WHAT is Pre-Training?
Pre-training refers to the initial stage in machine learning models where the model is exposed to a massive dataset to learn fundamental patterns. It's like a toddler learning to recognize objects before learning to read.
Stages in Pre-Training
Typically, pre-training comprises two stages - feature learning and fine-tuning. Feature learning involves exposure to vast amounts of unlabelled data, while fine-tuning involves a smaller set of labelled data for specific tasks.
Significance of Pre-Training
Pre-training empowers the model with a solid foundation of language understanding. It equips the model with a general understanding of language patterns before training it on task-specific data.
Pre-Training in Various Machine Learning Models
Numerous machine learning models utilize pre-training, including BERT, GPT-2 and 3, and RoBERTa, to up their game in language understanding tasks.
WHY Do We Need Pre-Training?
The process of pre-training might seem daunting, but its importance can't be understated. Let's explore why.
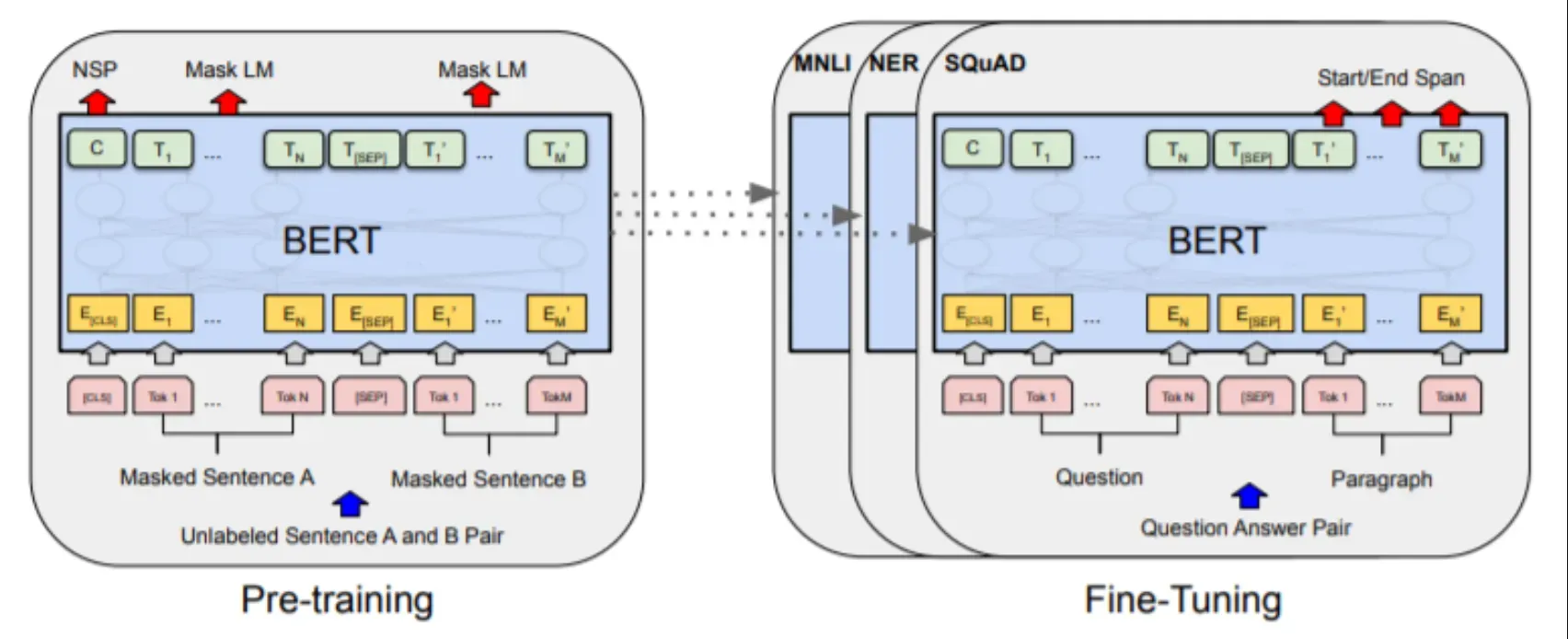
Building Stronger Foundations
Pre-training equips models with a thorough understanding of language features. This stronger foundation translates into superior performance when the model is fine-tuned on task-specific data.
Improving Generalization
Pre-training enables models to better generalize across different tasks by learning from a broader range of data, thus improving transfer learning capabilities.
Reducing Overfitting Risk
By exposing the model to an extensive dataset, pre-training reduces the risk of overfitting when fine-tuning on a specific, smaller dataset.
Saving Computational Resources
Arguably, one of the biggest perks of pre-training is resource-saving. By reusing pre-trained models and fine-tuning them on specific tasks, we can expedite model training while conserving computational resources significantly.
WHO Uses Pre-Training?
Whether you are a dabbling novice or a seasoned veteran of the machine learning universe, navigating the who's who in the vast plain of pre-training is a valuable exercise.
Data Scientists and AI Engineers
Pre-training is a boon to those who build, refine, and regulate machine learning models by providing them with a springboard for advanced model performance.
Researchers
Researchers find pre-training valuable for devising novel algorithms, improving existing models, or investigating the depths of unsupervised learning methodologies.
Tech Companies
Various technology companies, big or small, employ pre-training in their AI solutions to fuel cutting-edge innovations and iterate on their product offerings swiftly.
Individual Users
Even individual users or beginners in machine learning and AI can harness the power of pre-training by utilizing readily available pre-trained models for their projects.
WHEN Should We Use Pre-Training?
Pre-training isn't a one-size-fits-all solution. Defining the right time to use pre-training can help you yield optimal results.
When Dealing with Large Datasets
One should opt for pre-training when dealing with large and diverse datasets. Pre-training helps draw comprehensive language patterns and features from this data.
When Building Robust Language Models
When it comes to building robust language models like BERT or GPT-3, pre-training is indispensable. It provides these models with a fundamental understanding of language.
When Lack of Task-Specific Data
When the amount of task-specific data is limited, pre-training the model on a large corpus can be beneficial to improve model performance later.
When Time and Computational Resources are Limited
When time and computational resources are constrained, using a pre-trained model, and fine-tuning it on the task-specific data saves both.
WHERE is Pre-Training Implemented?
The fascinating world of pre-training isn't confined to a certain area. It finds application in various fields of machine learning.
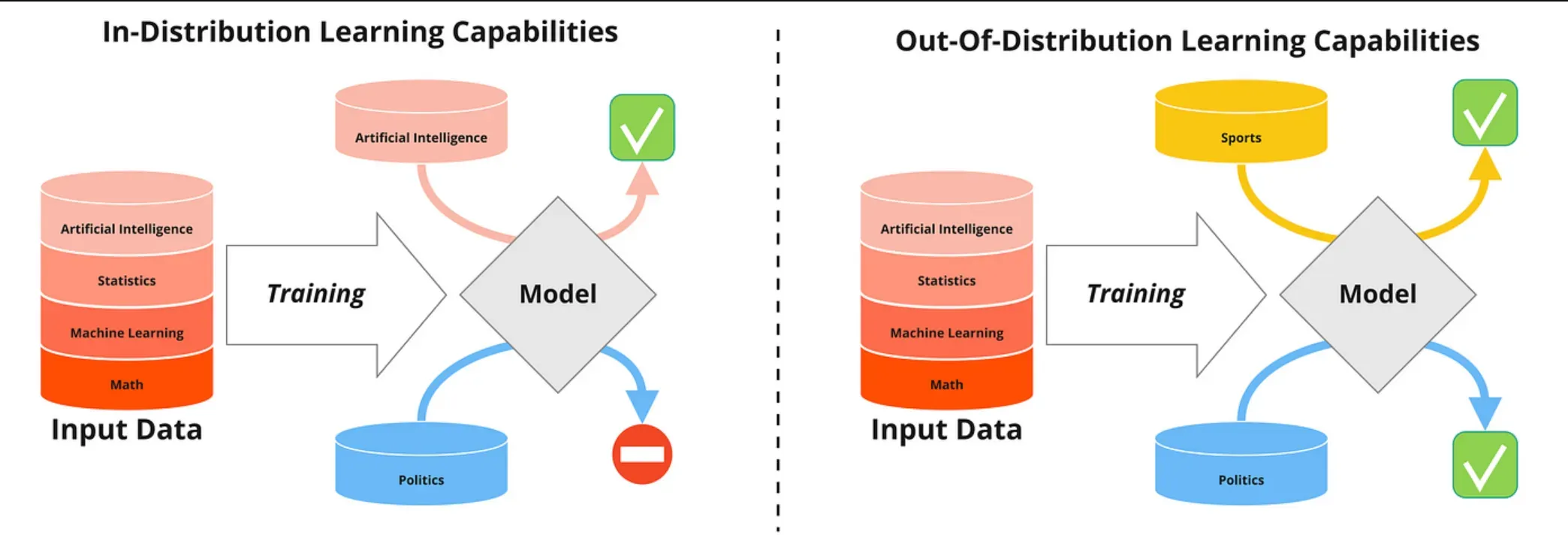
In Building AI Models
Pre-training forms the building blocks of AI models, including machine translation models, computer vision models, and voice recognition models.
In Fine-Tuning Tasks
Pre-training is the predecessor that sets the stage for fine-tuning tasks, allowing models to excel in specific tasks ranging from sentiment analysis to text extraction and more.
In Transfer Learning Approaches
In the realm of transfer learning, pre-training finds its place by providing a base model that can be adapted and fine-tuned for various tasks.
In AI-Based Applications
Pre-training plays a pivotal role in real-world AI-based applications like voice assistants, search engines, and AI chatbots, contributing to a more intuitive, human-like interaction.
HOW is Pre-Training Executed?
Embarking on the pathway of pre-training execution might seem arduous, but understanding each step will clear the fog and help pave the way.
Choosing the Right Dataset
Before diving into pre-training, one must choose an appropriate, large-scale dataset. This dataset should be diverse and cover a broad spectrum of language patterns to inculcate a comprehensive understanding.
Performing Feature Learning
Once the dataset is in place, the model goes through the feature learning phase, where it learns the intricate nuances of language, syntax, grammar, context, and more.
Fine-Tuning Phase
Post pre-training, the fine-tuning phase follows where the pre-trained model is further trained on a smaller, task-specific dataset. The model refines its capabilities for specific tasks during this phase.
Evaluate and Utilize the Model
Finally, after performing pre-training and fine-tuning, the model is evaluated on unseen data. Post-evaluation, the model is ready to be put to use in real-world applications.
Best Practices for Pre-Training
As we venture into the territory of pre-training, it's important to equip ourselves with some best practices. These guidelines will assist you with an efficient and effective pre-training process.
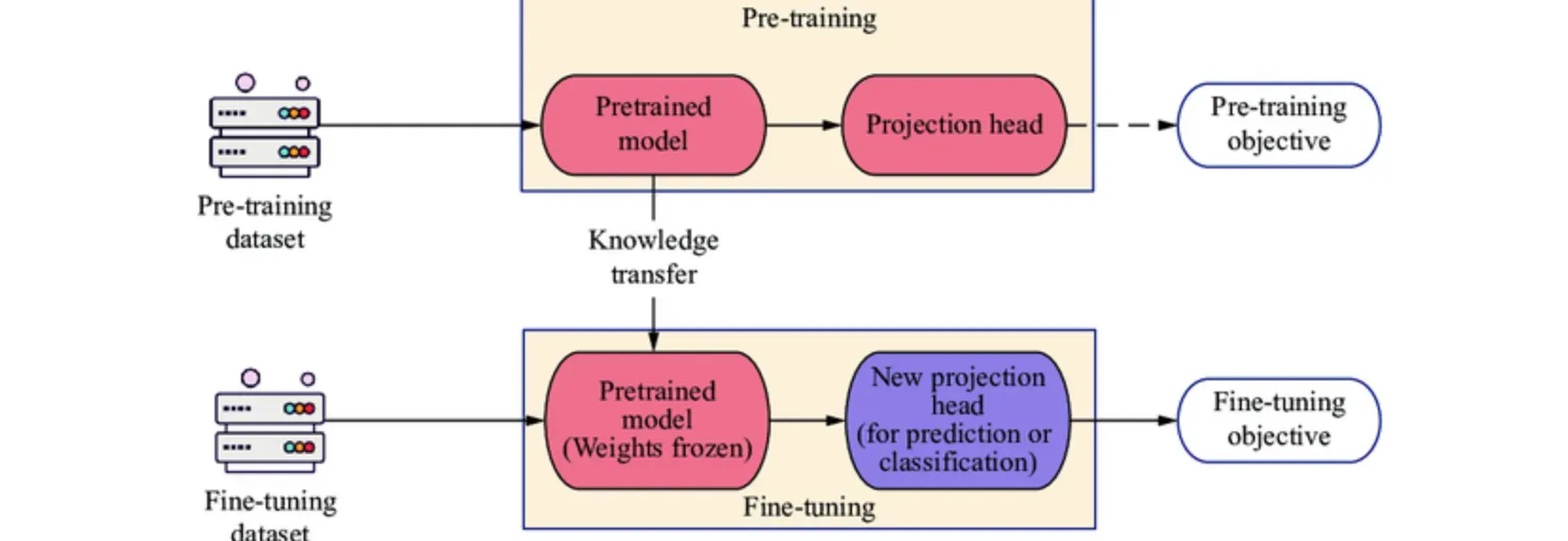
Choosing the Right Dataset
Selecting an appropriate dataset for pre-training can make or break your model's eventual performance. Aim for a large, diverse dataset that represents a broad range of language patterns and contexts. Consider public datasets like Wikipedia or the Common Crawl.
Matching Pre-Training and Fine-Tuning Domains
If possible, ensure the domains of your training and fine-tuning datasets are similar. For instance, if you're fine-tuning a model on medical texts, pre-training on a general language corpus and a corpus of medical literature might produce a better-performing model.
Cautious Use of Regularization
During pre-training, care should be taken to avoid overuse of regularization techniques such as dropout or weight decay. While these techniques can be beneficial to prevent overfitting, too much regularization can hinder the model’s ability to learn from the data.
Monitoring and Evaluating Performances
Regularly monitor the performance of your model during pre-training. Watch out for signs of overfitting, and evaluate the model on held-out validation data. By doing so, you can adjust training hyperparameters in a timely manner if required.
Frequently Asked Questions (FAQs)
Does pre-training in NLP improve the accuracy of NLP models?
Yes, pre-training improves model accuracy by providing a better understanding of language patterns, enhancing generalization, and enabling transfer learning across different NLP tasks.
What are some common use cases for pre-trained NLP models?
Pre-trained NLP models are commonly used for tasks like text classification, sentiment analysis, named entity recognition, text summarization, and machine translation.
How do I select the most suitable pre-trained NLP model for my task?
Consider factors such as task compatibility, language and domain, model architecture, training dataset, model performance, availability, resources required, bias and fairness, documentation, and community support.
What challenges come with pre-training in NLP?
Some challenges include addressing biases in pre-trained models, the computational demands of training large datasets, the need for labeled data for fine-tuning, and overcoming limitations for better performance.
What is the future of pre-training in NLP?
The future of pre-training in NLP involves addressing limitations, developing new techniques to improve pre-training methods, and enhancing language understanding and processing capabilities of NLP models.