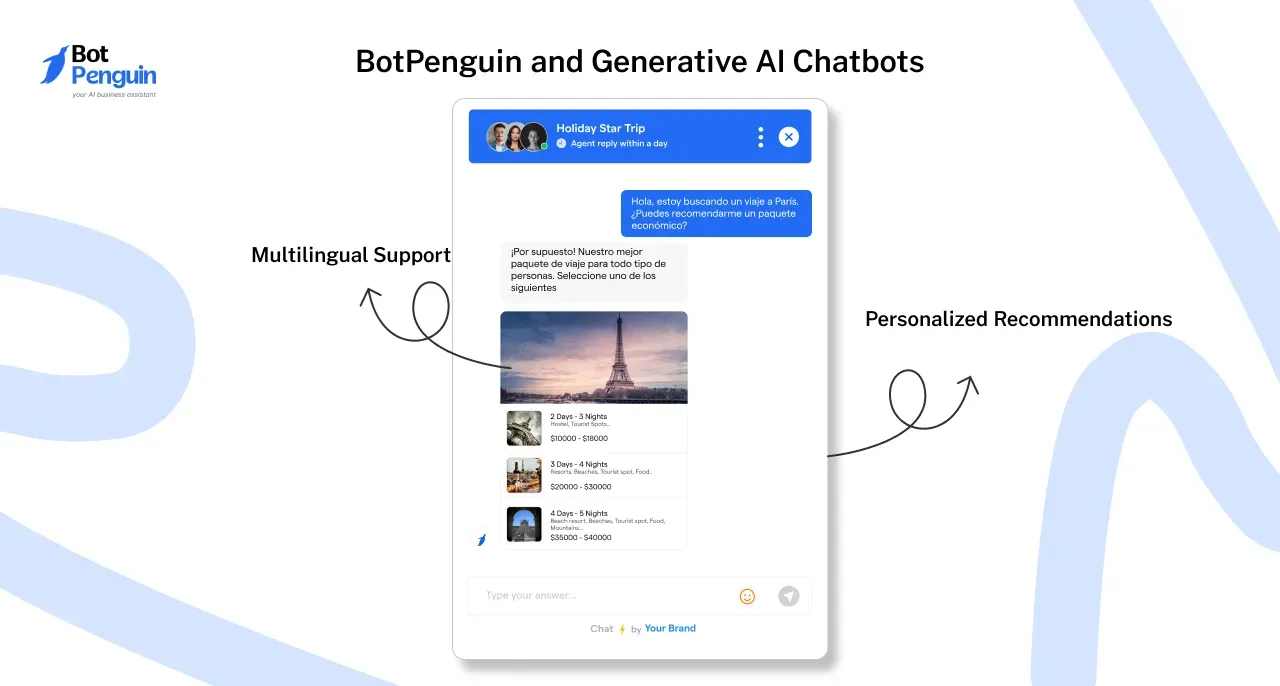
BotPenguin, as a chatbot platform, can significantly benefit from integrating generative AI models to enhance its conversational capabilities.
By leveraging these advanced models, BotPenguin can offer businesses more sophisticated chatbot solutions that provide improved user experiences.
Generative AI can help BotPenguin's chatbots understand complex user intents, generate more natural and contextually appropriate responses, and handle a broader range of queries without human intervention.
This integration can lead to higher customer satisfaction, increased engagement, and more efficient customer service for businesses using BotPenguin's platform.
Additionally, generative AI models can enable BotPenguin to offer advanced features such as multilingual support, sentiment analysis, and personalized recommendations, further distinguishing its offering in the competitive chatbot market.
Conclusion
In conclusion, the integration of generative AI models into AI chatbots has dramatically transformed the landscape of conversational AI.
These advanced language models have elevated chatbot interactions from simple, rule-based exchanges to nuanced, context-aware conversations that closely mimic human communication. The wide array of generative AI applications in this field continues to expand, offering innovative solutions across various industries.
BotPenguin, a leading platform in this space, exemplifies how generative AI can be harnessed to create more intelligent and responsive chatbots.
The future of conversational AI looks promising, with generative AI examples showcasing capabilities that were once thought impossible. From customer service to mental health support, these enhanced chatbots are redefining user experiences and operational efficiencies.
As language models continue to evolve, we can expect even more sophisticated generative AI applications in the chatbot domain.
Ultimately, the success of AI chatbots powered by generative AI hinges on their ability to provide value while maintaining ethical standards. Platforms like BotPenguin are at the forefront of this challenge, balancing innovation with responsibility.
As we move forward, the synergy between conversational AI and generative models will undoubtedly continue to push the boundaries of what's possible in human-machine interaction, opening up new horizons for businesses and users alike.
Frequently Asked Questions (FAQs)
How do Generative AI models improve chatbot accuracy?
Generative AI models enhance chatbot accuracy by understanding context and nuances, providing more precise and relevant responses based on learned data patterns.
What makes Generative AI chatbots more engaging?
Generative AI chatbots generate varied and interesting responses, making conversations feel natural and dynamic, which enhances user engagement.
How does Generative AI handle complex queries?
Generative AI models can analyze and understand complex queries, generating appropriate responses by leveraging vast amounts of training data and contextual understanding.
What are the privacy concerns with Generative AI chatbots?
Privacy concerns arise from the need to handle large amounts of personal data, requiring robust security measures and compliance with data protection regulations.
Can Generative AI chatbots reduce operational costs?
Yes, by automating customer interactions and providing efficient support, Generative AI chatbots can reduce the need for human intervention, lowering operational costs.
How do Generative AI models keep chatbot information up-to-date?
Generative AI models require regular updates and retraining with fresh data to maintain accurate and current information in chatbot interactions.