The advent of Artificial Intelligence (AI) has dramatically impacted how businesses operate and manage daily activities.
The development of various AI apps and technologies has helped organizations to make better-informed decisions and automate repetitive work, resulting in more efficient and productive operations.
With the popularity of well-known generative AI technologies like Midjourney and ChatGPT, businesses can produce new concepts, content, and solutions more quickly. This enhances decision-making, streamlines operations, and enables organizations to compete in an ever-changing market by developing new products and services.
According to a McKinsey analysis, the global generative AI market is estimated to reach $100 billion by 2025.
Generative AI comprises a variety of models and strategies for creating fresh data or content that resembles human data. There are various generative AI models, each with its content generation method.
This article covers the most prevalent types of generative AI and its applications in various industry fields.
Generative AI Models
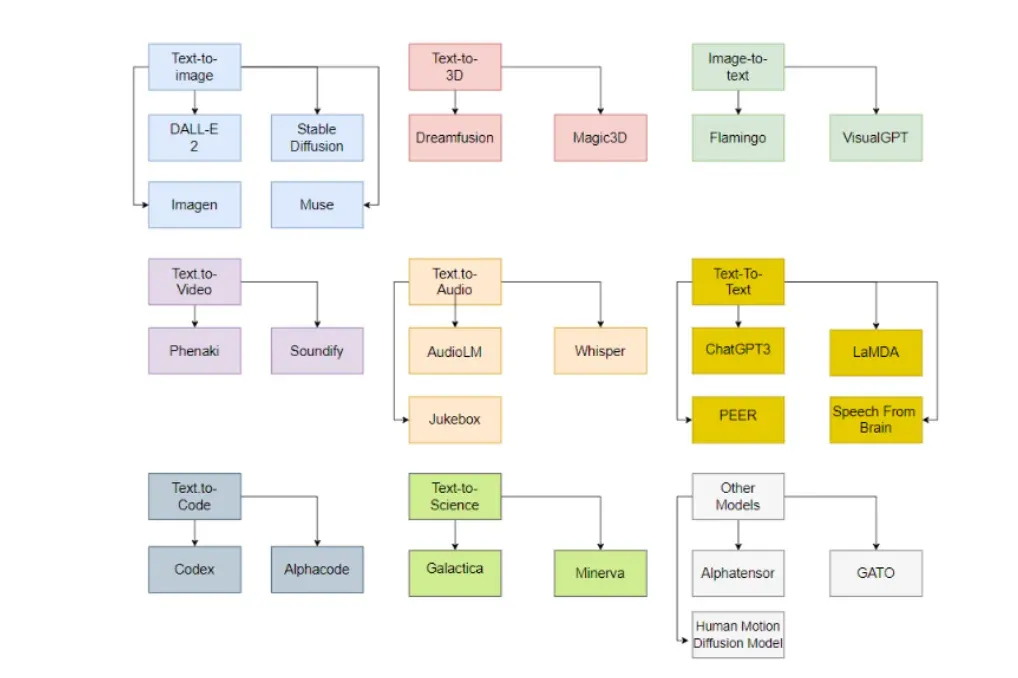
Generative AI models are algorithms that generate new content, such as images, videos, music, or text, similar to the training data they were fed.
These models use complex mathematical techniques to understand patterns and create new content replicating the input data's characteristics.
From art and design to healthcare and entertainment, generative AI models can create new content, generate realistic images, enhance customer experiences, and optimize various processes.
Types of Generative AI Models
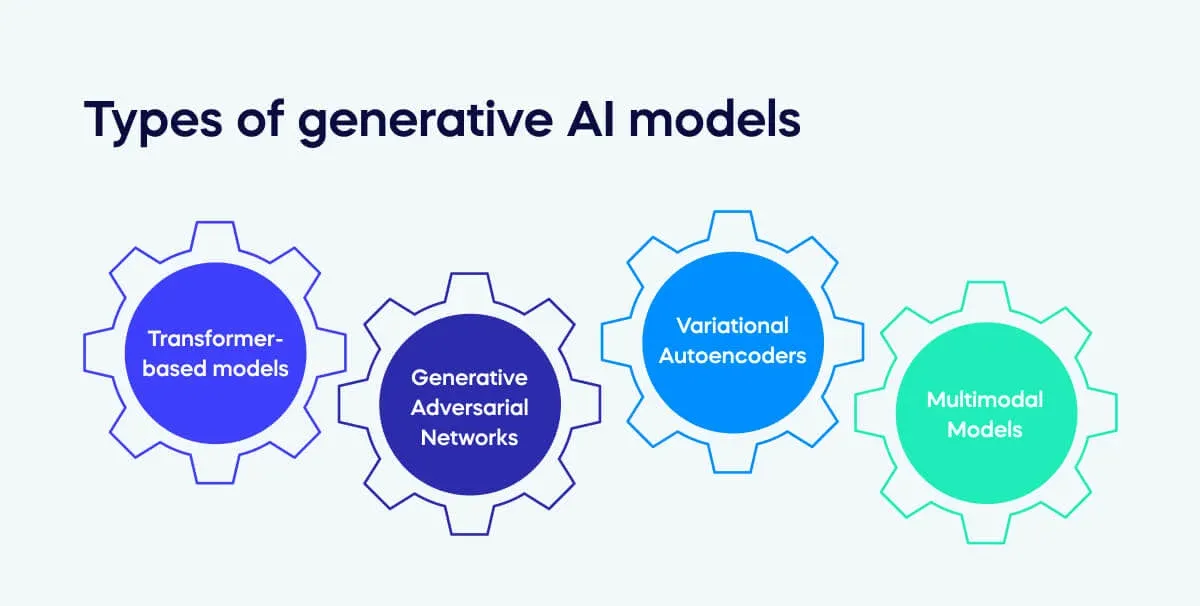
Now, let's dive into the three main types of generative AI models: Variational Autoencoders (VAEs), Generative Adversarial Networks (GANs), and Recurrent Neural Networks (RNNs).
Variational Autoencoders (VAEs)
VAEs are probabilistic models that learn the underlying structure of the input data and then generate new samples based on that structure.
They consist of an encoder network that compresses the input into a lower-dimensional representation and a decoder network that reconstructs the original input from the compressed representation.
By learning this compressed representation, VAEs can generate new data similar to the training data but differ subtly.
Applications of VAEs in Image Generation and Data Compression
VAEs have diverse applications, particularly in image generation and data compression. They learn dataset distribution to generate new images resembling training samples.
In data compression, VAEs encode data efficiently using a compressed representation, enabling compact input while maintaining meaningful reconstruction possibilities.
Generative Adversarial Networks (GANs)
GANs comprise a generator and a discriminator that compete in an adversarial setting. The generator creates new samples, and the discriminator distinguishes real from generated ones.
Both networks enhance their performance through this process, with the generator aiming to deceive the discriminator and the discriminator improving its ability to differentiate between real and generated samples.
Real-World Applications of GANs in Image and Video Synthesis
GANs have gained significant attention in computer vision, particularly image and video synthesis.
They can be used to create realistic images from text descriptions, generate artistic or fictional images, and even fill in missing parts of images.
GANs have also been utilized in video synthesis, enabling the generation of realistic videos given certain constraints or input.
Recurrent Neural Networks (RNNs)
RNNs are a class of neural networks specifically designed to handle sequential data, where the output depends not only on the input but also on the previous states of the model.
RNNs are well-suited for generative modeling tasks, which involve creating data sequences. They excel at capturing sequential dependencies, making them ideal for tasks like natural language generation and music composition.
Utilization of RNNs in Natural Language Generation and Music Composition
RNNs are used in natural language processing for coherent text generation. Applications include language translation, chatbots, and music composition for generating melodies, lyrics, and compositions.
Suggested Reading:
Applications of Generative AI Models
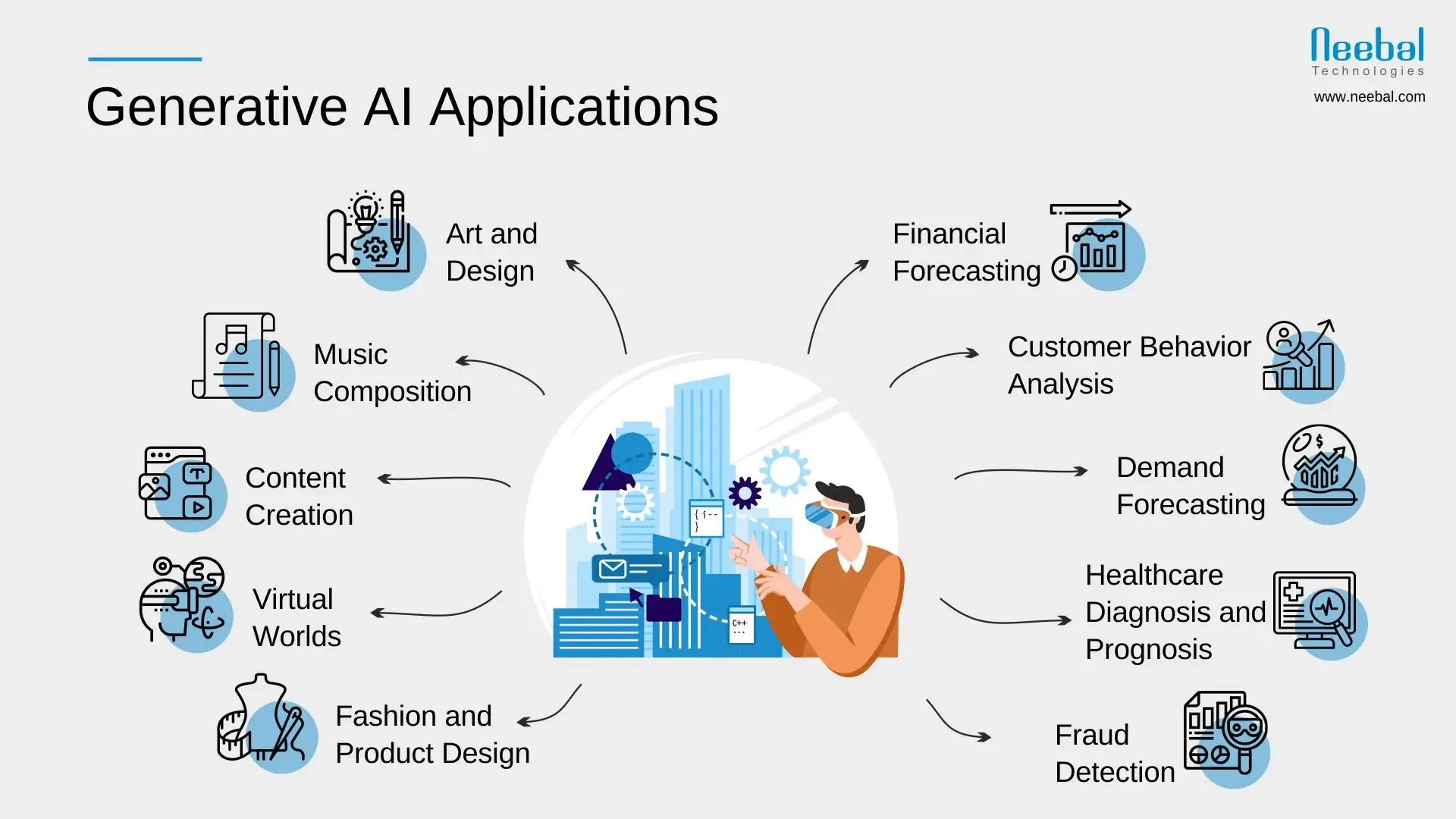
Generative AI models play a pivotal role in crafting immersive and interactive experiences, transforming various industries:
Art and Design
Generative AI fuels artistic creativity, generating unique artwork and compositions using techniques like Variational Autoencoders (VAEs). Notable applications include creating digital paintings, and blending human intuition with AI's creativity in galleries.
Midjourney, Canva AI, DALL-E 2, and Jasper Art are popular examples of AI art generators.
While the results rendered by these tools aren't perfect, they can easily rival human creativity or surpass it.
Music Composition
In music, Recurrent Neural Networks (RNNs) analyze musical patterns to compose original melodies and harmonies and even fit specific composer styles.
Musicians collaborate with AI-generated pieces, exploring new musical landscapes while incorporating their own unique artistic expressions and interpretations.
Content Generation
These models extend beyond visuals and sounds, generating written content that resembles human-written text. AI-written news stories streamline journalism, while AI-crafted fiction and video game dialogues enhance immersion.
Furthermore, generative AI is used in marketing and advertising to create personalized content, product descriptions, and social media posts that resonate with target audiences, improving engagement and conversion rates.
Virtual Reality (VR) and Gaming
Generative AI shapes VR and gaming with realistic environments that adapt to user actions. It creates dynamic, lifelike landscapes for immersive gaming experiences using real-world data like satellite imagery.
It also enhances character development and behavior generation, generating realistic virtual beings with dynamic personalities and responding to user interactions in real-time.
This technology opens up endless possibilities for interactive and immersive storytelling in virtual worlds.
Suggested Reading:
Healthcare and Drug Discovery
In healthcare, AI analyzes medical data for drug discovery and medical image synthesis. It accelerates research, aiding in accurate diagnosis by generating high-resolution medical images and evaluating drug molecules.
Generative AI plays a crucial role in healthcare and drug discovery by analyzing vast amounts of medical data to identify patterns, predict treatment outcomes, and discover new potential drugs.
It aids in accurate diagnosis by generating high-resolution medical images, enabling doctors to detect abnormalities with enhanced precision.
Fashion and Design
Fashion sees innovative designs and trend forecasts with generative AI. It influences garment design, explores fabric combinations, and predicts trends by analyzing vast data and steering targeted design decisions.
Lately, generative AI models are increasingly being employed for personalized and sustainable fashion.
Generative AI Chatbots
Chatbots are becoming more popular among businesses for providing customer support and automating customer service processes. With the help of generative AI models, chatbots can understand natural language and generate human-like responses, making conversations more engaging and personal for customers.
Integrating generative AI in chatbots enables them to offer personalized recommendations, analyze customer feedback, and provide more efficient solutions in various industries, such as e-commerce, finance, and healthcare.
Businesses can leverage chatbots to improve customer experience, reduce response time, and increase customer satisfaction.
And, taking your first step toward Generative AI chatbots isn't that tough. Meet BotPenguin- the home of chatbot solutions. With all the heavy work of chatbot development already done for you, deploy chatbots for multiple platforms and make multi-channel support look easy for business:
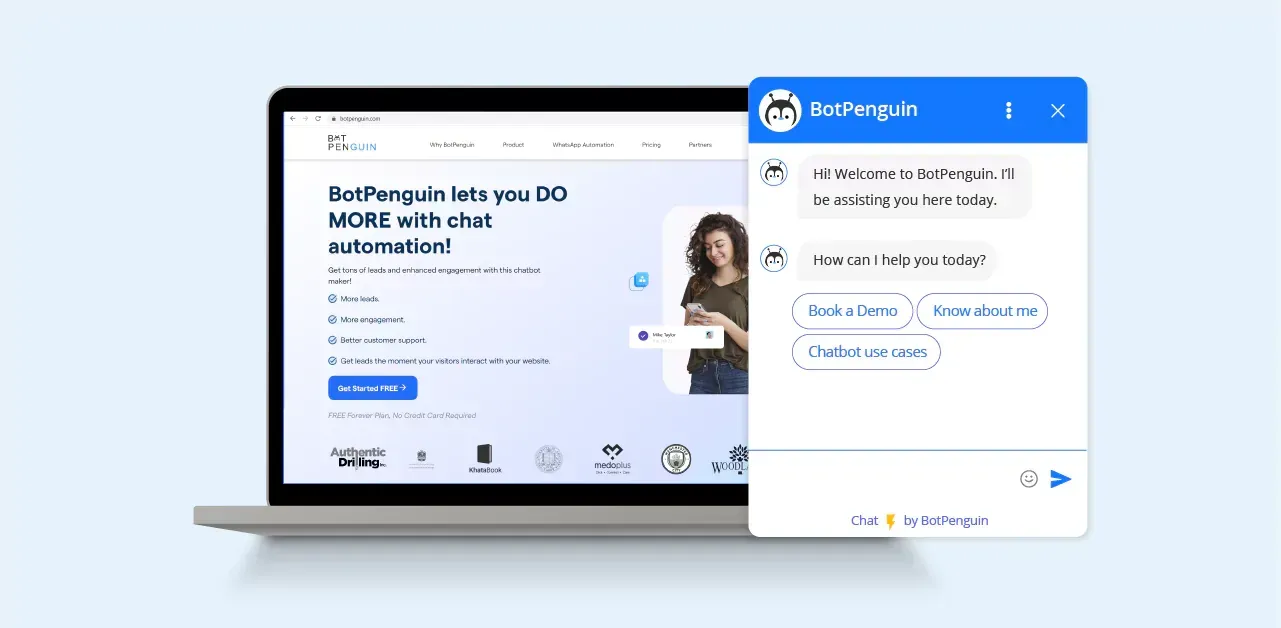
Benefits and Limitations of Generative AI Models
Generative AI models bring many benefits that revolutionize various domains. However, being aware of their limitations and challenges is also essential.
Benefits of Generative AI Models
- Boost creativity and innovation in various fields by generating unique content.
- Increase efficiency by quickly producing diverse and innovative ideas for artists, designers, and developers.
Limitations and Challenges
- Address ethical concerns and biases in generated content by ensuring diverse and unbiased training data.
- Ensure reliability and quality of output by rigorous testing, focusing on coherence, consistency, and adherence to standards. Continuous monitoring and improvement are essential for user satisfaction.
Conclusion
Across all industries, generative AI is critical for automating repetitive processes, increasing productivity, and improving decision-making.
Generative AI use cases range from healthcare and manufacturing to real estate, finance, and entertainment.
By automating creative processes like content generation and addressing other critical goals like predictive analysis, this AI technology can successfully generate distinctive and engaging user experiences.
Companies that effectively use generative AI have the potential to transform a variety of industries, and those that do so will be well-positioned to grow revenue, cut expenses, and enhance efficiency.
Excited about the potential of AI-based chatbots? Don't wait—try BotPenguin now!
Exploring game-changing apps is crucial as technology evolves. These tools amp up productivity, spark creativity, and provide crucial support across diverse fields.
Unlock the potential of generative AI! It's not just about today; it's about unleashing new possibilities in your work and projects.
Embrace this transformative technology to revolutionize how you engage, create, and accomplish tasks. Start your AI journey and witness the innovation firsthand!
Suggested Reading:
Frequently Asked Questions (FAQs)
What are the different types of generative AI models?
Generative AI models include Variational Autoencoders (VAEs), Generative Adversarial Networks (GANs), and Transformers. Each type has its unique approach to generating content, such as images, text, or music.
How are generative AI models used in the gaming industry?
Generative AI models enhance gaming experiences by creating dynamic and interactive environments. These models can generate realistic landscapes, diverse characters, and compelling narratives, immersing players in virtual worlds.
What applications do generative AI models have in healthcare?
Generative AI models are utilized in healthcare for medical image synthesis, drug molecule design, and predicting patient outcomes. They assist in accurate diagnosis, virtual screening of potential drugs, and accelerating research and development.
In which industries are generative AI models applied in fashion?
Generative AI models are transforming the fashion industry by aiding in fashion design, trend forecasting, and personalized shopping experiences. They can generate unique clothing designs, analyze fashion trends, and optimize inventory management.
How can generative AI models be used for content creation?
Generative AI models can automate content generation by assisting in writing articles, creating music, or designing artwork. They provide endless variations and ideas, helping content creators in various fields.
What are the limitations of generative AI models?
Generative AI models face challenges related to bias in data, ensuring output quality, and the ethical implications of their generated content. Monitoring for biases and quality control are essential to maintain fairness and reliability.