What is Generative AI?
Generative AI is a type of artificial intelligence that uses algorithms to generate new and original content, such as images, video, text, or audio. It is based on deep learning models that can learn from large datasets and generate novel outputs with little or no human input.
Generative AI has applications in various fields, including art, music, gaming, and content creation. It is a rapidly evolving technology that has the potential to revolutionize many industries in the coming years.
Key Components of Generative AI
- Algorithms: These are the mathematical engines that drive the creative process, helping AI systems learn patterns and generate new content.
- Training Data: Like any good student, AI needs a solid foundation to learn from. This is where training data comes in, providing real-world examples that the AI can use to hone its skills.
- Evaluation Metrics: To ensure that our AI is generating high-quality content, we need a way to measure how well it's doing. Evaluation metrics help us assess the AI's performance and make improvements as needed.
Real-World Applications
Generative AI has a wide range of practical applications. Here are a few examples:
- Art and Design: By using Generative AI, artists and designers can create stunning visuals and graphics, pushing the boundaries of their creativity.
- Text Generation: From writing emails to crafting news articles, Generative AI can help automate the creation of written content, saving time and resources.
- Music Composition: Who says AI can't have an ear for music? Generative AI can generate new tunes and melodies, offering a fresh perspective on musical composition.
Generative AI Terminology
1. Essential Vocabulary and Concepts
A. Latent Space
Latent space is an essential concept in generative AI, referring to a lower-dimensional representation of data. By mapping input data to this space, generative models can learn the underlying relationships and patterns, enabling them to generate new samples with similar properties.
B. Conditioning
Conditioning in generative AI involves providing additional information to an AI model to guide its output. For example, when generating images of clothing, conditioning the model with a specific color or style will lead to the creation of images that match the given criteria.
C. Fine-Tuning
Fine-tuning is the process of adjusting a pre-trained AI model to improve its performance on a specific task or dataset. By building on existing knowledge, fine-tuning allows for faster training and better results than training a model from scratch.
2. Key Metrics in Generative AI
A. Inception Score
The Inception Score is a metric used to evaluate the quality of AI-generated images. It measures how diverse and realistic the generated images are by comparing them to a pre-trained image classifier. A higher Inception Score indicates better image quality and diversity.
B. Frechet Inception Distance
The Frechet Inception Distance (FID) is another metric used to assess the quality of AI-generated images. It compares the distribution of generated images to that of real images, with a lower FID indicating a closer match and better image quality.
Why is Generative AI Important?
1. Advantages of Generative AI
Generative AI offers numerous benefits, including:
- Efficiency: With AI handling the heavy lifting of content creation, humans can focus on other tasks, leading to increased productivity.
- Innovation: Generative AI can come up with ideas and designs that might never have occurred to a human, leading to new breakthroughs and discoveries.
- Personalization: AI can tailor content to individual users, creating unique experiences that cater to specific preferences and needs.
2. Impact on Industries
Generative AI is already making waves across various industries:
- Marketing and Advertising: AI-generated content can help businesses create targeted ads and promotional materials, boosting engagement and conversions.
- Healthcare: By generating new drug compounds or analyzing medical images, Generative AI can help expedite research and improve patient outcomes.
- Entertainment: AI-generated music, movies, and video games can offer unique experiences, pushing the boundaries of what's possible in entertainment.
3. Ethical Considerations
As with any powerful technology, there are ethical considerations to keep in mind:
- Misinformation: There's potential for AI-generated content to be used maliciously, spreading false information and deepfakes.
- Job Displacement: While Generative AI can increase efficiency, it might also lead to job losses in certain sectors.
- Bias: AI systems are only as objective as the data they're trained on. If the training data contains bias, the AI-generated content may also be biased.
Who Uses Generative AI?
1. Research Institutions and Organizations
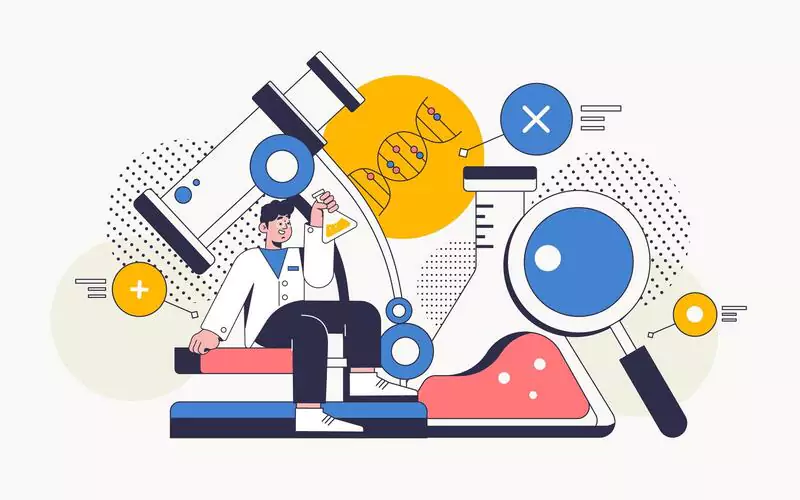
Generative AI has captured the attention of researchers in academia and industry alike. Universities, tech giants, and research labs are all exploring the potential of this cutting-edge technology. From AI-driven art installations to groundbreaking scientific discoveries, these institutions are pushing the boundaries of what's possible with Generative AI.
2. Businesses and Industries
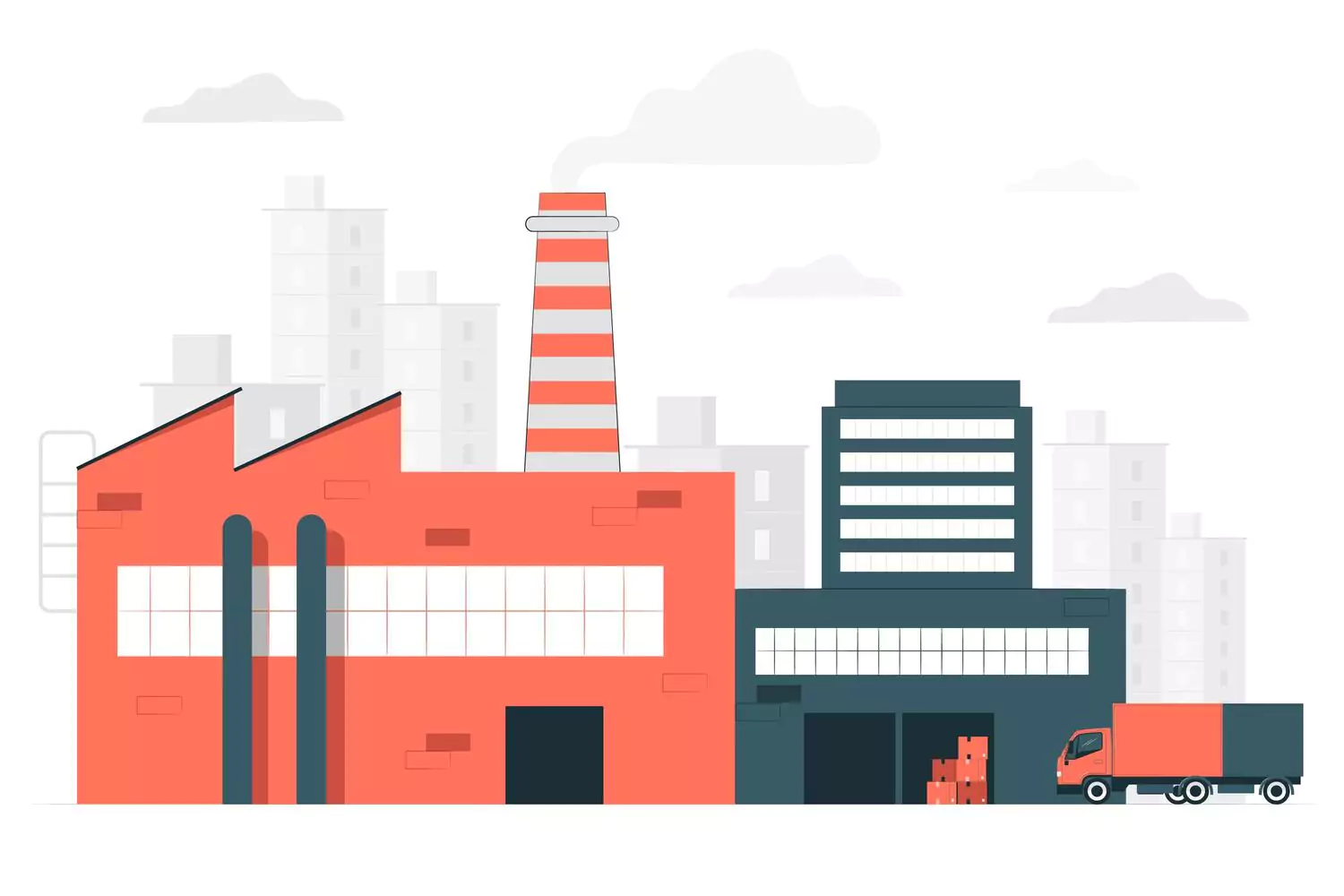
Companies across various sectors are starting to harness the power of Generative AI. Marketing firms are using AI-generated content for personalized ads, while fashion designers are embracing generative algorithms to create unique patterns and styles. In healthcare, pharmaceutical companies are exploring AI-generated drug compounds to expedite drug discovery. The possibilities are endless!
3. Individual Developers and Enthusiasts
You don't need to be a part of a large organization to play with Generative AI. Thanks to open-source libraries and frameworks, individual developers and enthusiasts can experiment with AI-generated content in their own projects. Whether it's creating a custom AI-generated playlist or designing an eye-catching website, the sky's the limit for these creative minds.
When did Generative AI Emerge?
Historical Evolution of Generative AI
The journey of Generative AI began in the mid-20th century, with the birth of artificial intelligence itself. Early AI systems focused on rule-based approaches, but as technology advanced, researchers began to explore more sophisticated techniques, like neural networks.
In the early 2000s, machine learning, and deep learning started to gain traction, laying the groundwork for Generative AI. These approaches allowed AI systems to learn patterns from data, giving them the ability to generate new content based on that data.
Where is Generative AI Applied?
1. Media and Entertainment
Generative AI is making waves in the media and entertainment industries. From creating mesmerizing visual effects to composing catchy tunes, AI-generated content is transforming the way we experience movies, music, video games, and more.
2. Healthcare and Medicine
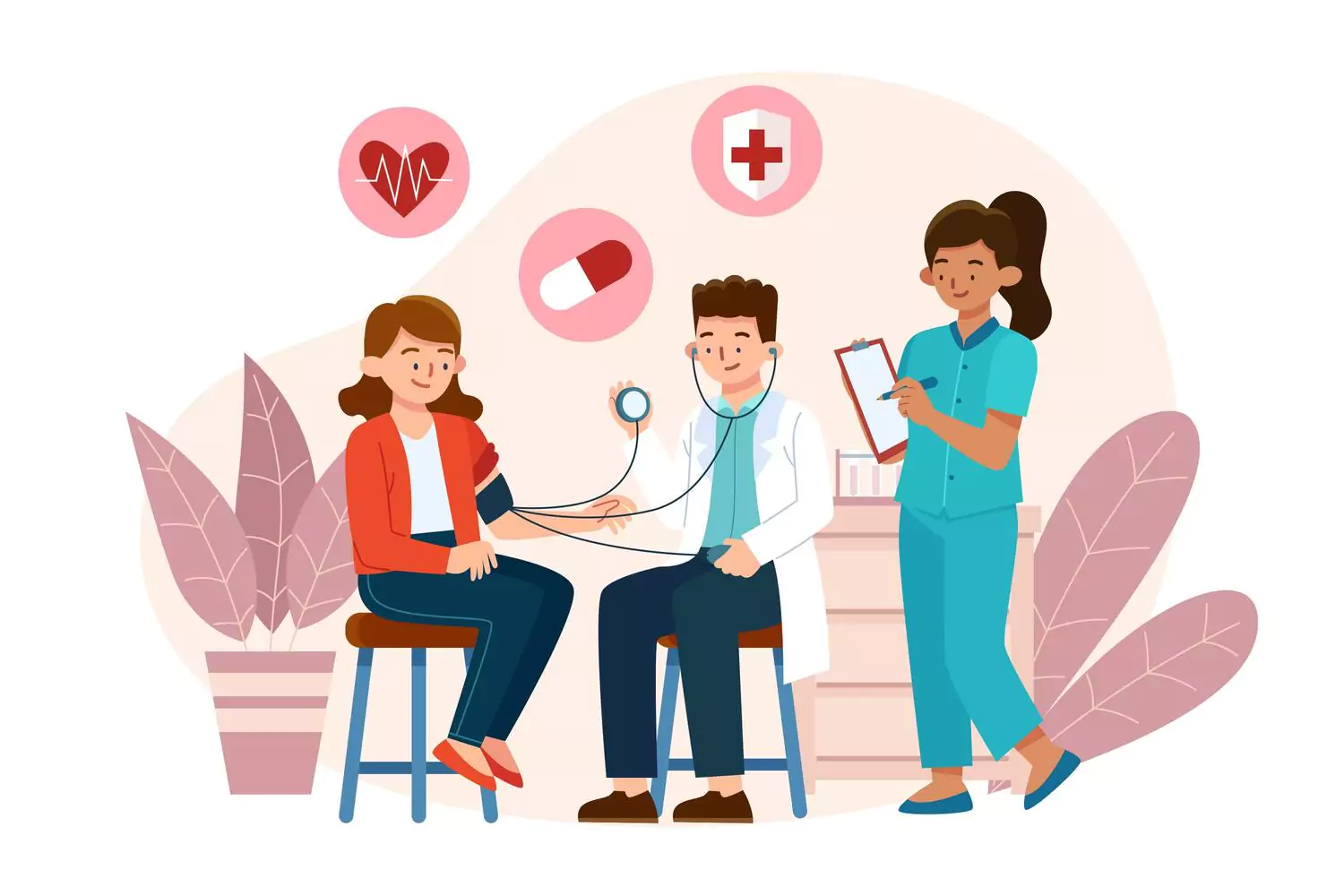
In the world of healthcare and medicine, Generative AI has the potential to revolutionize drug discovery, diagnostics, and personalized treatment plans. AI-generated drug compounds can speed up research, while the analysis of medical images can lead to faster and more accurate diagnoses.
3. Finance and Economics
Generative AI is also finding its footing in finance and economics. From predicting stock market trends to developing tailored investment strategies, AI-generated insights can help investors and financial analysts make more informed decisions.
4. Robotics and Automation
In the realm of robotics and automation, Generative AI can enable robots to learn and adapt to new situations, making them more versatile and efficient. AI-generated control algorithms can lead to more precise movements and improved decision-making.
How Does Generative AI Work?
1. Key Algorithms and Techniques
A. Generative Adversarial Networks (GANs)
GANs are a powerful generative technique that involves two neural networks – a generator and a discriminator – competing against each other. The generator creates fake samples, while the discriminator tries to distinguish between real and fake data. Through this adversarial process, the generator learns to produce more realistic output.
B. Variational Autoencoders (VAEs)
VAEs are another generative approach that uses neural networks to generate new data samples. They work by encoding input data into a lower-dimensional space, then decoding it back to the original space. By controlling the encoded space, VAEs can generate new, unique samples similar to the input data.
C. Transformer Models
Transformer models are a type of neural network architecture specializing in natural language processing. They have been particularly successful in generating human-like text, as seen in language models like OpenAI's GPT-3.
2. Training and Evaluation of Generative AI Models
Training a generative AI model involves feeding it large amounts of data, allowing it to learn underlying patterns and structures. During training, the model adjusts its parameters to minimize the difference between its output and the target data.
Evaluating generative models can be challenging, as there is often no single "correct" output. Instead, researchers use various evaluation metrics to assess the quality, diversity, and realism of the AI-generated content. These metrics help identify areas for improvement and ensure the model is generating high-quality output.
Challenges and Limitations of Generative AI
1. Technical Challenges
Generative AI models can face several technical challenges, such as:
- Training complexity: Training generative models can be computationally intensive and time-consuming, requiring powerful hardware and efficient algorithms.
- Mode collapse: A common issue in generative models, mode collapse occurs when the model generates only a limited variety of outputs, failing to capture the full diversity of the input data.
- Evaluation: Assessing the quality of AI-generated content can be challenging, as there may not be a single "correct" output. Researchers must rely on a variety of evaluation metrics, which may not always align with human perception.
2. Data Privacy and Security Issues
Data privacy and security are critical concerns in the world of generative AI. As models learn from large amounts of data, they may inadvertently memorize sensitive information, such as personal details or confidential information. Ensuring that AI-generated content does not expose private data or compromise security is an ongoing challenge for researchers and practitioners.
FAQs
1. What is Generative AI?
Generative AI is a type of artificial intelligence that can create new content, like images, text, or music, by learning from existing data and making predictions.
2. How does Generative AI work?
It works using advanced machine learning algorithms, like deep learning and neural networks, to analyze patterns in data and generate original content based on those patterns.
3. What are some popular applications of Generative AI?
Popular applications include creating realistic images, writing text or poetry, generating music, designing products, and enhancing creativity in various fields.
4. What is the difference between Generative AI and other AI types?
Generative AI creates new content, while other AI types, like discriminative AI, focus on classifying and predicting outcomes based on existing data.
5. Are there any ethical concerns with Generative AI?
Yes, ethical concerns include potential misuse for creating deepfakes, spreading false information, and copyright infringement. Responsible use and regulation are essential to address these issues.