What is Connectionism?
Connectionism is an approach within the field of cognitive science that hopes to explain mental phenomena using artificial neural networks (ANN).
It's like trying to mimic how the human brain operates, using a vast network of interconnected nodes or "neurons."
Historical Context
The roots of connectionism date back to the early 20th century but it gained substantial momentum in the 1980s with the reinvigoration of interest in neural networks.
It was partly because of the limitations observed in symbolic AI approaches.
Key Concepts
At its core, connectionism involves concepts like pattern recognition, distributed representation, and parallel processing, closely mirroring the assumed operations of the human brain.
Connectionism vs Symbolic AI
Unlike Symbolic AI, which relies on high-level, human-understandable symbols to process information, Connectionism processes information through patterns and distributed representations.
The Brain Analogy
Just as neurons in the human brain strengthen or weaken their connections based on stimuli, neural networks in connectionism adjust the weights of their connections based on the input data they receive.
Why is Connectionism Important?
Understanding the significance of Connectionism can offer insights into its impact on AI development.
Mimicking Human Cognition
Connectionism's approach to mimicking human brain functions holds the promise of developing systems that can perform complex tasks akin to human cognition.
Solving Non-Linear Problems
Its ability to handle non-linear problems and patterns makes it indispensable in fields requiring pattern recognition, like speech and image recognition.
Parallel Processing
Connectionism models can perform multiple calculations simultaneously, akin to parallel processing in the human brain, which allows for faster processing of information.
Learning and Adaptation
Neural networks are capable of learning from the data they are exposed to and adapting their responses accordingly, making systems more intuitive and smarter over time.
Handling Ambiguity
Connectionism is adept at dealing with ambiguous or incomplete data, making it valuable in real-world applications where data is seldom perfect.
Where is Connectionism Applied?
The application areas of Connectionism span across various fields, demonstrating its versatility.
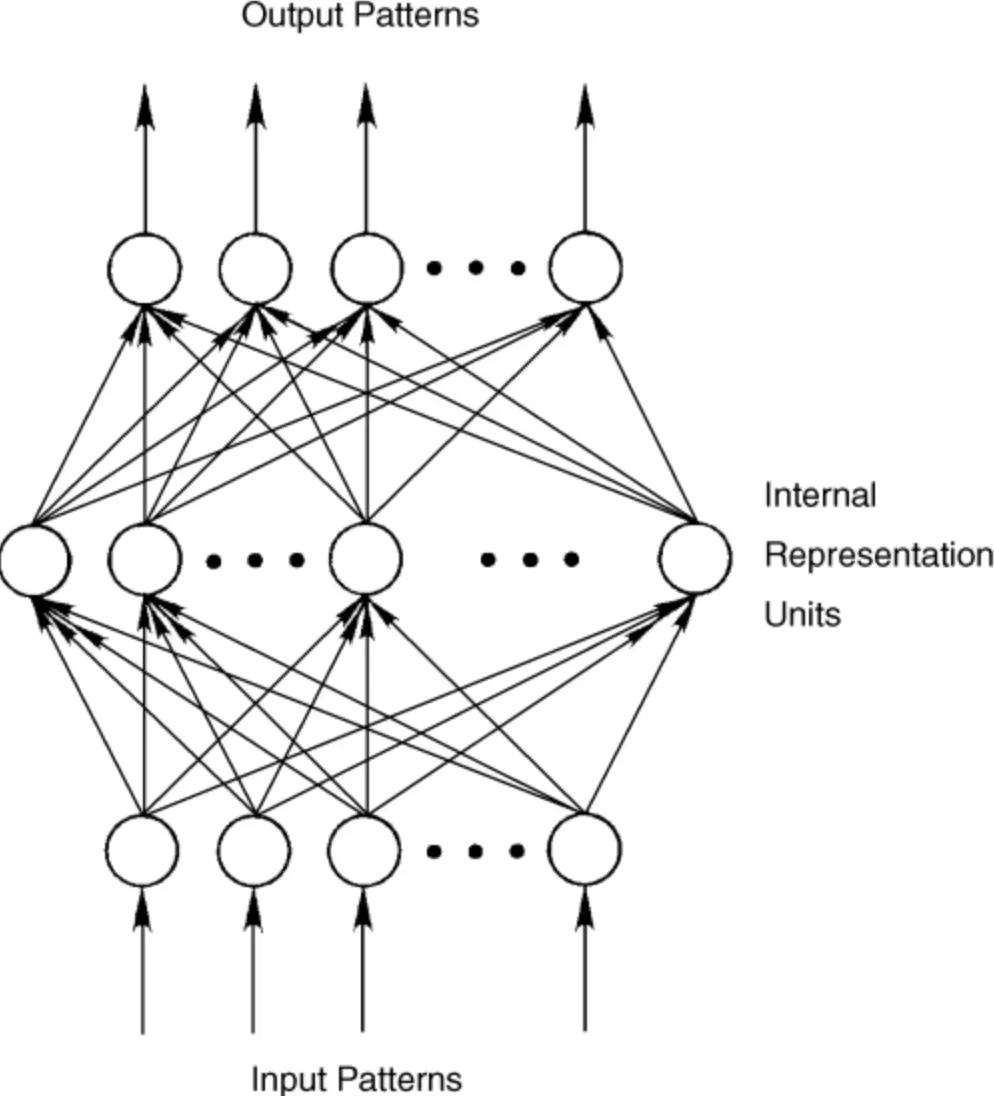
Image and Speech Recognition
Connectionism models have revolutionized how machines interpret human languages and visuals, leading to advancements in AI assistants and automated photo tagging.
Robotics
Robots equipped with connectionist systems can learn from their environment and interactions, becoming more adept at navigating and performing tasks.
Data Mining
Connectionism aids in extracting patterns and valuable information from large datasets, enhancing decision-making processes in businesses.
Medical Diagnosis
AI systems based on connectionist models can assist in predicting diseases and medical conditions by recognizing patterns in patient data.
Natural Language Processing (NLP)
Connectionist models are fundamental in translating languages, generating text, and understanding human speech in real-time.
How does Connectionism Work?
Let's peel back the layers to understand the mechanics of Connectionism.
Neural Networks
At the heart of connectionism lie neural networks, which are composed of input and output layers, as well as one or more hidden layers connecting them.
Training Algorithms
Neural networks learn through training algorithms like backpropagation, which adjusts the weights of connections based on errors in output.
Activation Functions
These functions determine whether a neuron should be activated, based on the strength of its input signals, influencing how data flows through the network.
Weights and Biases
Each connection in a neural network has an associated weight and bias, determining the importance of inputs and the threshold for neuron activation.
Learning and Adaptation
Through processes like reinforcement learning, networks can adapt and improve over time, fine-tuning their responses to inputs.
When did Connectionism Gain Popularity?
Tracing back to when Connectionism started making waves helps us understand its evolution.
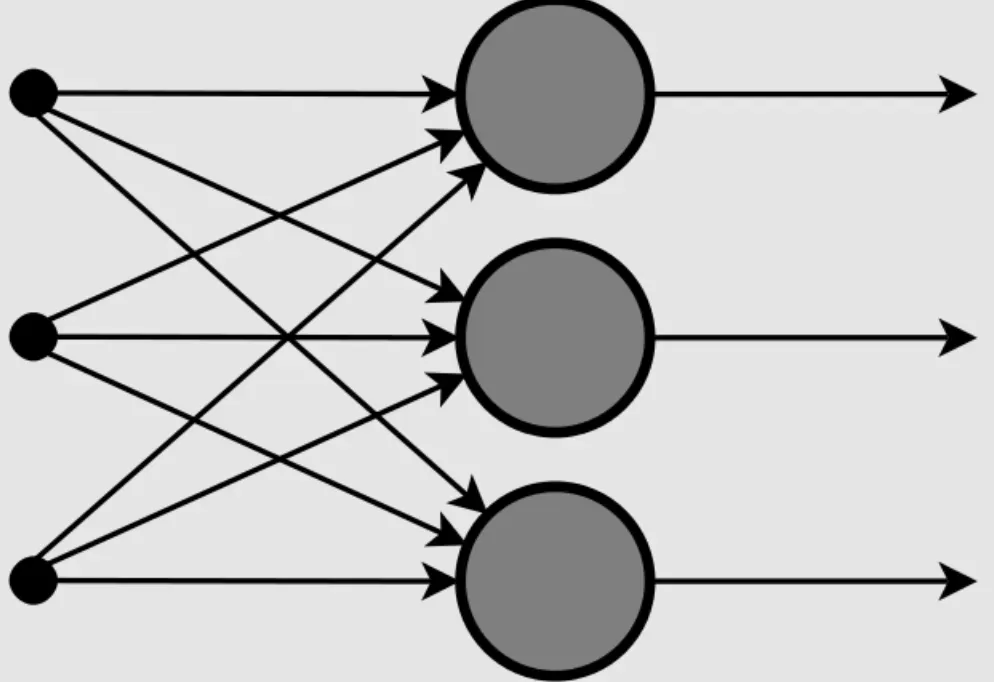
Early Developments
While the foundational concepts date back to the early 20th century, it wasn't until the 1980s that significant advancements in computing power and algorithms rejuvenated interest in neural networks.
The AI Winter
Connectionism offered a new direction during the so-called AI winters, when progress in traditional AI approached a standstill due to limitations in technology and methodologies.
Revival in the 1980s
The introduction of the backpropagation algorithm in the 1980s by Geoffrey Hinton and others marked a turning point, providing an efficient way for neural networks to learn from data.
Rise of Deep Learning
The advent of deep learning in the early 21st century, a subset of connectionism, marked a significant leap forward, with neural networks going deeper and becoming more complex.
Current Renaissance
Today, connectionism underpins many state-of-the-art technologies in AI, from autonomous vehicles to sophisticated chatbots, reflecting its widespread acceptance and application.
Who is Behind Connectionism?
Understanding the key figures and organizations in connectionism gives a face to the theoretical framework.
Pioneers
Researchers like Warren McCulloch and Walter Pitts laid the early groundwork with their models of neural networks in the 1940s.
Modern Contributors
Geoffrey Hinton, Yann LeCun, and Yoshua Bengio, often referred to as the "Godfathers of AI," have been instrumental in the development of deep learning technologies.
Research Institutions
Institutions like MIT, Stanford, and the University of Toronto have been central to advancing connectionist theories and technologies.
Tech Giants
Companies such as Google, Facebook, and IBM have heavily invested in connectionist research, applying its principles to develop cutting-edge technology.
Open Source Community
The open-source community has also played a pivotal role in connectionism's growth, providing accessible tools and frameworks for neural network development.
Challenges in Connectionism
Despite its advancements, connectionism faces several challenges that impact its development and application.
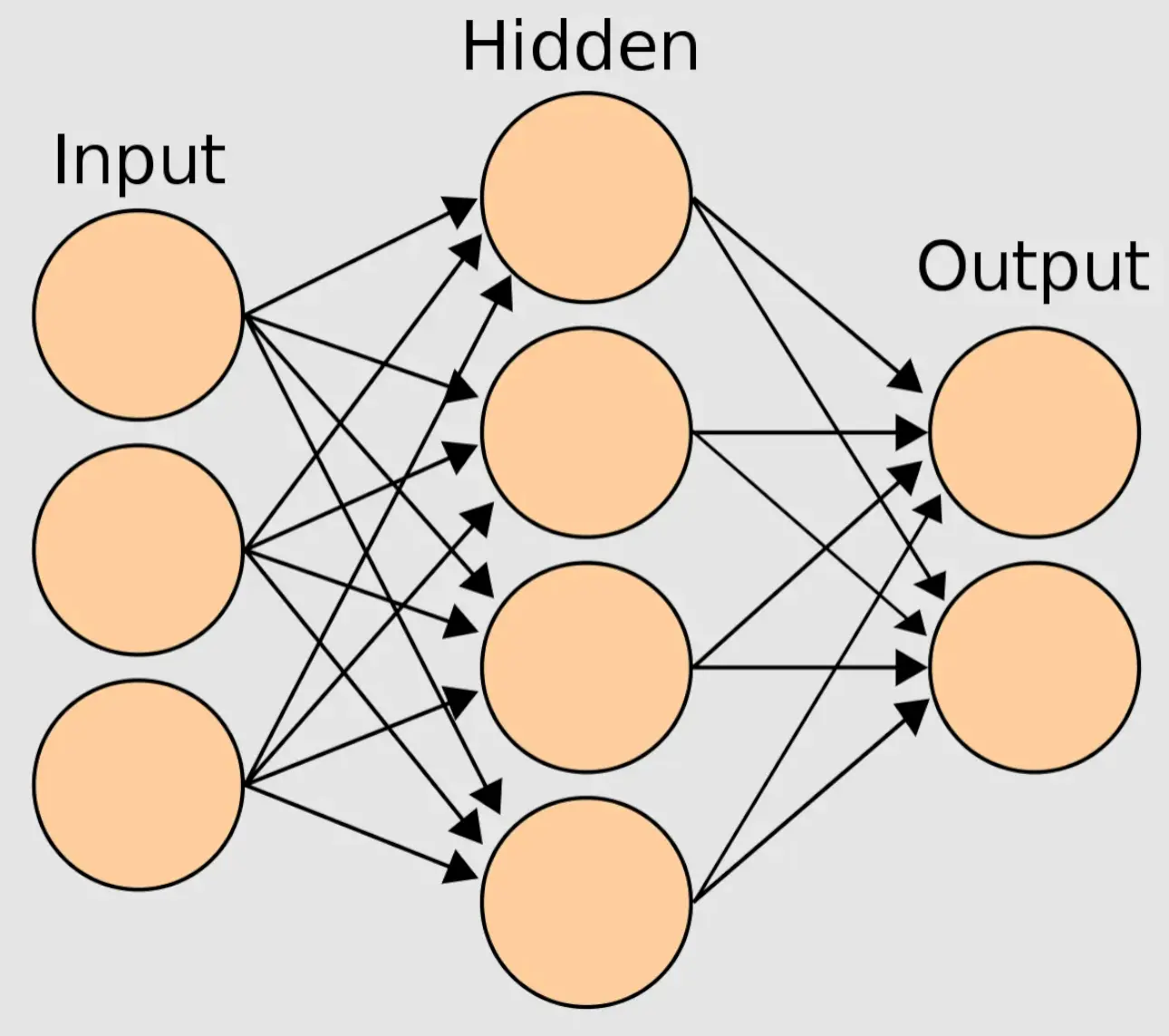
Computational Resources
The training of complex neural networks requires significant computational resources, which can be a barrier to entry for some organizations and researchers.
Interpretability
Neural networks are often criticized for being "black boxes," where the decision-making process is not transparent or easily understood.
Overfitting
Connectionist models can sometimes become too tailored to their training data, losing the ability to generalize to new, unseen data.
Data Bias
AI systems are only as unbiased as the data they're trained on. Connectionist models can inadvertently propagate or amplify biases present in their training data.
Ethical Considerations
The deployment of connectionist models, especially in sensitive areas like surveillance and personal data analysis, raises ethical and privacy concerns.
Best Practices in Connectionism
Adhering to best practices ensures the effective and ethical use of connectionist models.
Data Diversity
Ensuring training data is diverse and representative can mitigate bias and improve the model's ability to generalize.
Regularization Techniques
Implementing techniques like dropout can help prevent overfitting, making models more robust.
Interpretability Efforts
Working towards making neural networks more interpretable helps in understanding and trusting their decisions.
Ethical Guidelines
Adopting ethical guidelines for AI development and deployment is crucial to ensure technology serves the greater good without infringing on privacy or rights.
Continuous Learning
Given the rapidly evolving nature of connectionism, continuous learning and adaptation are necessary for those working in the field.
Trends in Connectionism
Staying atop trends in connectionism provides a glimpse into its future direction.
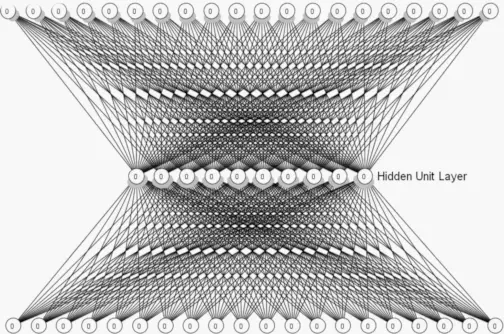
AI Explainability
Increasing emphasis is being placed on making AI systems more explainable and transparent.
Energy-efficient Computing
Developing more energy-efficient neural networks is a priority, considering the environmental impact of training large models.
Neuromorphic Computing
Research in creating hardware that mimics the neural structures of the human brain could revolutionize connectionist models.
Quantum Neural Networks
Exploring the intersection of quantum computing and neural networks offers potential breakthroughs in processing capabilities.
Integration with Symbolic AI
Combining connectionist models with symbolic AI could lead to more powerful and versatile AI systems.
Connectionism, with its vast web of neurons and revolutionary approach to problem-solving, continues to be a cornerstone of artificial intelligence. Its journey from theoretical musings to the backbone of modern AI is a testament to the relentless pursuit of understanding and emulating the intricacies of the human mind.
As we venture further into the future of technology, connectionism remains a key player, shaping the trajectory of AI development and application.
Frequently Asked Questions (FAQs)
What Distinguishes Connectionism from Traditional AI Approaches?
Connectionism models AI on human neural networks, emphasizing parallel processing and distributed representation, unlike traditional rule-based AI.
How Does Connectionism Model Learning and Memory?
It models them through the adjustment of synaptic weights within a network, similar to learning and memory formation in the brain.
Can Connectionism Handle Ambiguous or Incomplete Information?
Yes, connectionist networks like neural networks can generalize from learned data, making them robust to noise and incomplete information.
What's the Significance of Neural Networks in Connectionism?
Neural networks are the core architecture in connectionism, designed to replicate the brain's interconnected neurons for information processing.
How Does Connectionism Contribute to AI Explainability?
Connectionism, through layers of a neural network, can be opaque, often leading to the AI explainability problem, contrary to rule-based systems.