What is Fine-Tuning?
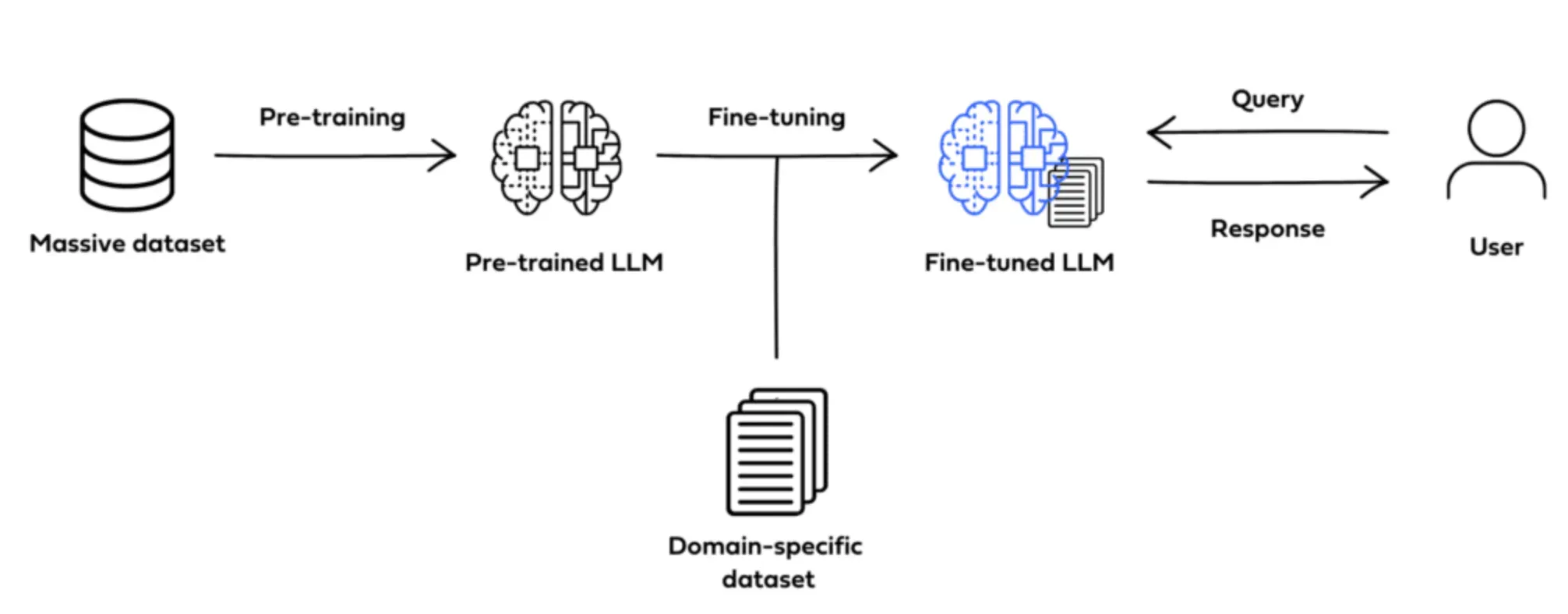
When we speak of fine-tuning in the context of LLMs, we refer to the process in which a pre-trained model continues its training on a specific dataset.
Its goal is to prepare the model for specific tasks and adapt to the complexities and nuances of the new dataset—a bit like prepping an all-round athlete for a specific sport.
Fine-Tuning Vs. Training from Scratch
Fine-tuning is like giving an extra edge to the pre-trained model, a quick brush-up to adjust its parameters, unlike a complete training overhaul.
This means saving effort, time, and computational resources which could be exhaustive if you were training from square one.
Components of Fine-Tuning
When fine-tuning an LLM, the retraining targets several components or parameters of the model, such as weights and biases, to better align its response patterns with the specific task or dataset at hand.
This might remind you of a musical number's fine adjustment before the curtain goes up.
Significance of Fine-Tuning
Fine-tuning is the final critical step that adds another layer of specificity to the pre-trained model.
It's like tailoring a generic coat to fit the individual perfectly, making fine-tuning a valuable technique for creating application-specific models.
Why is Fine-Tuning Necessary?
Fine-Tuning isn't just some extra perk—it's a necessary step in reaping the best results. Let's see why.
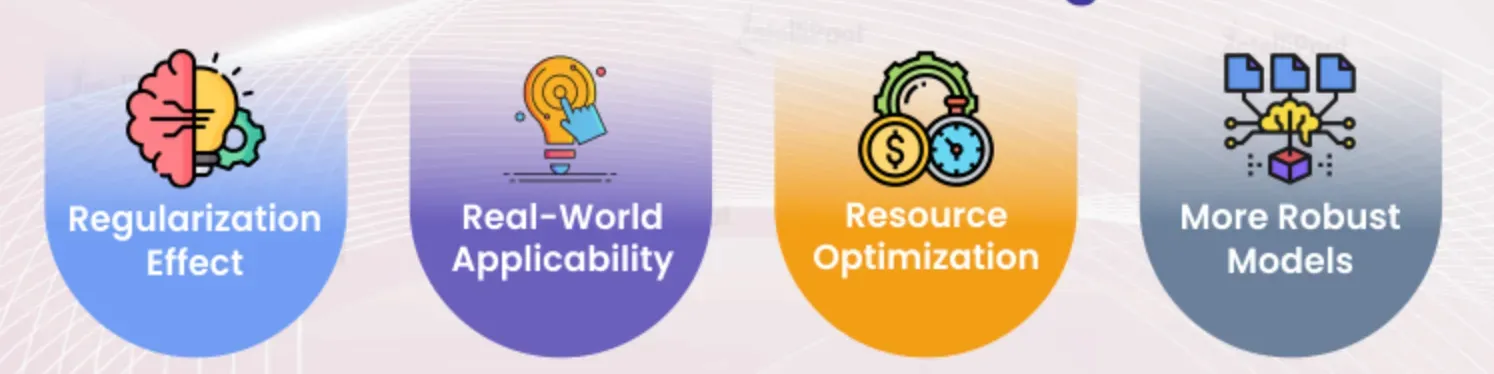
Customized Language Understanding
Fine-tuning is quite like editing a translated text—it helps bring out the nuances that might have been lost in translation.
It enhances the model's performance on specific tasks that might fall out of the boundary of the pre-training data, ensuring a more custom-tailored language understanding.
Efficient Use of Resources
Instead of going through the computationally-intensive process of creating a new model, you take an already well-developed model and make a few adjustments, saving resources and time.
Coping with Data Variations
Diverse datasets pose different challenges and require understanding of unique language styles and nuances. Hence, fine-tuning acts like a language tutor helping LLMs to adjust its understanding to these variations.
Rapid Deployment
In the fast-paced world of technology, getting models off the ground fast matters. Luckily, with a pre-trained model, fine-tuning paves the way for faster deployments without sacrificing efficacy.
Where is Fine-Tuning Used?
Fine-Tuning isn't confined to one niche—it has a wide and varied fan base. Let's see where it finds its relevance.
Sentiment Analysis
In sentiment analysis, fine-tuning helps LLMs break down text and understand sentiment behind it more accurately. Much like catching subtle cues in a conversation, it helps the model interpret the underlying tone.
Text Classification
Fine-tuning is a great ally in adapting LLMs models to accurately classify specific types of documents or content pieces. Just like having a librarian who knows exactly where to file each book!
Named Entity Recognition
With tasks like named entity recognition, fine-tuning contributes in speeding up and enhancing the process by understanding the specificities of the dataset.
Conversational AI
Fine-tuning significantly enriches Conversational AI. It's like equipping chatbots and virtual assistants with an in-depth understanding of language to provide more responsive and accurate responses, creating a more human-like conversation.
How Does Fine-Tuning Work?
Here's the process behind the magic of fine-tuning, step by step.
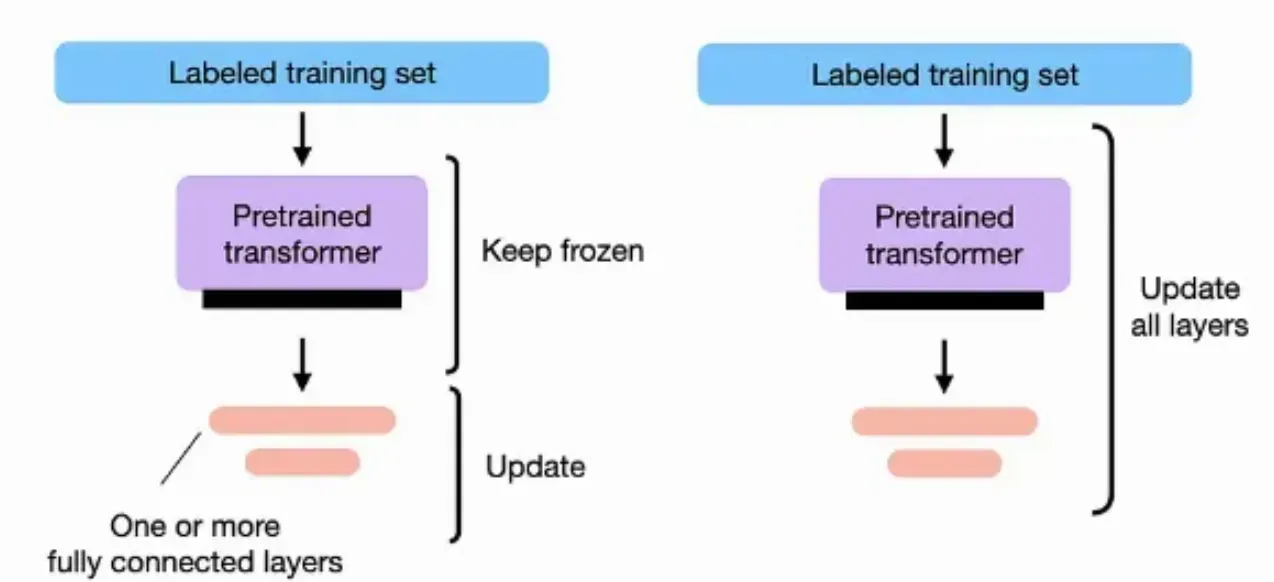
Initialization
The journey starts with a pre-trained model already learned general language features. Just like a journeyman getting ready to master his craft.
Adjustment
The fine-tuning process involves adjusting the model's parameters like tweaking the gears of a watch to ensure perfect timing.
Monitoring
We then observe how the model learns by monitoring the changes made and tweaking them if necessary. It's the process of catching the nuanced manifestations of the new learning.
Evaluation
We check how well the model learned through its fine-tuning using specific metrics. Did it cross the finish line? If yes, how well did it perform? This step ensures that the model is ready to face real-world tasks.
Frequently Asked Questions (FAQs)
What is the difference between fine-tuning and training a model from scratch?
Fine-tuning modifies a pre-trained model for a specific task, while training from scratch involves training a model from random initial weights. Fine-tuning leverages pre-existing knowledge, while training from scratch requires training on a new dataset.
What if I don't have access to a pre-trained model?
If a pre-trained model is not available, training from scratch might be necessary. However, fine-tuning allows you to benefit from the knowledge and expertise obtained from training on massive and diverse datasets.
Can I fine-tune a model for multiple tasks simultaneously?
In most cases, it's better to fine-tune a model for a single task to ensure optimal performance. Fine-tuning for multiple tasks simultaneously can lead to interference and decreased performance.
How do I choose the right pre-trained model for fine-tuning?
Select a pre-trained model that aligns closely with your task in terms of architecture and domain expertise. Also, consider models that have achieved state-of-the-art results in related tasks.
How can I avoid overfitting during fine-tuning?
To avoid overfitting, use techniques like regularization, dropout, and early stopping. Also, monitor the model's performance on validation data and adjust hyperparameters accordingly.