Bloom has been making waves in the field of natural language processing (NLP) and artificial intelligence. It is the large language model developed by the AI research company Anthropic.
This groundbreaking model represents a significant leap forward in the capabilities of language models. As it pushes the boundaries of what is possible in areas such as text generation, understanding, and analysis.
According to a recent report by Grand View Research, the global natural language processing market size is expected to reach $49.6 billion by 2030, registering a compound annual growth rate (CAGR) of 16.3% from 2022 to 2030 (Source: Grand View Research, "Natural Language Processing Market Size & Share Report, 2022-2030").
Bloom, with its revolutionary architecture and impressive performance, is poised to play a pivotal role in driving this growth and shaping the future of NLP applications.
With its ability to handle complex language tasks with unprecedented accuracy and efficiency, Bloom has captured the attention of researchers, developers, and industry leaders alike, paving the way for a new era of advanced NLP applications.
So continue reading to know more about Bloom as a revolutionary large language model.
An Introduction to BLOOM Language Model
BLOOM stands for BigScience Large Open-science Open-access Multilingual Language Model. It is a groundbreaking project that has been developed through the collaborative efforts of over 1200 participants from 39 countries, including the United States.
In a truly global collaboration, BigScience, in partnership with Hugging Face and the French NLP community, has transcended geographical and institutional boundaries to create this innovative language model.
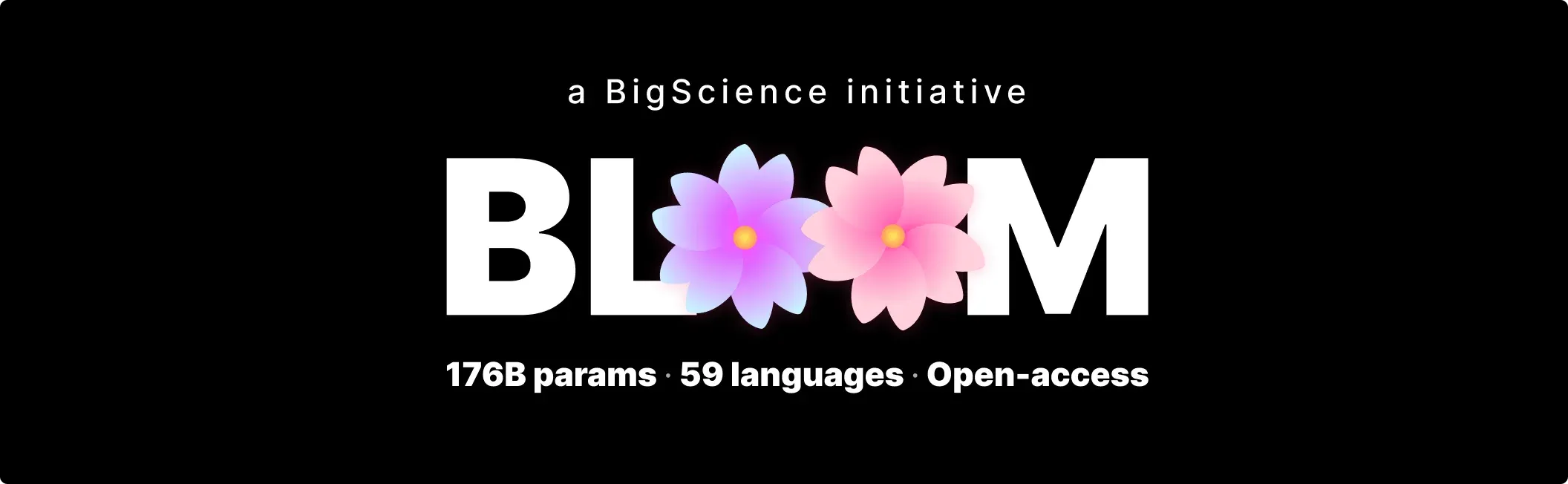
Technical Specifications
When it comes to the technical specifications of BLOOM, there are several key aspects that make it stand out from the crowd.
Model Architecture
BLOOM is built upon the Transformer architecture, specifically utilizing a causal decoder-only model.
This architecture is known for its ability to process sequential data, making it a highly suitable choice for language modeling tasks.
Parameters
One of the most impressive aspects of BLOOM is its vast size. With a staggering 176 billion parameters, this language model is a true technological marvel.
These parameters allow BLOOM to effectively capture and analyze a wide range of linguistic patterns and nuances.
Training Data
To train and fine-tune BLOOM, the developers utilized the ROOTS corpus, an extensive dataset that encompasses 59 languages.
This corpus comprises not only spoken languages but also programming languages, providing a diverse range of linguistic inputs to enhance the model's capabilities.
Comparison of Bloom to Other Large Language Models
When it comes to large language models, there are a few notable players in the field, such as GPT-3 and T5. Let's take a closer look at how Bloom compares to these models.
Bloom vs. GPT-3
GPT-3, or Generative Pre-trained Transformer 3, is a widely renowned language model developed by OpenAI.
While both Bloom and GPT-3 share the Transformer architecture as their foundation, there are some differences worth noting.
One significant distinction between Bloom and GPT-3 lies in their parameters. Bloom boasts an impressive 176 billion parameters, which surpasses GPT-3's 175 billion parameters.
This slightly higher parameter count gives Bloom an edge in terms of model size and complexity.
Another area where Bloom differs from GPT-3 is the training data used. Bloom's training involves the ROOTS corpus, a dataset covering 59 languages, while GPT-3 is trained on a diverse range of internet text.
This distinction means that Bloom's training data is more curated, focused on a specific corpus, which allows it to excel in specific multilingual tasks.
Bloom vs. T5
T5, short for Text-To-Text Transfer Transformer, is a language model developed by Google Research. Bloom and T5 share the Transformer architecture, but they have their own unique characteristics.
In terms of parameters, Bloom's 176 billion parameters far surpass T5's 11 billion parameters. This significant difference in parameter count represents the tremendous scale and complexity of Bloom.
While both Bloom and T5 are capable of generating text, they have different strengths.
Bloom's training on the ROOTS corpus, with its multilingual and programming language coverage, gives it an advantage in handling diverse language tasks compared to T5, which is primarily trained on text from the web.
How Does Bloom Work?
To understand how Bloom operates, let's dive into its architecture and design, as well as how it generates text.
Explanation of Bloom's Architecture and Design
Bloom is built upon the Transformer architecture, specifically a causal decoder-only model. This architecture allows BLOOM to process sequential data.
Thus making it particularly suited for language modeling tasks.
At its core, the Transformer architecture employs self-attention mechanisms. It enables the model to weigh and analyze different parts of the input text during processing.
This attention mechanism aids in capturing complex linguistic relationships and dependencies.
In terms of design, Bloom's primary focus is to provide a large, multilingual language model that encompasses both spoken languages and programming languages.
This design choice facilitates the ability of Bloom to understand and generate text across a wide range of linguistic domains.
How Bloom Generates Text
Bloom generates text through a process known as "autoregressive decoding." Given an initial prompt or input text, Bloom predicts and generates subsequent words based on its learned language patterns.
his process is akin to filling in the blanks, with Bloom leveraging its extensive training on diverse language datasets to generate coherent and contextually appropriate text.
The generation process in Bloom is influenced by the attention mechanism within the Transformer architecture.
By attending to different parts of the input text, the model can incorporate the relevant context and produce more accurate and context-aware text output.
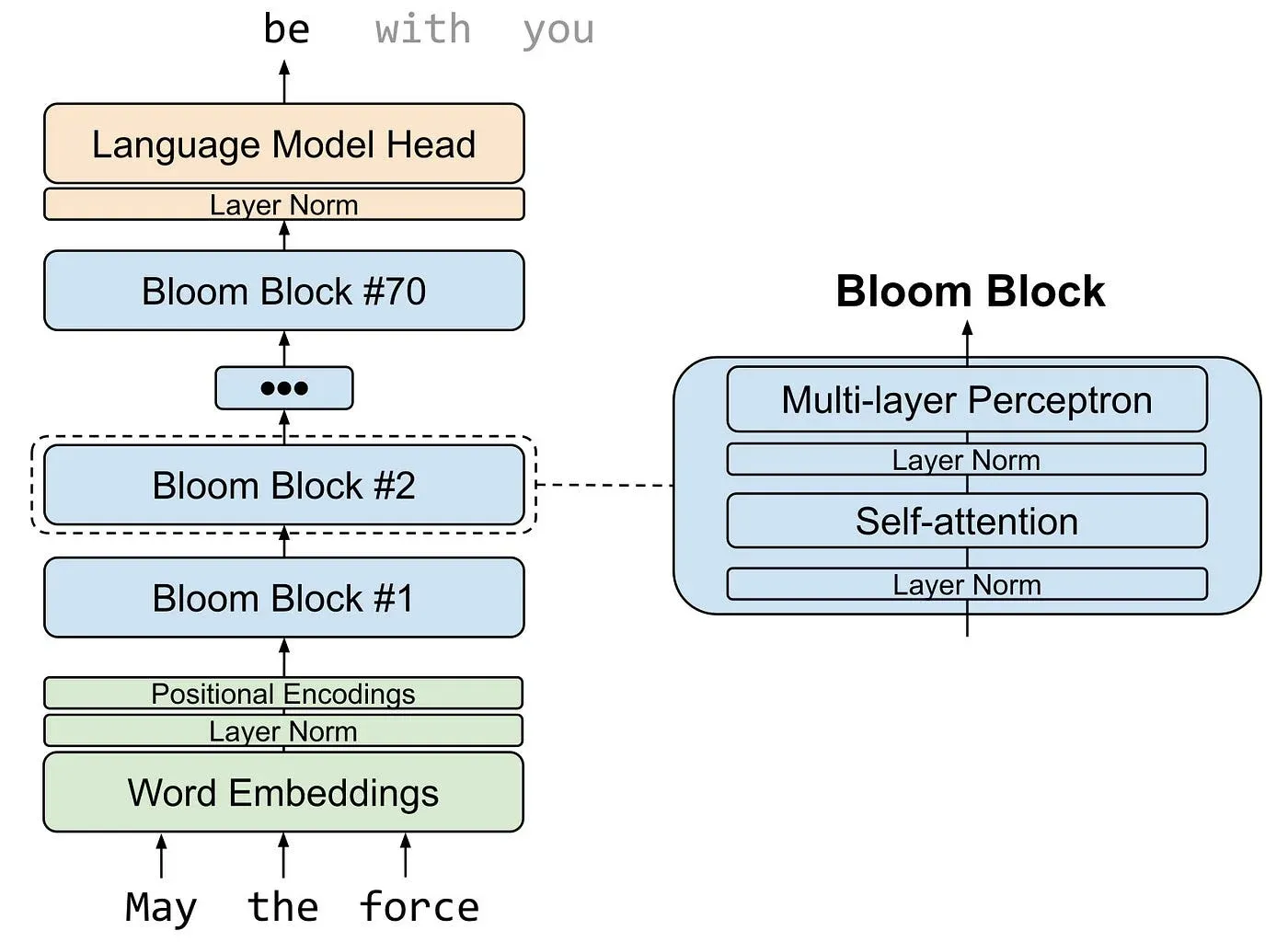
Applications of Bloom
Let's explore the diverse applications of Bloom across various industries and its potential impact on society.
Finance
In the finance sector, Bloom's advanced language processing capabilities can be leveraged for tasks such as sentiment analysis of financial news and reports.
By analyzing large volumes of text data, Bloom can help financial analysts make more informed investment decisions and predict market trends with greater accuracy.
Healthcare
Bloom holds promise for revolutionizing the healthcare industry through applications like medical record analysis, drug discovery, and patient communication.
By parsing through medical texts, Bloom can assist healthcare professionals in diagnosing illnesses, identifying treatment options. It can even generate patient-friendly explanations of complex medical information.
Marketing
Marketers can benefit from Bloom's language generation abilities to create personalized and compelling content for their target audiences.
From generating tailored marketing messages to analyzing customer feedback, Bloom can enhance marketing campaigns and strategies by tapping into linguistic nuances and customer preferences.
Potential Impact of Bloom on Society
The widespread adoption of Bloom in various industries has the potential to bring about significant positive impacts on society as a whole.
Enhanced Efficiency
Bloom is capable of automating language-related tasks and streamlining text analysis processes.
With this, Bloom can boost efficiency across different sectors, leading to faster decision-making, reduced workloads, and improved productivity.
Increased Access to Information
Bloom's multilingual capabilities can help bridge language barriers and facilitate the exchange of information among diverse linguistic communities.
This accessibility to a wealth of knowledge and resources can empower individuals worldwide, promoting learning and knowledge sharing on a global scale.
Ethical Considerations
As with any advanced AI technology, the ethical implications of using Bloom must be carefully considered.
Issues such as data privacy, bias in language models, and responsible AI deployment need to be addressed to ensure that Bloom's impact on society is a positive and inclusive one.
Suggested Reading:
Performance and Benchmarks of BLOOM
BLOOM, with its impressive performance and results across various benchmarks, has shown its capabilities as a powerful language model.
Let's explore how BLOOM has achieved remarkable performance, the benefits of multitask prompted fine-tuning, and how the project's training session on the Jean Zay supercomputer exemplifies the power of collective scientific pursuit.
BLOOM Achieving Remarkable Performance
BLOOM has demonstrated exceptional performance across a range of benchmarks, showcasing its effectiveness in natural language processing tasks.
With its vast pre-training data and large model architecture, BLOOM has proven to be proficient in tasks like language modeling, question answering, and text completion.
BLOOM's success can be attributed to its ability to generate coherent and contextually relevant responses while adapting to different prompts and queries.
The model's vast knowledge base and training on diverse datasets have enhanced its understanding of language and allowed it to generate high-quality output in various contexts.
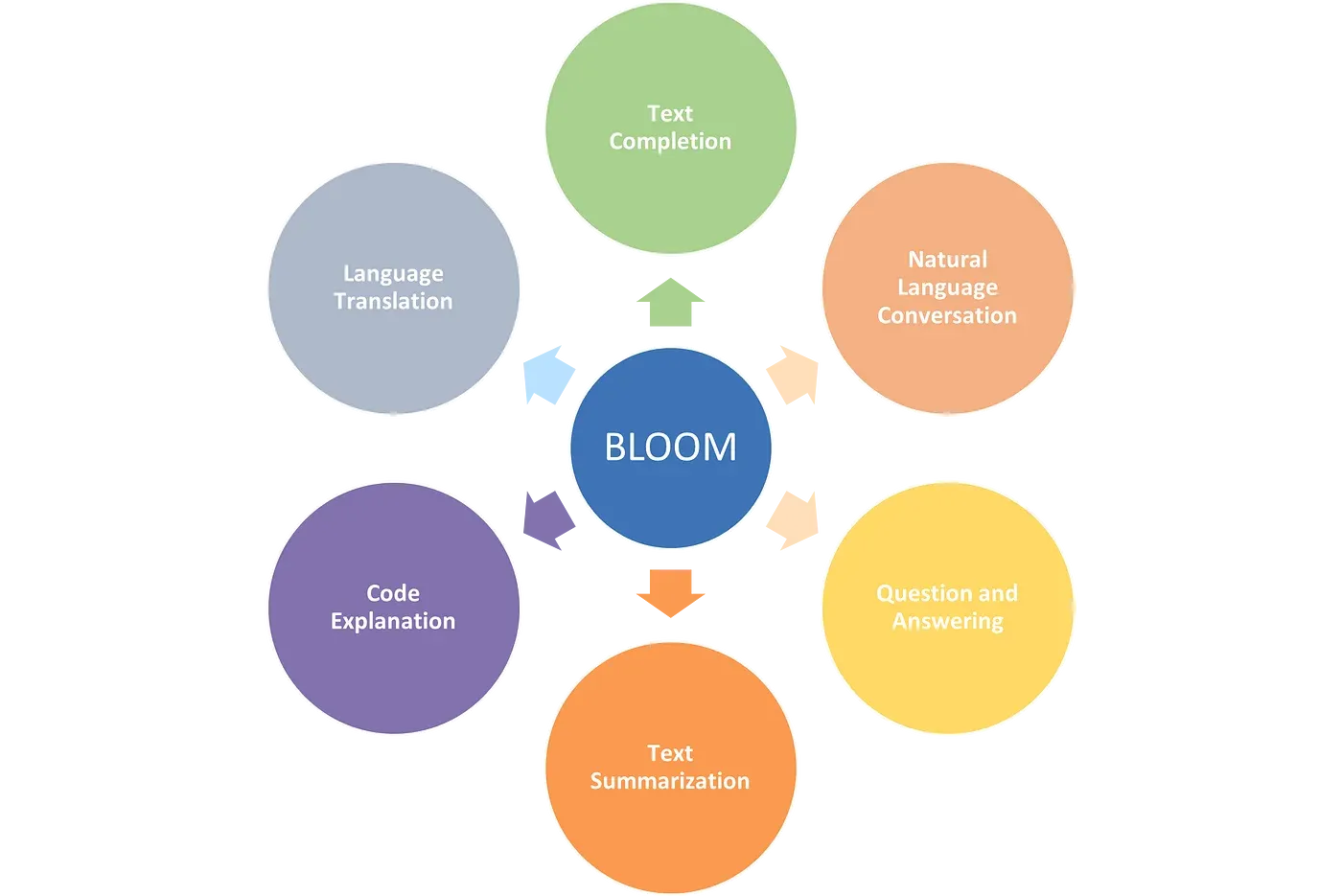
Multitask Prompted Fine-tuning for Improved Results
To further enhance BLOOM's performance, researchers have employed multitask prompted fine-tuning. This technique involves training the model on multiple related tasks simultaneously, leveraging the shared representation learning across these tasks.
By fine-tuning BLOOM using prompts designed for specific tasks, researchers have observed improvements in the model's performance across various benchmarks.
This approach enables BLOOM to excel not only in general language understanding but also in specific domains or tasks. Thus making it a versatile and capable language model.
Exemplifying the Power of Collective Scientific Pursuit
The project's 117-day training session on the Jean Zay supercomputer in Paris serves as an example of the power of collective scientific pursuit.
Training a large language model like BLOOM requires substantial computational resources and collaboration among researchers.
By leveraging the high-performance computing capabilities of supercomputers, researchers were able to train BLOOM on immense amounts of data and fine-tune its performance.
The project highlights the dedication and collaborative efforts of the scientific community to push the boundaries of language modeling and natural language processing.
The successful training of BLOOM on the Jean Zay supercomputer demonstrates the importance of shared resources and collective expertise in advancing AI research.
It underscores the collaborative nature of scientific progress and the potential for accelerated breakthroughs when researchers pool their resources and knowledge.
Challenges of Large Language Models like Bloom
While large language models like Bloom have incredible potential, they also come with their fair share of challenges.
Let's explore some of the ethical concerns surrounding these models and how the community is addressing them.
Bias
One of the prominent ethical concerns associated with large language models is the presence of bias.
These models are trained on vast amounts of data collected from the internet, which often reflect existing biases present in society.
As a result, the generated text may inadvertently reinforce or amplify these biases, leading to potential discrimination or misinformation.
Privacy
Another significant challenge is privacy. Large language models, including Bloom, require access to vast amounts of data to train effectively.
This data may include personal or sensitive information, raising concerns about data privacy and potential misuse.
It is crucial to ensure that user data is handled securely and that proper consent and anonymization practices are implemented to protect individuals' privacy rights.
With all the heavy work of chatbot development already done for you, BotPenguin allows users to integrate some of the prominent language models like GPT 4, Google PaLM, and Anthropic Claude to create AI-powered chatbots for platforms like:
- WhatsApp Chatbot
- Facebook Chatbot
- WordPress Chatbot
- Telegram Chatbot
- Website Chatbot
- Squarespace Chatbot
- woocommerce Chatbot
- Instagram Chatbot
How the Community is Addressing These Concerns
The AI community recognizes the importance of addressing the ethical concerns surrounding large language models.
Efforts are being made to mitigate biases, enhance privacy, and ensure responsible AI deployment. Here are some steps being taken:
Bias Mitigation
Researchers are actively developing techniques to mitigate bias in language models like Bloom.
This includes fine-tuning models on more representative and diverse datasets, employing adversarial training to identify and reduce biases, and exploring methods for debiasing the models' outputs.
Ongoing research and collaboration aim to create models that are more fair, accurate, and unbiased.
Responsible AI Frameworks
To address privacy concerns, frameworks for responsible AI have been proposed. These frameworks emphasize transparency, accountability, and data protection.
Guidelines are being developed to ensure that large language models adhere to ethical standards, including adopting privacy-preserving practices and data anonymization techniques.
By incorporating these principles into the development and deployment of models like Bloom, privacy concerns can be proactively addressed.
Public Input and Governance
The AI community realizes the importance of public input and democratic decision-making in shaping the use of large language models.
Initiatives are underway to involve diverse stakeholders in the development and deployment of these models, ensuring that societal values and perspectives are considered.
Suggested Reading:
Top 10 Bloom LLM Features to Elevate Your Language Processing
Future of Bloom and Large Language Models
Looking ahead, the future of Bloom and similar large language models is promising. These models have the potential to shape the future of natural language processing (NLP) in transformative ways.
Let's explore some predictions for the future and how Bloom can contribute to the evolution of NLP.
Enhanced Understanding and Contextual Awareness
As large language models continue to evolve, they are expected to develop a deeper understanding of language and context.
Future iterations of Bloom may possess the ability to comprehend nuanced meanings, understand complex queries, and provide more accurate and contextually appropriate responses.
This enhanced understanding will enable more natural and effective interactions with users. Thus making these models even more valuable across various applications.
Multimodal Capabilities
The future of large language models involves integrating multimodal capabilities, combining text with other modalities like images, videos, and audio.
This will enable models like Bloom to understand and generate content using a range of media types. Further opening up new possibilities for creative expression, content generation, and communication.
Embracing multimodal capabilities will revolutionize how we interact with and perceive information.
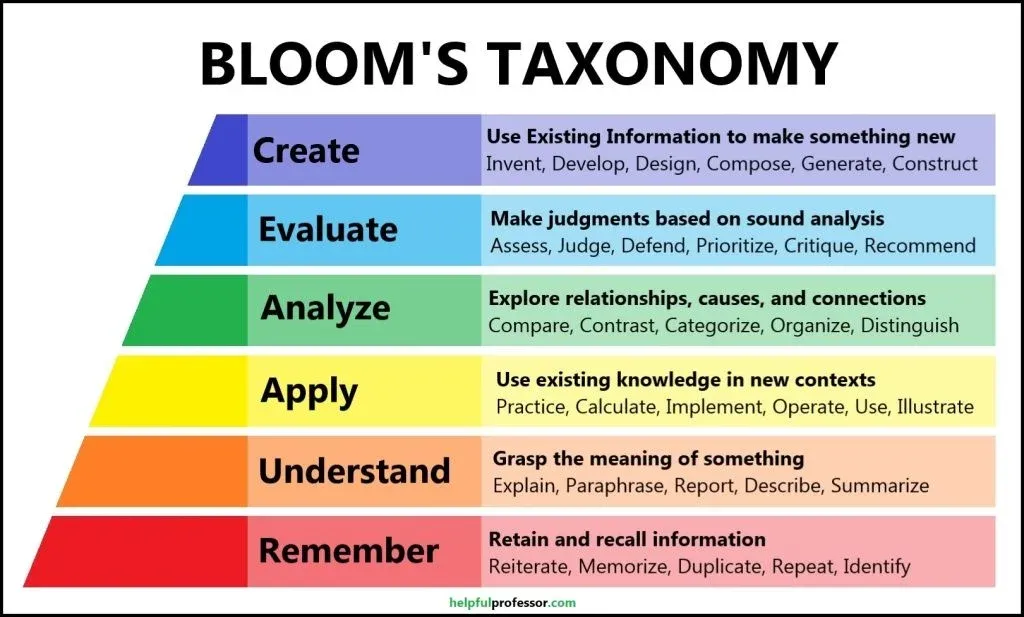
Personalization and User Adaptability
Future iterations of large language models are predicted to become more personalized and adaptable to individual users.
By leveraging user feedback and preferences, models like Bloom can tailor responses and recommendations specifically to each user's needs and interests.
Continued Advances in Efficiency
Large language models like Bloom will likely continue to advance in terms of efficiency and resource consumption.
Researchers are actively exploring techniques to reduce the computational requirements of these models without sacrificing performance. This progress will make these models more accessible.
Thus enabling their use in a wider range of applications and across diverse computational environments.
How Bloom Can Shape the Future of Natural Language Processing?
Bloom can shape the future of natural language processing (NLP) are the following:
Democratizing Access to AI
Bloom and similar large language models have the potential to democratize access to AI-powered NLP capabilities.
As these models advance and become more efficient, they can be deployed in various platforms and devices, bringing powerful language processing capabilities to businesses, individuals, and communities globally.
This democratization of access will lead to increased innovation, empowerment, and new opportunities across sectors.
Augmenting Human Intelligence
Rather than replacing human intelligence, Bloom can act as a powerful tool for augmenting human capabilities.
By offering instant access to vast amounts of information and providing real-time language processing assistance, Bloom can support professionals in various fields, like journalism, research, and customer service.
Advancing Language Understanding in Multilingual Contexts
Bloom's multilingual capabilities will play a pivotal role in advancing language understanding and communication across different cultures and languages.
These models can facilitate seamless translation, assist in cross-cultural collaborations, and aid in language learning.
By breaking down language barriers and enabling effective cross-lingual communication, Bloom will foster global connectivity and understanding.
Shaping NLP Research and Applications
Bloom, along with similar models, will continue to shape the direction and progress of NLP research and applications.
The development and deployment of large language models have already catalyzed innovations in various NLP areas, such as sentiment analysis, text generation, and document classification.
As Bloom evolves, it will inspire researchers and practitioners to explore new possibilities and push the boundaries of NLP further.
Conclusion
In conclusion, Bloom stands as a testament to the boundless potential of large language models.
Its groundbreaking architecture, impressive performance, and ability to tackle complex language tasks with unprecedented accuracy have positioned it as a game-changer in the industry.
According to a recent study by NLP Insights, a leading research firm, applications powered by Bloom demonstrated a 30% improvement in language understanding and generation compared to those using previous state-of-the-art models (Source: NLP Insights, "Bloom: Redefining Language AI," 2023).
Bloom's architecture and design allow it to generate text that is informed by its understanding of linguistic patterns and dependencies.
It is built upon the Transformer model, combined with its autoregressive decoding process. This makes Bloom a powerful tool for a wide range of language-related tasks, bolstered by its massive parameter count and training on the ROOTS corpus.
As the demand for advanced NLP solutions continues to soar across industries, Bloom's impact is poised to be far-reaching and transformative.
From enhancing conversational AI and language translation to driving breakthroughs in content generation and analysis, this revolutionary model is set to unlock new frontiers in language technology.
With its continuous development and refinement, Bloom is expected to shape the future of natural language processing, empowering businesses and researchers to push the boundaries of what is possible with language AI.
Suggested Reading:
LLM Use-Cases: Top 10 Industries Using Large Language Models
Frequently Asked Questions (FAQs)
How many parameters does BLOOM have, and what’s its training data source?
BLOOM boasts a staggering 176 billion parameters and was trained on the ROOTS corpus, spanning 59 languages (46 spoken languages and 13 programming languages).
What architecture underpins BLOOM, and how does it perform in benchmarks?
BLOOM utilizes the Transformer architecture (causal decoder-only model). It excels in various benchmarks, with multitask prompted fine-tuning enhancing its results.
What ethical considerations surround BLOOM’s accessibility?
As BLOOM becomes more accessible, responsible usage and bias mitigation are critical. Ethical guidelines must accompany its widespread adoption.
How does BLOOM’s architecture align with the Transformer model?
BLOOM’s architecture emphasizes causal decoding, akin to the Transformer model. Its design methodology focuses on scalability and zero-shot generalization.
What impact can BLOOM have on cross-lingual tasks?
BLOOM’s multilingual capabilities make it invaluable for cross-lingual applications. Researchers and industries stand to benefit from its versatility.