What is a Data set?
A dataset is a collection of structured or unstructured data points that can be analyzed to conclude, identify patterns, or train machine learning models.
Datasets come in various formats, such as spreadsheets, databases, or text files, and can be derived from numerous sources, including surveys, web scraping, or sensors. Researchers and professionals utilize datasets for statistical analysis, predictive modeling, and decision-making.
The quality and relevance of datasets are crucial for obtaining accurate and meaningful results from any analysis.
Therefore, data cleaning, preprocessing, and feature selection are essential steps when working with datasets to ensure the data is accurate, consistent, and ready for analysis.
Types of Data Sets:
Data sets can be categorized based on their content or purpose. Some common types of data sets include:
Structured Data Sets:
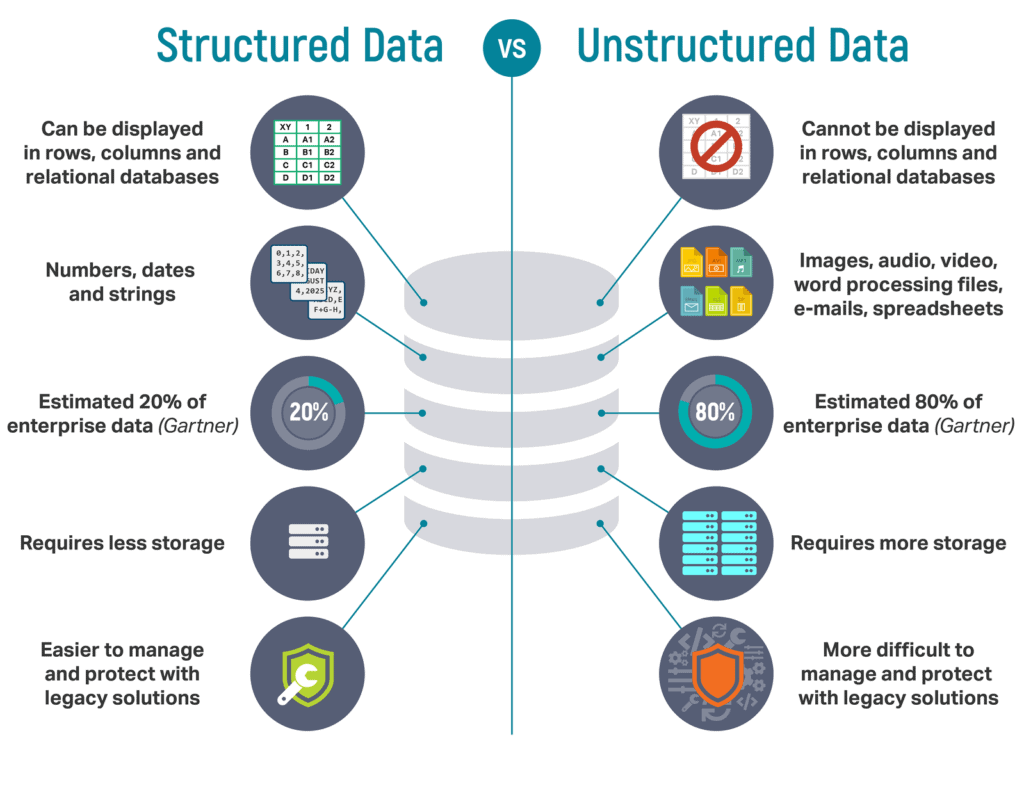
Structured data sets are highly organized and follow a specific format or schema.
They contain predefined fields or columns, and each data point is typically of the same data type.
Examples of structured data sets include spreadsheets, databases, and CSV files.
Unstructured Data Sets:
Unstructured data sets are the opposite of structured data sets and don't follow a specific format.
They contain a variety of data types, such as text, images, and videos, and may not have a defined schema.
Examples of unstructured data sets include social media posts, emails, and customer feedback.
Semi-structured Data Sets:
Semi-structured data sets have some organization but are not as rigid as structured data sets.
They contain a mixture of structured and unstructured data and may have a flexible schema.
Examples of semi-structured data sets include XML and JSON files.
Time Series Data Sets:
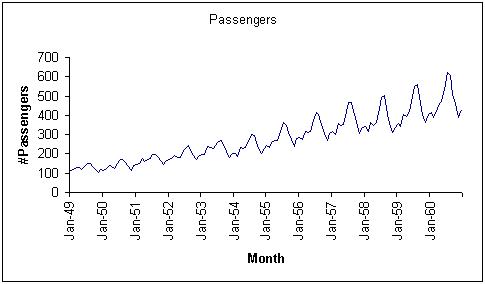
Time series data sets are used to track changes in a specific variable over time.
They are often used in finance, weather forecasting, and other fields where time is a critical factor.
Examples of time series data sets include stock market prices, climate data, and website traffic.
Panel Data Sets:
Panel data sets contain information about a group of individuals or entities over time.
They are often used in social sciences, economics, and marketing research.
Examples of panel data sets include surveys that track respondents over time, longitudinal studies, and customer behavior data.
Cross-sectional Data Sets:
Cross-sectional data sets are used to study a group of individuals or entities at a specific point in time.
They are often used in healthcare, social sciences, and marketing research.
Examples of cross-sectional data sets include census data, market research surveys, and clinical trial data.
Understanding the different types of data sets can help you choose the appropriate analysis techniques and tools for your specific needs.
Data Set Collection and Processing:
Data sets can be collected from various sources, including surveys, sensors, databases, and social media. Once collected, they need to be stored and processed for analysis. Here are some important steps and techniques for data set collection and processing:
Data Set Collection:
Data sets can be collected from primary or secondary sources.
Primary data sources involve collecting data from individuals or entities through surveys, experiments, or observations.
Secondary data sources involve collecting data from existing databases, websites, or publications.
Data Set Storage:
Data sets need to be stored in a secure and organized manner to ensure their integrity and accessibility.
Common storage methods include spreadsheets, databases, and cloud-based solutions.
Data Cleaning and Preprocessing:
Data cleaning and preprocessing are essential steps in preparing a data set for analysis.
Data cleaning involves identifying and correcting errors, inconsistencies, and missing data.
Data preparation is changing data into an analysis-ready format, such as normalizing, scaling, or encoding categorical variables.
Data Processing and Analysis:
Once the data set is cleaned and preprocessed, it can be analyzed using various tools and techniques.
Python and R are popular programming languages for data processing and analysis.
Other tools and techniques include data visualization, machine learning algorithms, and statistical analysis.
Understanding how data sets are collected and processed is crucial for obtaining accurate and meaningful insights. By following best practices for data collection, storage, cleaning, and preprocessing and using appropriate tools and techniques for analysis, you can make the most of your data set and unlock valuable insights.
Suggested Reading:
Multidimensional Scaling
Data Set Ethics and Privacy:
Data collection and usage raise important ethical considerations regarding privacy, consent, and fairness. Here are some key points to consider when dealing with data sets:
Ethical Considerations:
Data collection and usage should be transparent and ethical, with clear data protection and usage guidelines.
Researchers should obtain informed consent from participants and ensure their privacy is protected.
Data usage should not harm or discriminate against individuals or groups based on race, gender, age, or other factors.
Privacy Concerns:
Privacy concerns have become more prevalent with the rise of big data and data analytics.
Personal data can be collected, stored, and analyzed without individuals' knowledge or consent, leading to potential privacy violations.
Data breaches and hacking incidents can also compromise individuals' privacy and expose sensitive information.
Best Practices for Protecting Privacy:
Best practices for protecting individuals' privacy when collecting and analyzing data sets include anonymizing data, limiting data collection to only what is necessary, and obtaining informed consent from participants.
Researchers should also follow established data protection regulations and guidelines, such as GDPR in Europe or HIPAA in the United States.
Data encryption, secure storage, and access control measures can also help protect individuals' privacy and prevent data breaches.
Understanding the ethical considerations and privacy concerns around data collection and usage is crucial for maintaining the trust and credibility of data-driven research and analysis. By following best practices and guidelines for protecting individuals' privacy, researchers can ensure that their work is conducted in a transparent and ethical manner.
Suggested Reading: Cloning Tool
Data Set Visualization:
For comprehending and presenting data findings, data visualization is a crucial tool. When data is presented visually, patterns, trends, and relationships are easier to identify and comprehend. Consider the following points when working with data visualization:
Importance of Data Visualization:
Data visualization is an essential component of data analysis because it facilitates the dissemination of insights and findings to a larger audience.
It enables users to identify patterns and associations that may not be apparent in raw data or tables.
Visualization can also make it easier to identify outliers and anomalies in data.
Common Data Visualization Types:
There are numerous data visualizations, such as scatter plots, histograms, heat maps, and bar charts.
Scatter plots illustrate the association between two variables.
Histograms illustrate the distribution of one variable.
Heat maps depict the intensity of data values in a two-dimensional matrix.
Bar graphs illustrate the comparison of multiple data sets.
Software and Instruments for Data Visualization:
There are numerous tools and applications for data visualization, including Tableau, Excel, R, and Python.
Tableau is a popular tool for data visualization that enables users to create interactive dashboards and visualizations.
Excel also includes data visualization tools, such as charts and graphs.
R and Python are programming languages with robust libraries for data visualization, including ggplot2 and matplotlib.
Data visualization is an important step in the data analysis process that can help to communicate insights and findings to a broader audience. By understanding common types of visualizations and using appropriate tools and software, researchers can create effective visualizations that communicate insights and findings effectively.
FAQs about Data Sets
What is a Data set?
A dataset is a collection of structured or unstructured data points that can be analyzed to conclude, identify patterns, or train machine learning models.
Datasets come in various formats, such as spreadsheets, databases, or text files, and can be derived from numerous sources, including surveys, web scraping, or sensors. Researchers and professionals utilize datasets for statistical analysis, predictive modeling, and decision-making.
The quality and relevance of datasets are crucial for obtaining accurate and meaningful results from any analysis.
Therefore, data cleaning, preprocessing, and feature selection are essential steps when working with datasets to ensure the data is accurate, consistent, and ready for analysis.
Types of Data Sets:
Data sets can be categorized based on their content or purpose. Some common types of data sets include:
Structured Data Sets:
Structured data sets are highly organized and follow a specific format or schema.
They contain predefined fields or columns, and each data point is typically of the same data type.
Examples of structured data sets include spreadsheets, databases, and CSV files.
Unstructured Data Sets:
Unstructured data sets are the opposite of structured data sets and don't follow a specific format.
They contain a variety of data types, such as text, images, and videos, and may not have a defined schema.
Examples of unstructured data sets include social media posts, emails, and customer feedback.
Semi-structured Data Sets:
Semi-structured data sets have some organization but are not as rigid as structured data sets.
They contain a mixture of structured and unstructured data and may have a flexible schema.
Examples of semi-structured data sets include XML and JSON files.
Time Series Data Sets:
Time series data sets are used to track changes in a specific variable over time.
They are often used in finance, weather forecasting, and other fields where time is a critical factor.
Examples of time series data sets include stock market prices, climate data, and website traffic.
Panel Data Sets:
Panel data sets contain information about a group of individuals or entities over time.
They are often used in social sciences, economics, and marketing research.
Examples of panel data sets include surveys that track respondents over time, longitudinal studies, and customer behavior data.
Cross-sectional Data Sets:
Cross-sectional data sets are used to study a group of individuals or entities at a specific point in time.
They are often used in healthcare, social sciences, and marketing research.
Examples of cross-sectional data sets include census data, market research surveys, and clinical trial data.
Understanding the different types of data sets can help you choose the appropriate analysis techniques and tools for your specific needs.
Data Set Collection and Processing:
Data sets can be collected from various sources, including surveys, sensors, databases, and social media. Once collected, they need to be stored and processed for analysis. Here are some important steps and techniques for data set collection and processing:
Data Set Collection:
Data sets can be collected from primary or secondary sources.
Primary data sources involve collecting data from individuals or entities through surveys, experiments, or observations.
Secondary data sources involve collecting data from existing databases, websites, or publications.
Data Set Storage:
Data sets need to be stored in a secure and organized manner to ensure their integrity and accessibility.
Common storage methods include spreadsheets, databases, and cloud-based solutions.
Data Cleaning and Preprocessing:
Data cleaning and preprocessing are essential steps in preparing a data set for analysis.
Data cleaning involves identifying and correcting errors, inconsistencies, and missing data.
Data preparation is changing data into an analysis-ready format, such as normalizing, scaling, or encoding categorical variables.
Data Processing and Analysis:
Once the data set is cleaned and preprocessed, it can be analyzed using various tools and techniques.
Python and R are popular programming languages for data processing and analysis.
Other tools and techniques include data visualization, machine learning algorithms, and statistical analysis.
Understanding how data sets are collected and processed is crucial for obtaining accurate and meaningful insights. By following best practices for data collection, storage, cleaning, and preprocessing and using appropriate tools and techniques for analysis, you can make the most of your data set and unlock valuable insights.
Data Set Ethics and Privacy:
Data collection and usage raise important ethical considerations regarding privacy, consent, and fairness. Here are some key points to consider when dealing with data sets:
Ethical Considerations:
Data collection and usage should be transparent and ethical, with clear guidelines for data protection and usage.
Researchers should obtain informed consent from participants and ensure their privacy is protected.
Data usage should not harm or discriminate against individuals or groups based on race, gender, age, or other factors.
Privacy Concerns:
Privacy concerns have become more prevalent with the rise of big data and data analytics.
Personal data can be collected, stored, and analyzed without individuals' knowledge or consent, leading to potential privacy violations.
Data breaches and hacking incidents can also compromise individuals' privacy and expose sensitive information.
Best Practices for Protecting Privacy:
Best practices for protecting individuals' privacy when collecting and analyzing data sets include anonymizing data, limiting data collection to only what is necessary, and obtaining informed consent from participants.
Researchers should also follow established data protection regulations and guidelines, such as GDPR in Europe or HIPAA in the United States.
Data encryption, secure storage, and access control measures can also help protect individuals' privacy and prevent data breaches.
Understanding the ethical considerations and privacy concerns around data collection and usage is crucial for maintaining the trust and credibility of data-driven research and analysis. By following best practices and guidelines for protecting individuals' privacy, researchers can ensure that their work is conducted in a transparent and ethical manner.
Data Set Visualization:
For comprehending and presenting data findings, data visualization is a crucial tool. When data is presented visually, patterns, trends, and relationships are easier to identify and comprehend. Consider the following points when working with data visualization:
Importance of Data Visualization:
Data visualization is an essential component of data analysis because it facilitates the dissemination of insights and findings to a larger audience.
It enables users to identify patterns and associations that may not be apparent in raw data or tables.
Visualization can also make it easier to identify outliers and anomalies in data.
Common Data Visualization Types:
There are numerous data visualizations, such as scatter plots, histograms, heat maps, and bar charts.
Scatter plots illustrate the association between two variables.
Histograms illustrate the distribution of one variable.
Heat maps depict the intensity of data values in a two-dimensional matrix.
Bar graphs illustrate the comparison of multiple data sets.
Software and Instruments for Data Visualization:
There are numerous tools and applications for data visualization, including Tableau, Excel, R, and Python.
Tableau is a popular tool for data visualization that enables users to create interactive dashboards and visualizations.
Excel also includes data visualization tools, such as charts and graphs.
R and Python are programming languages with robust libraries for data visualization, including ggplot2 and matplotlib.
Data visualization is an important step in the data analysis process that can help to communicate insights and findings to a broader audience. By understanding common types of visualizations and using appropriate tools and software, researchers can create effective visualizations that communicate insights and findings effectively.
FAQs regarding Data Sets
What is a data set?
A data set is an organized collection of data points or observations stored in a structured format. There are numerous applications for data sets, including analysis, modeling, and prediction.
What are a few illustrations of data sets?
Customer transaction data, sensor data, weather data, demographic data, and social media data are examples of data sets. The selection of the data set depends on the particular requirements of the business or researcher.
How does one create a data set?
Creating a data set entails identifying the data sources, collecting and cleansing the data, transforming the data into a usable format, and storing the data in a structured format. It is essential to maintain data quality and uniformity throughout the process.
How do you determine which data set to analyze?
Consider the research question, the type of data required, and the scope of the analysis when selecting an appropriate data set for analysis. It is essential to ensure that the data set is accurate, representative, and relevant to the population.
What are the best practices for data set management?
Best practices for managing data sets consist of documenting data sources, maintaining data quality, ensuring data security and privacy, and adhering to legal and ethical standards. Regular backups and updates are also essential for data integrity.