What if machines could learn? Imagine algorithms so advanced that they can analyze data and find patterns on their own without explicit programming. These self-learning algorithms are transforming businesses today.
Machine learning is revolutionizing industries from online retail to transportation. By examining large datasets, machine learning development models can unlock game-changing insights to solve complex problems. The applications are endless - from predicting customer behavior to optimizing supply chains.
This blog post explores the immense potential of machine learning for business growth. We'll cover real-world examples of machine learning development in action, from Netflix's personalized recommendations to Uber's dynamic pricing.
You'll discover best practices for leveraging machine learning and practical tips to avoid common pitfalls. Whether you're an executive seeking a competitive edge or a budding data scientist, this blog has something for you.
By the end, you'll be itching to harness ML to take your business to the next level! Sound enticing? Read on...
Benefits of Machine Learning Development for Business
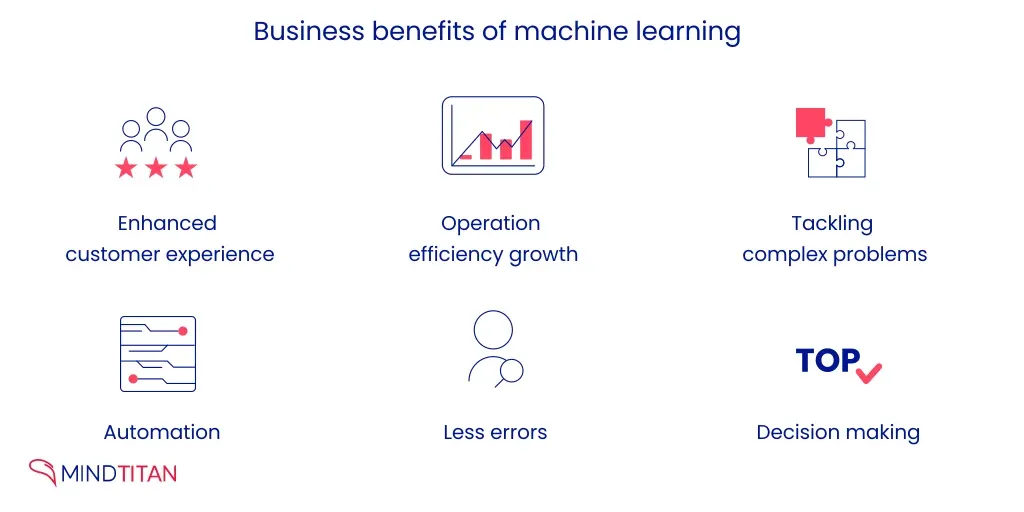
Machine learning development applications have changed the way businesses operate in a data-driven world. Using machine learning development, businesses can unlock several benefits that enhance their decision-making processes, improve the customer experience, increase operational efficiency, and enable better planning through predictive analytics.
Improved Decision-Making Processes
Machine learning development application algorithms can analyze vast amounts of data quickly and accurately, providing valuable insights for effective decision-making.
By identifying patterns, trends, and correlations within the data, businesses can make informed decisions based on data-driven insights rather than relying solely on intuition or guesswork.
Enhanced Customer Experience
With machine learning, businesses can personalize customer experiences to meet individual preferences and needs.
By analyzing customer behavior, preferences, and purchase history, businesses can create targeted marketing campaigns, recommend relevant products or services, and provide personalized customer support.
This level of personalization enhances customer satisfaction and loyalty.
Now, if you want to begin with machine learning development to enhance your customer experience but have no clue about how to do it, then check out the NO-CODE chatbot platform, named BotPenguin.
With all the heavy work of chatbot development already done for you, BotPenguin allows users to integrate some of the prominent language models like GPT 4, Google PaLM, and Anthropic Claude to create chatbots that are powered by machine learning for platforms like:
- WhatsApp Chatbot
- Facebook Chatbot
- WordPress Chatbot
- Telegram Chatbot
- Website Chatbot
- Squarespace Chatbot
- woocommerce Chatbot
- Instagram Chatbot
Increased Operational Efficiency
Machine learning development applications can optimize various business processes, automating repetitive tasks and streamlining operations. This can increase efficiency and productivity while reducing errors and minimizing costs.
Machine learning algorithms can analyze large datasets to identify bottlenecks, optimize workflows, and automate routine tasks, freeing up employees' time to focus on higher-value activities.
Predictive Analytics for Better Planning
Machine learning development application enables businesses to leverage predictive analytics and make accurate forecasts based on historical data.
By using advanced algorithms, businesses can predict customer behavior, market trends, demand patterns, and potential risks.
This allows businesses to make proactive decisions, optimize resource allocation, and develop effective strategies to stay ahead in a competitive marketplace.
Next, we will see the process of Machine Learning Development.
Process of Machine Learning Development
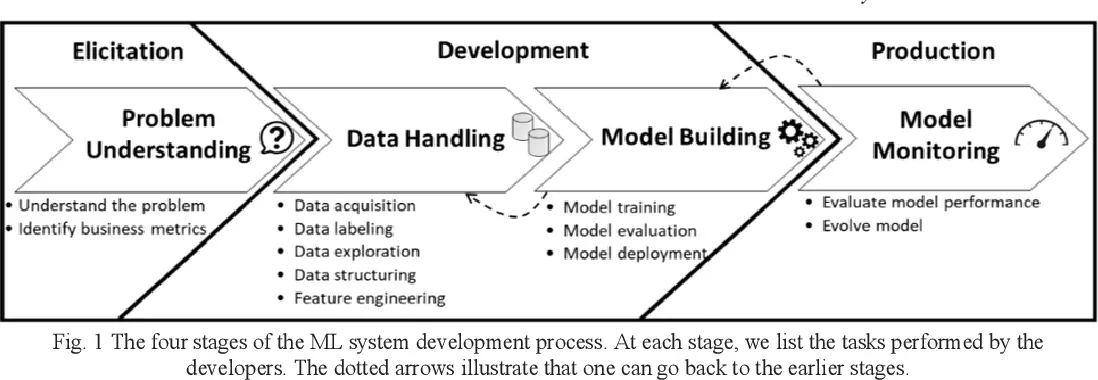
To successfully implement machine learning development applications, businesses need to follow a structured process that encompasses various steps.
Here is a breakdown of the key stages involved:
Identifying Business Problems and Goals
The first step is to identify the specific business problems or goals that machine learning development applications can address.
This involves understanding the challenges faced by the business, defining clear objectives, and determining how machine learning can provide a solution or improvement.
Data Collection and Preparation
Good quality and relevant data are essential for accurate machine-learning models.
Businesses need to collect and prepare the data by cleaning, organizing, and transforming it into a suitable format for processing.
This involves removing outliers, handling missing values, and ensuring data consistency.
Suggested Reading:
Why Machine Learning Development is Pivotal for Your Business?
Choosing the Appropriate Machine Learning Algorithm
Different machine learning development application algorithms are suited for different types of problems.
Businesses need to select the most appropriate algorithm based on the nature of the problem, available data, and desired outcomes.
This step requires an understanding of the strengths and limitations of different algorithms.
Feature Engineering and Model Training
Feature engineering involves selecting the relevant features or variables from the dataset to build the machine learning development application.
Businesses need to carefully engineer these features to enhance the performance and accuracy of the model.
Once the features are defined, the model is trained using the prepared data.
Model Evaluation and Refinement
After training the model, businesses must evaluate its performance to ensure its accuracy and effectiveness.
This involves testing the model with new data and assessing its predictive capabilities. Based on the evaluation results, businesses can refine and optimize the model to improve its performance.
Through this systematic process, businesses can use machine learning development applications to solve complex problems, extract meaningful insights, and drive informed decision-making.
By understanding the benefits and following the development process, businesses can harness the power of machine learning to thrive in today's competitive business landscape.
Next, we will see challenges and considerations in Machine Learning Development.
Challenges and Considerations in Machine Learning Development
Implementing machine learning development can bring a multitude of benefits to businesses.
However, it is essential to know the challenges and considerations that can arise throughout the process.
From data quality and availability to the interpretability of machine learning models, businesses need to navigate these obstacles to ensure successful implementation.
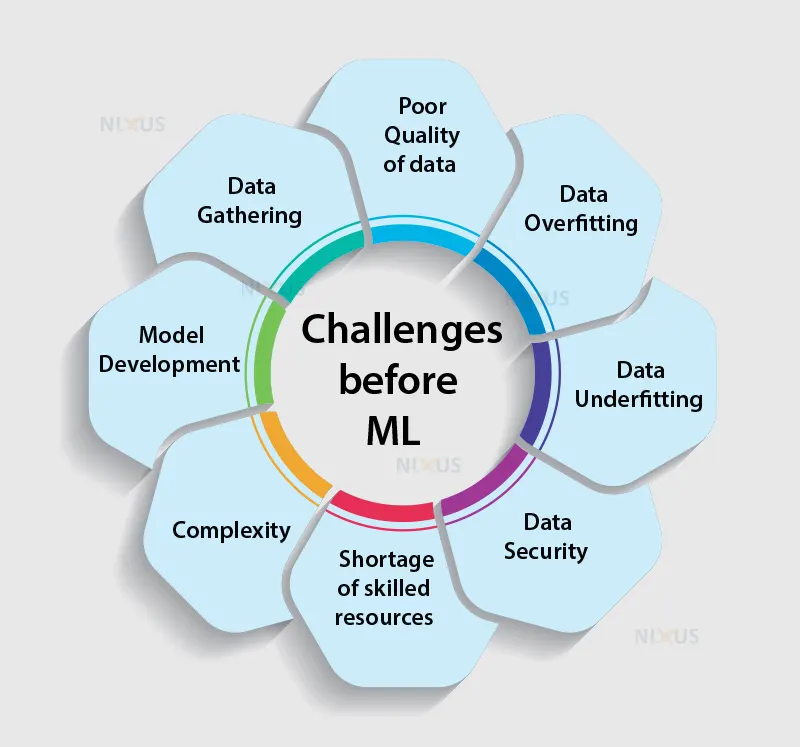
Data Quality and Availability
One of the primary challenges in machine learning development is the quality and availability of data.
Machine learning development application algorithms rely heavily on large volumes of high-quality data for accurate and reliable results. Inadequate or incomplete data can lead to biased or misleading outcomes.
Therefore, businesses must ensure that their data collection processes are robust, the data is cleaned and preprocessed effectively, and they have access to a sufficient amount of representative data for training their models.
Bias and Ethical Considerations
Bias in machine learning algorithms has gained significant attention in recent years.
Biased data or algorithmic biases can lead to unfair outcomes or perpetuate existing biases, such as discrimination or unequal treatment.
Therefore, businesses need to be diligent in addressing bias during the development process. This includes considering the diversity and representativeness of their data, implementing fairness measures, and regularly evaluating and mitigating bias in their models.
Ethical considerations are also crucial when it comes to machine learning development application. Businesses must ensure that their use of machine learning aligns with regulatory requirements and ethical standards.
They should consider potential unintended consequences, such as privacy concerns, security vulnerabilities, or automated decision-making that may have a significant impact on individuals.
Transparency and accountability in using machine learning development application technologies are key aspects to be mindful of.
Interpretability of Machine Learning Models
Machine learning models, such as deep neural networks, can be complex and difficult to interpret, sometimes called "black-box" models.
This lack of interpretability can be a challenge, especially in industries where decisions need to be explained or justified.
When applying machine learning development application in such domains, it's important for businesses to consider the trade-off between model complexity and interpretability.
Employing techniques like feature importance analysis or using inherently interpretable models can assist in addressing this challenge.
Scalability and Implementation Issues
Scalability is an important consideration for businesses looking to implement machine learning at scale.
Developing and training models may require substantial computational resources, and as datasets grow, maintaining efficient and scalable systems becomes crucial.
Additionally, integrating machine learning models into existing workflows and systems can present implementation challenges.
Businesses must consider the infrastructure requirements, deployment strategies, and potential integration issues to ensure smooth adoption and utilization of machine learning solutions.
While these challenges may seem daunting, with careful planning, thoughtful consideration, and the right expertise, businesses can overcome them in their machine learning development endeavors.
It is important to approach these challenges as opportunities for growth and improvement. By addressing data quality, bias, interpretability, and scalability, businesses can harness the power of machine learning and unlock its full potential to drive innovation and success.
Next, we will cover best practices and tips for businesses.
Suggested Reading:
Best Practices and Tips for Businesses
When it comes to machine learning development, businesses can follow certain best practices and tips to ensure successful implementation and maximize the benefits they can derive from it.
From starting with a clear problem statement to continuously evaluating and refining models, here are some key practices to consider.
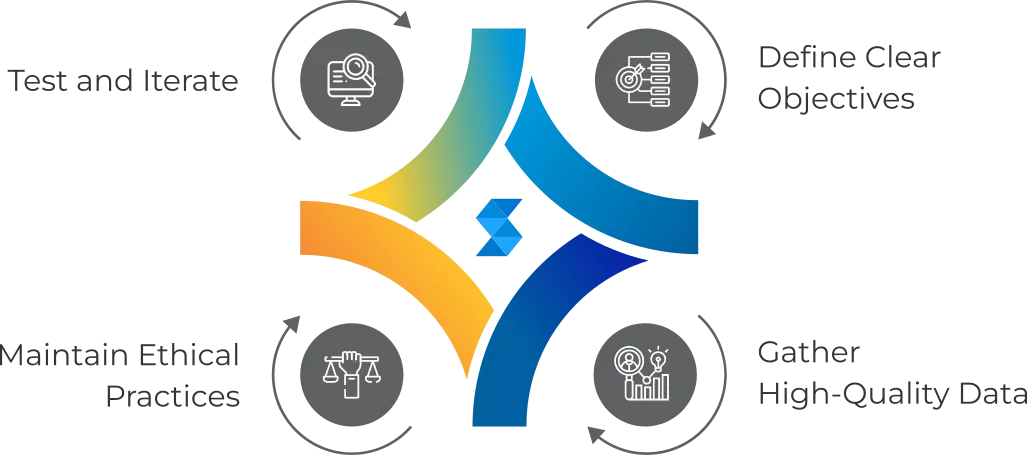
Start with a Clear Problem Statement
Before diving into machine learning development, it is crucial to start with a clear problem statement.
Clearly define the problem you aim to solve or the goal you want to achieve with machine learning.
This helps in setting the right direction, aligning efforts, and avoiding unnecessary time and resource waste.
Involve Domain Experts and Stakeholders
Involving domain experts and stakeholders throughout the machine learning development process is essential for success.
Domain experts possess valuable insights and knowledge about the problem domain and can contribute to feature engineering, model selection, and evaluation.
Stakeholders, on the other hand, ensure that the machine learning development application aligns with business objectives and requirements.
Ensure Data Quality and Transparency
Data quality is critical for accurate and reliable machine learning models. Ensure that the data used for training and testing is clean, representative, and free from bias.
Implement data validation and preprocessing techniques to handle missing values, outliers, and inconsistencies.
Additionally, promote transparency by documenting data sources, preprocessing steps, and any assumptions made during the development process.
Continuously Evaluate and Refine Models
Machine learning development application are not static; they require continuous evaluation and refinement.
Regularly assess the performance of your models using appropriate evaluation metrics. Monitor key metrics such as accuracy, precision, recall, or mean squared error and compare them against your defined success criteria.
Use this feedback to refine your models, incorporate new data, and improve their performance.
Keep Up with Advancements in Machine Learning
Machine learning rapidly evolves, with new techniques and algorithms being developed regularly.
To stay competitive and harness the full potential of machine learning, businesses need to keep up with these advancements.
Stay informed about the latest research, attend conferences, and invest in continuous learning and development for your machine learning teams.
Last but not least, let us cover some real-world examples of businesses leveraging machine learning.
Real-world Examples of Businesses Leveraging Machine Learning
Machine learning development applications have become prevalent in various industries, transforming how businesses operate and deliver value to their customers.
Here are a few real-world examples of businesses leveraging machine learning to enhance their offerings.
Amazon's Personalized Recommendations
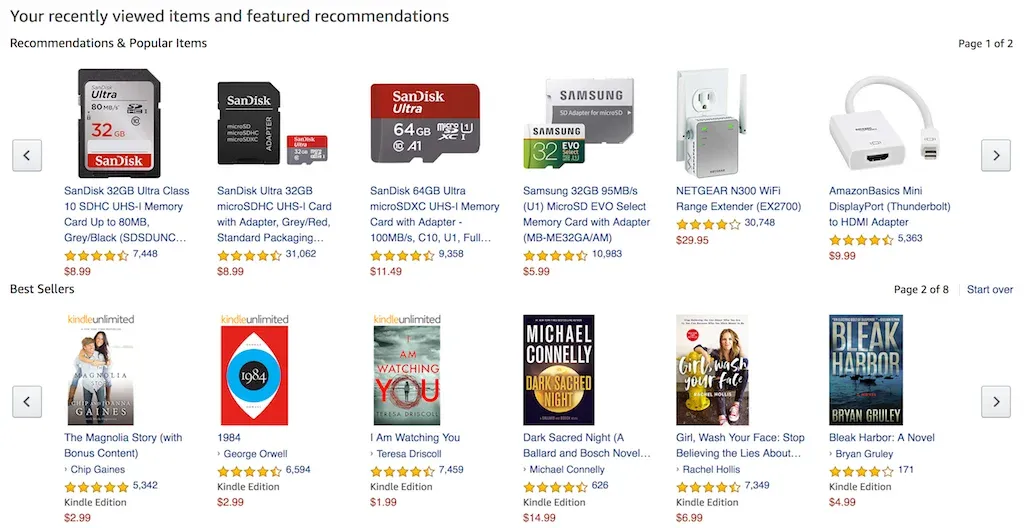
Amazon's recommendation system is powered by machine learning algorithms that analyze customer behavior, purchase history, and product attributes.
By understanding individual preferences, Amazon provides personalized recommendations to its customers, increasing customer satisfaction and sales.
Netflix's Movie and Show Suggestions
Netflix uses machine learning algorithms to recommend personalized movies and show suggestions to its subscribers.
By analyzing viewing history, preferences, and ratings, Netflix delivers content tailored to individual tastes, enhancing the user experience and increasing engagement.
Uber's Dynamic Pricing
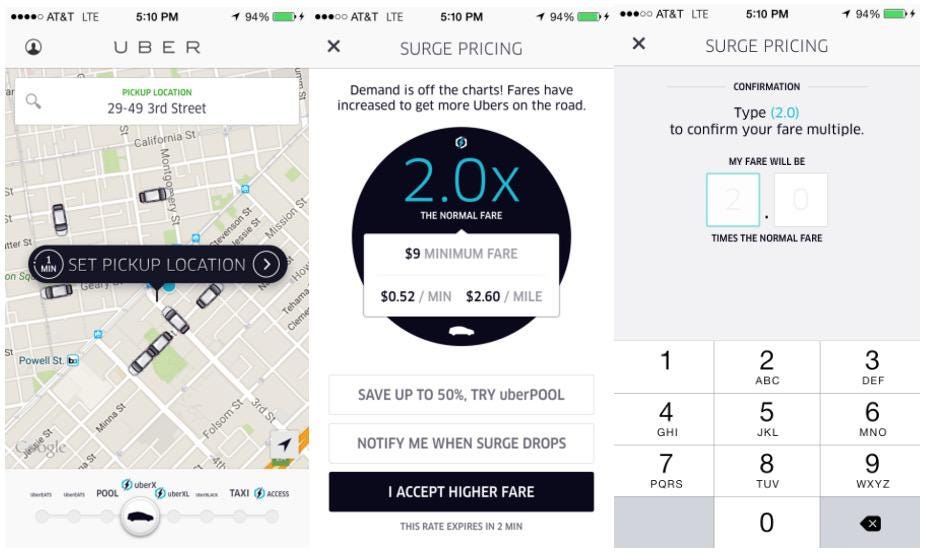
Uber uses machine learning to determine dynamic pricing based on supply and demand, traffic conditions, and time of day.
By optimizing pricing in real-time, Uber can balance rider demand and driver supply, leading to efficient matching and improved service availability.
Spotify's Customized Playlists
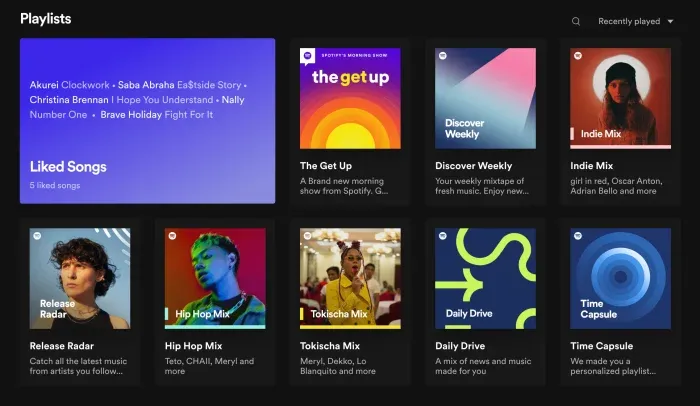
Spotify uses machine learning development application to create customized playlists for its users.
By analyzing listening patterns, music preferences, and user behavior, Spotify's algorithms generate playlists tailored to individual tastes, providing a personalized music streaming experience.
These examples illustrate how machine learning can be leveraged to enhance customer experiences, optimize operations, and drive business growth.
By adopting best practices and learning from real-world use cases, businesses can embark on their own machine-learning journey and unlock the endless possibilities it offers.
Conclusion
The potential of machine learning is astounding - from optimizing complex supply chains to providing personalized recommendations. As this technology continues advancing rapidly, virtually every industry stands to benefit.
However, realizing the full promise of machine learning requires thoughtful strategy and execution. By following the best practices outlined here, you can set your organization up for success. Start with clear business objectives, invest in quality data, and ensure transparency.
Don't let the challenges intimidate you - with the right expertise and continuous refinement, machine learning can transform how you operate. Study the real-world examples to uncover new opportunities in your domain.
The future is here. Will you lead with the power of machine learning or lag behind? The choice is clear. Take the first step today towards leveraging ML and unlocking sustainable competitive advantage for your business. The possibilities are endless.
Suggested Reading:
What Are the Applications of Machine Learning in Different Sectors
Frequently Asked Questions (FAQs)
How can small businesses with limited resources leverage machine learning development?
Small businesses can leverage pre-built machine learning development applications, collaborate with cloud services, and focus on specific use cases for cost-effective implementation and benefits.
In what ways does machine learning contribute to customer relationship management (CRM) for businesses?
Machine learning in CRM optimizes customer interactions by personalizing communication, predicting customer preferences, and improving overall customer satisfaction and loyalty.
Can machine learning development help businesses improve their supply chain management?
Yes, machine learning development applications optimize supply chain management by forecasting demand, enhancing inventory management, and improving overall business logistics efficiency.
What role does machine learning play in enhancing marketing strategies for businesses?
Machine learning development applications improve marketing strategies through personalized campaigns, customer behavior analysis, and targeted advertisements, boosting overall marketing effectiveness and return on investment.
How can businesses ensure the ethical use of machine learning in their operations?
Ensuring ethical use involves transparent algorithms, responsible data practices, and adherence to regulatory guidelines, promoting trust and responsible AI implementation.
What types of data are crucial for successful machine learning development in businesses?
High-quality and diverse data, including historical records, customer feedback, and relevant industry data, are crucial for training accurate and effective machine learning models in businesses.
Are there industry-specific considerations when implementing machine learning in businesses?
Yes, industries may have unique challenges and opportunities, necessitating a tailored approach to machine learning implementation based on specific business requirements and goals.
How can businesses measure the ROI of their machine learning development initiatives?
Measuring ROI involves tracking key performance indicators, assessing improvements in efficiency or revenue, and comparing the costs of implementation, providing a comprehensive evaluation of the impact of machine learning development initiatives.