What is Multi-task Learning?
Multi-task Learning is an approach in machine learning where a model is trained simultaneously on multiple related tasks, using a shared representation.
The idea is that learning tasks in parallel can improve the model’s performance on each task, thanks to the shared information and patterns.
Core Objective
The primary aim of MTL is to improve learning efficiency and prediction accuracy for each task by leveraging the domain-specific information contained in the training signals of related tasks.
The Mechanisms
Underneath the hood, MTL works by interpreting the commonalities and differences across tasks, which guides the model to focus on shared features that are beneficial for multiple tasks.
Applications
From natural language processing (NLP) and computer vision to speech recognition, MTL has found utility in a wide array of domains, enhancing performance and efficiency.
Importance
In the vast data-driven landscape, MTL stands out for its ability to handle complexity, reduce overfitting, and make efficient use of data, proving itself as an invaluable asset in the AI toolkit.
Who Benefits from Multi-task Learning?
Identifying the beneficiaries of MTL illuminates its versatility and wide-reaching impact.
AI Researchers
Those at the frontier of AI and machine learning find MTL invaluable for pushing the boundaries of what's possible, and exploring new methodologies and innovations.
Data Scientists
Professionals working with complex datasets across industries employ MTL to derive enhanced insights and models from their data.
Technology Companies
From startups to tech giants, companies leverage MTL to improve their products and services, whether it's in improving search algorithms, enhancing image recognition features, or refining recommendation systems.
Healthcare Sector
MTL aids in various healthcare applications, from patient diagnosis to treatment recommendations, by integrating multiple data sources and tasks.
Academia
Educators and students alike delve into MTL to explore its theoretical underpinnings and practical applications in research and development projects.
Why is Multi-task Learning Important?
Grasping the essence of MTL's significance sheds light on why it's heralded in the machine learning community.
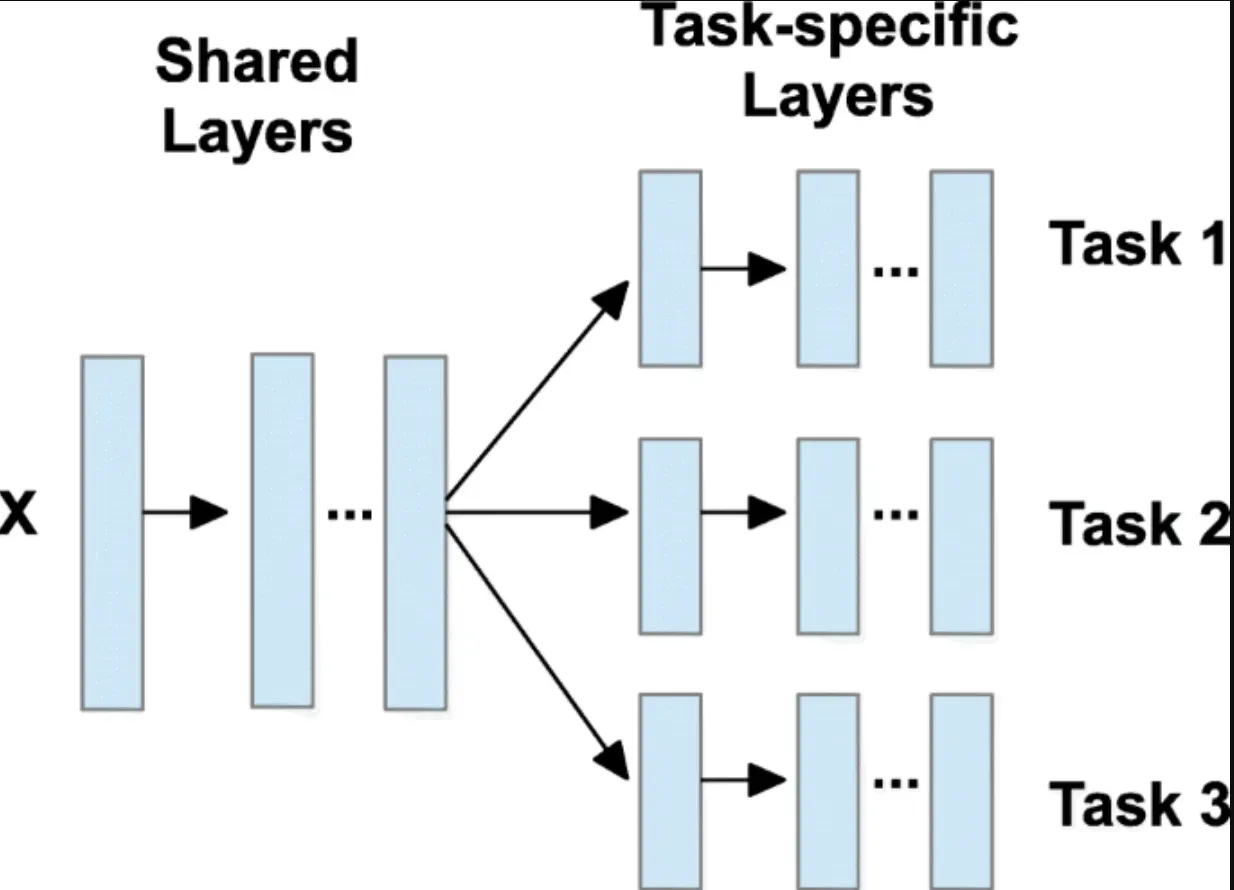
Efficiency in Learning
By sharing representations among related tasks, MTL can significantly reduce the time and resources required for training models.
Improved Generalization
MTL models tend to generalize better on unseen data, thanks to the regularization effect of learning tasks in conjunction.
Enhanced Performance
Leveraging shared knowledge across tasks often leads to improved accuracy and performance on individual tasks.
Flexibility
MTL’s adaptability makes it applicable across a range of fields and challenges, showcasing its utility in handling complex, multifaceted problems.
Data Utilization
In scenarios with limited data, MTL can make the most of available information by sharing relevant features across tasks.
When to Use Multi-task Learning?
Knowing when to implement MTL can elevate its effectiveness and applicability.
Task Relatedness
MTL is most effective when the tasks at hand are related or complementary, allowing the algorithm to capitalize on shared insights.
Small Data Sets
In situations where data for a particular task is scarce, MTL can leverage additional data from related tasks to improve learning outcomes.
Domain Complexity
For complex domains where tasks can benefit from shared understanding, MTL offers a pathway to managing and interpreting such complexity.
Resource Constraints
When computational resources or data collection capabilities are limited, MTL provides a more efficient alternative to single-task learning models.
Model Simplification
When the goal is to streamline the modeling process for multiple tasks, MTL offers a cleaner, more integrated approach.
How Does Multi-task Learning Work?
Delving into the mechanics of MTL reveals the synergy that powers its success.
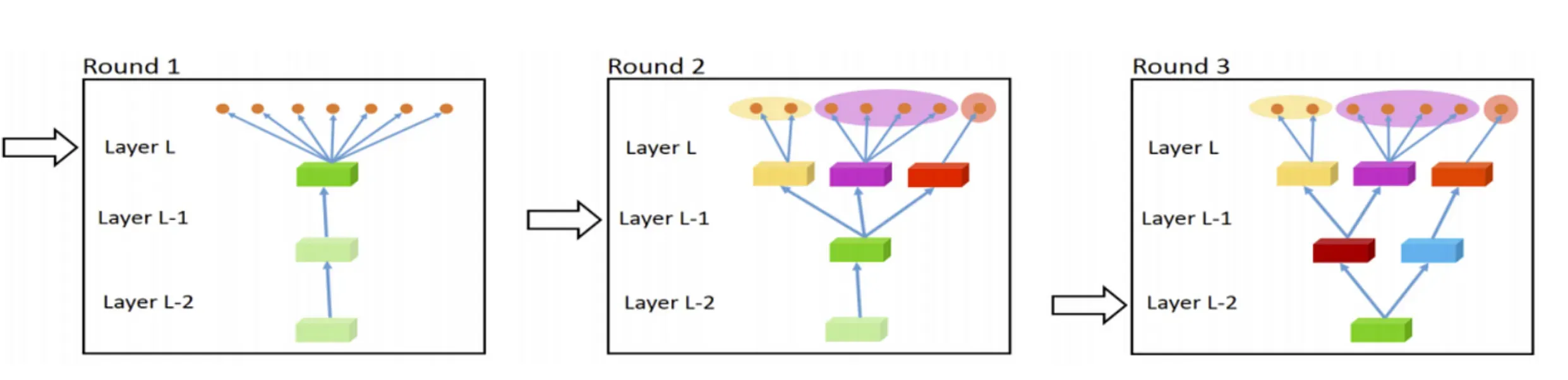
Shared Architecture
The foundational element of MTL is its shared neural network architecture, which allows tasks to learn from each other.
Task-Specific Components
While sharing common features, MTL models also include task-specific layers or parameters to cater to the unique aspects of each task.
Loss Function
A joint loss function that aggregates the losses from each task guides the training process, ensuring balanced learning across tasks.
Regularization Techniques
MTL models often employ regularization techniques to prevent overfitting to any single task and encourage a more generalized model.
Optimization Strategies
Effective MTL involves carefully balancing the influence of each task during training, which can involve sophisticated optimization strategies.
Challenges in Multi-task Learning
Despite its potential, MTL is not without its hurdles and complexities.
Task Interference
Finding the right balance between tasks is crucial, as too much emphasis on one task can lead to interference and degrade overall performance.
Complexity in Design
Designing an MTL model that effectively shares information across tasks while maintaining task-specific capabilities is a challenging feat.
Scalability
As the number of tasks increases, maintaining efficiency and performance in MTL models can become increasingly difficult.
Data Imbalance
Discrepancies in the size and quality of data across tasks can affect learning, necessitating sophisticated balancing techniques.
Evaluation Metrics
Evaluating the performance of MTL models can be complex due to the need to consider multiple tasks and their interactions.
Best Practices in Multi-task Learning
Adhering to best practices can maximize the efficacy and potential of MTL.
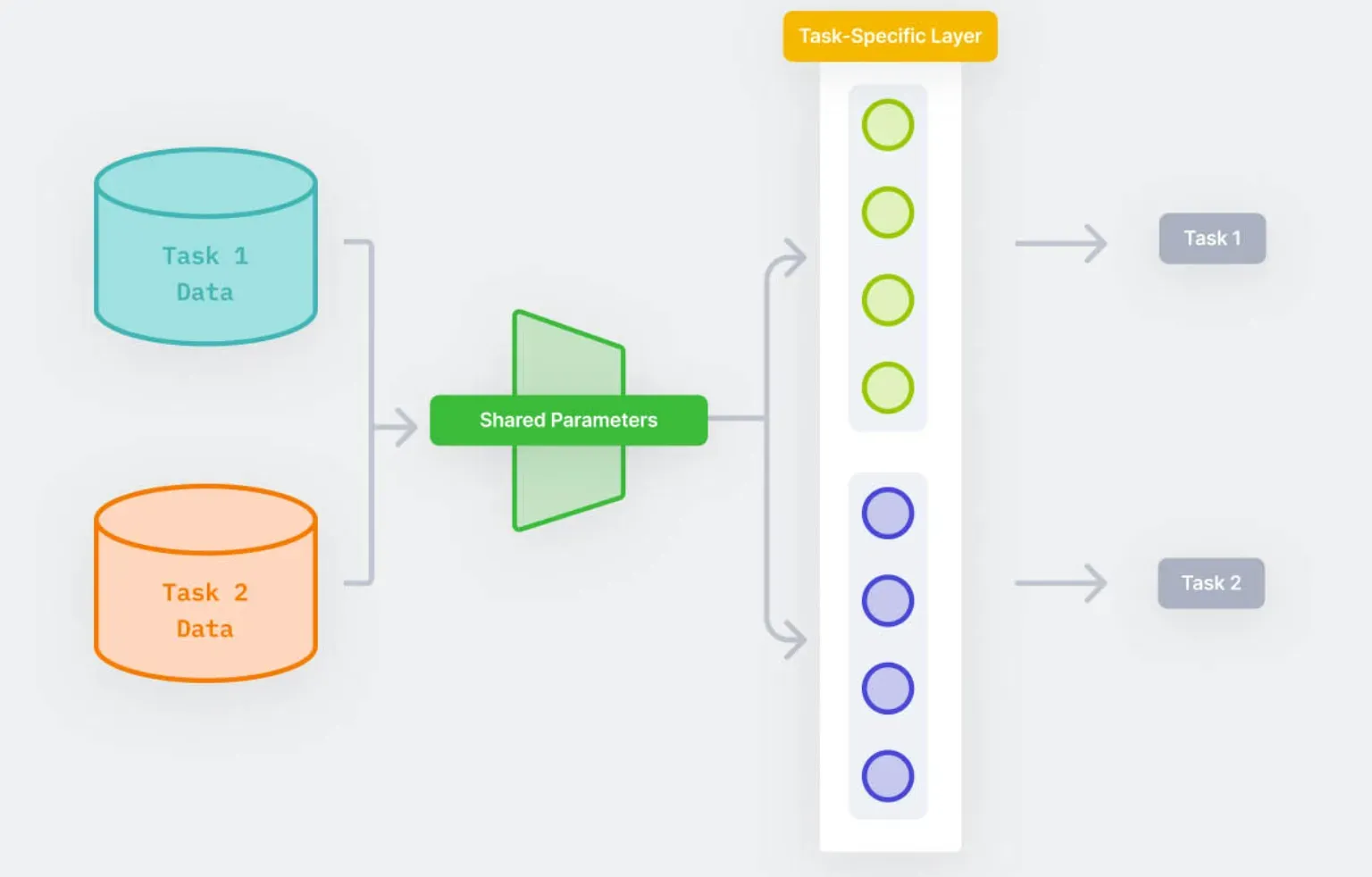
Careful Task Selection
Choose tasks that are related and can benefit from shared learning, ensuring a synergistic relationship.
Balanced Training
Implement strategies to maintain balance in training across tasks, preventing dominance by any single task.
Modular Design
Design MTL models with modularity in mind, allowing for flexible sharing of features and task-specific adjustments.
Continuous Evaluation
Regularly evaluate the model’s performance on all tasks, using a variety of metrics to ensure balanced learning.
Experimentation and Iteration
Experiment with different architectures, loss functions, and optimization strategies to find the best setup for your specific set of tasks.
Future Trends in Multi-task Learning
Looking ahead, the evolution of MTL is set to continue its trajectory toward greater sophistication and broader applicability.
Deep Learning Integration
Further integration with advanced deep learning models and architectures will likely enhance MTL's capabilities and applications.
Cross-domain MTL
Exploration of MTL across vastly different domains could unlock new synergies and insights, broadening its utility.
Automation
Advancements in AutoML (Automated Machine Learning) may lead to more automated approaches to designing and optimizing MTL models.
Scalability Solutions
Continued research is expected to address scalability challenges, making MTL more efficient for larger numbers of tasks.
Enhanced Interpretability
Efforts to improve the interpretability of MTL models will aid in understanding the shared and task-specific features, leading to more insightful models.
Frequently Asked Questions (FAQs)
How does Multi-task Learning improve Model Generalization?
By jointly learning related tasks, multitask learning utilizes shared representations, leading to models that generalize better on unseen data.
Can multi-task learning reduce the need for large datasets?
Yes, multi-task learning can leverage shared information across tasks, reducing the need for large, task-specific datasets for effective learning.
How does Multi-task Learning influence Training Time?
While multi-task learning may initially increase training time due to multiple objectives, it can ultimately result in more efficient models that solve several tasks simultaneously.
What determines the choice of tasks in Multi-task Learning?
Tasks are chosen based on their relevance and the potential to share useful information, improving the performance across all tasks involved.
Can Multitask Learning be applied across different Data Modalities?
Yes, multitask learning is flexible across modalities, allowing for tasks involving various types of data (text, images, etc.) to be learned together.