What is Predictive Analysis?
Predictive Analysis is the use of statistical algorithms and machine learning techniques to analyze historical data and make predictions about future events. It involves identifying patterns and trends in data to make informed decisions and improve business outcomes.
Types of Predictive Analysis
There are several types of Predictive Analysis, including regression analysis, decision trees, neural networks, and time series analysis.
Techniques Used in Predictive Analysis
Some of the key techniques used in Predictive Analysis include data mining, data visualization, and predictive modeling.
Why is Predictive Analysis Important?
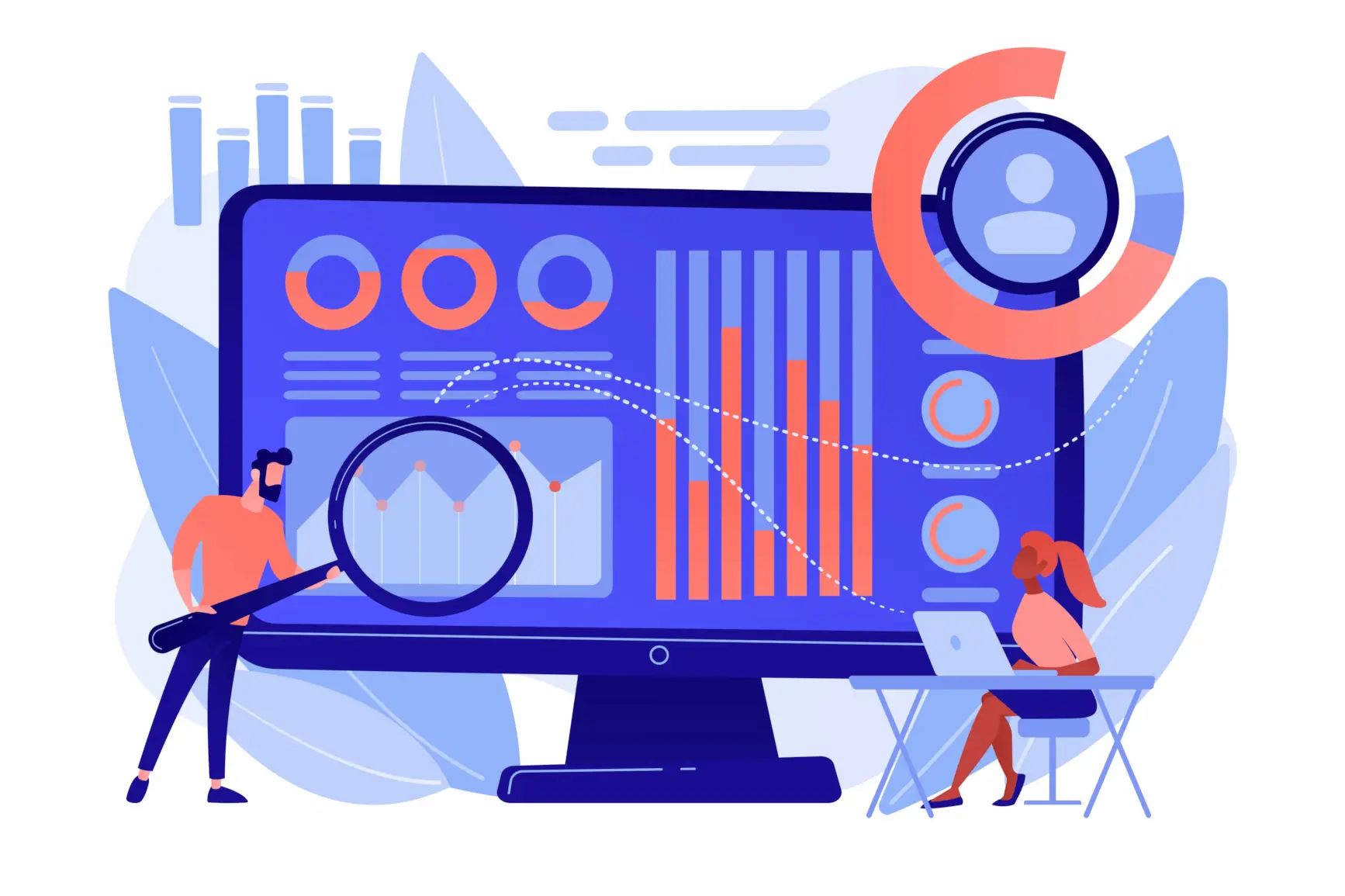
Informed Decision-Making
Predictive analysis provides valuable insights and forecasts, allowing businesses and individuals to make data-driven decisions with greater confidence.
Resource Optimization
By predicting future trends and demands, organizations can allocate resources more effectively, improving efficiency and reducing costs.
Risk Management
Predictive analysis helps identify potential risks and vulnerabilities, enabling proactive measures to mitigate or prevent adverse outcomes.
Customer Experience Enhancement
Understanding customer behavior and preferences through predictive analysis enables businesses to tailor their offerings and improve customer satisfaction and loyalty.
Competitive Advantage
Leveraging predictive analysis can provide a competitive edge by identifying opportunities, anticipating market changes, and staying ahead of industry trends.
Who Uses Predictive Analysis?
Retail and E-commerce
Retailers and e-commerce businesses use predictive analysis to forecast demand, optimize pricing, and personalize customer experiences.
Healthcare
Healthcare organizations leverage predictive analysis for patient risk assessment, early disease detection, and resource allocation.
Finance and Banking
Financial institutions use predictive analysis for credit scoring, fraud detection, and investment strategy optimization.
Manufacturing
Manufacturers apply predictive analysis to optimize production processes, manage inventory, and predict equipment maintenance needs.
Marketing and Sales
Marketers and sales teams utilize predictive analysis to identify potential customers, improve targeting, and enhance customer relationship management.
When to Use Predictive Analysis?
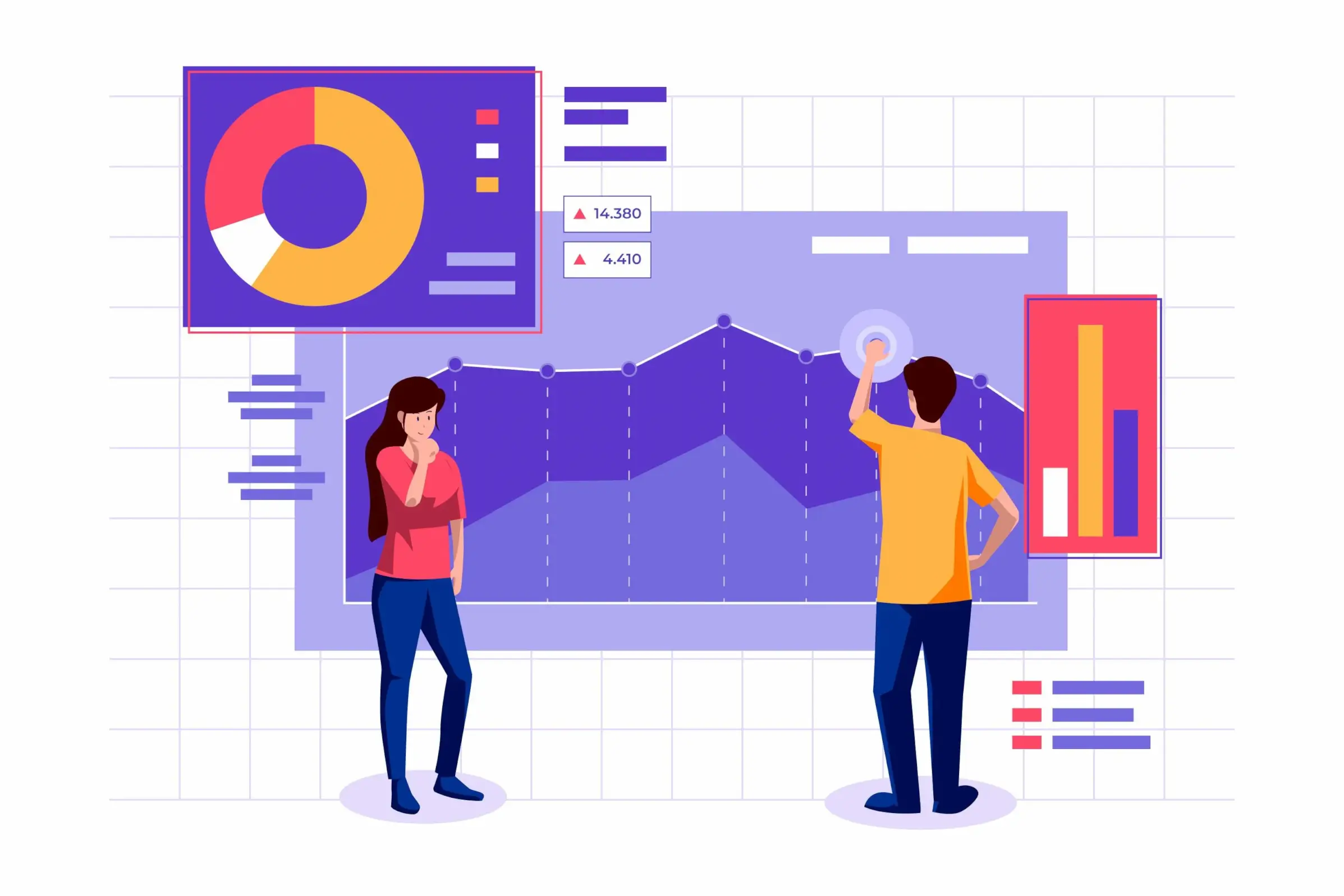
Predictive Analysis can be used in a variety of situations where data-driven insights are required. Some of the key use cases for Predictive Analysis include risk management, demand forecasting, and fraud detection.
Impact of Predictive Analysis on Decision-Making
Predictive Analysis can have a significant impact on decision-making by providing valuable insights into customer behavior, market trends, and business performance.
Predictive Analysis for Future Planning
Predictive Analysis can also be used for future planning by identifying potential risks and opportunities and developing strategies to mitigate or capitalize on them.
How Does Predictive Analysis Work?
Predictive Analysis involves several key steps, including data collection and preparation, model building, and evaluation and deployment.
Data Collection and Preparation for Predictive Analysis
Data collection and preparation involves identifying relevant data sources, cleaning and formatting the data, and selecting the appropriate variables for analysis.
Techniques Used in Predictive Analysis
Techniques used in Predictive Analysis include data mining, machine learning, and statistical analysis.
Data Sources for Predictive Analysis
Data sources for Predictive Analysis include structured and unstructured data from a variety of sources, including customer data, social media, and IoT devices.
Importance of Data Quality in Predictive Analysis
Data quality is essential for accurate Predictive Analysis, as inaccurate or incomplete data can lead to incorrect predictions and poor decision-making.
Challenges in Data Collection for Predictive Analysis
Challenges in data collection for Predictive Analysis include data privacy concerns, data fragmentation, and data integration issues.
Predictive Modeling
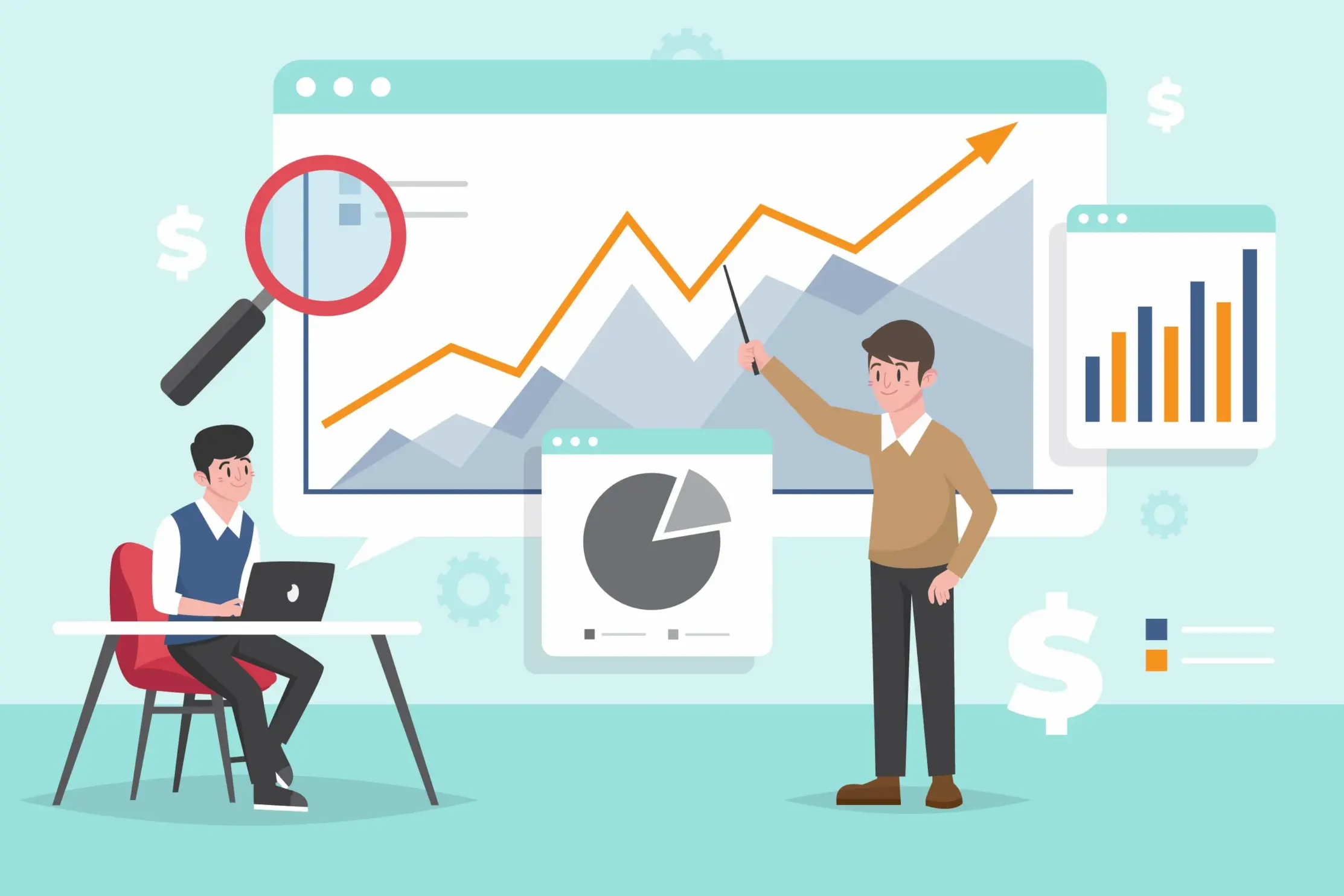
Data Collection and Preparation
Gather relevant data from various sources, clean and preprocess it to ensure quality, and format it for use in predictive modeling.
Feature Selection and Engineering
Identify the most relevant features for prediction, and create or transform features to enhance the predictive power of the model.
Model Selection
Choose a suitable machine learning algorithm or predictive modeling technique based on the problem, data, and desired outcomes.
Model Training and Tuning
Train the selected model using the prepared data, and fine-tune its parameters to achieve optimal performance and avoid overfitting or underfitting.
Model Evaluation and Deployment
Evaluate the model's performance using appropriate metrics, and deploy it in a real-world setting to generate predictions and inform decision-making.
Machine Learning in Predictive Analysis
Machine Learning enhances predictive analysis by using algorithms to analyze data and make accurate predictions based on patterns and trends.
Supervised Learning
Supervised learning algorithms use labeled data to train models, enabling them to make predictions based on known input-output relationships in predictive analysis.
Unsupervised Learning
Unsupervised learning algorithms identify patterns and relationships within unlabeled data, helping to uncover hidden insights and improve predictive analysis.
Feature Engineering
Machine learning techniques can be used to create new features or transform existing ones, enhancing the predictive power of the analysis.
Model Evaluation and Validation
Machine learning provides methods for evaluating model performance, such as cross-validation and performance metrics, ensuring reliable predictive analysis.
Ensemble Methods
Ensemble methods combine multiple machine learning models to improve predictive accuracy and reduce the risk of overfitting or underfitting.
Challenges in Predictive Analysis
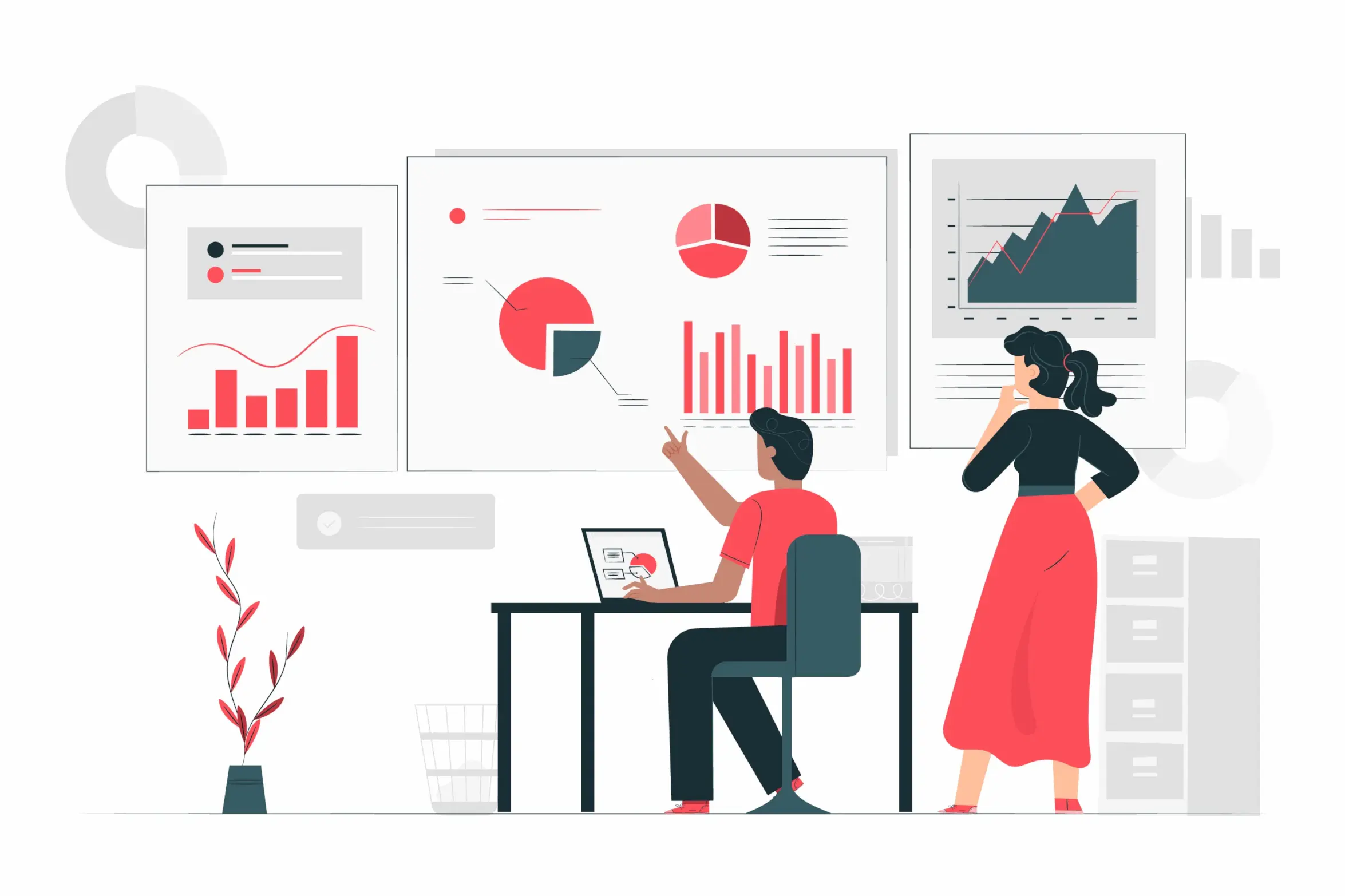
Data Quality and Preprocessing
Ensuring data quality and handling missing or inconsistent data are critical challenges in predictive analysis, as they directly impact model accuracy.
Feature Selection
Identifying the most relevant features for prediction can be difficult and time-consuming, but it's essential for building effective predictive models.
Model Selection and Tuning
Choosing the right algorithm and fine-tuning its parameters can be complex, requiring expertise and experimentation to achieve optimal results.
Overfitting and Underfitting
Balancing model complexity to avoid overfitting (too specific) or underfitting (too general) is crucial for accurate and reliable predictions.
Real-time Predictions
Implementing predictive analysis in real-time scenarios can be challenging due to computational constraints and the need for continuous model updates.
TL;DR
Predictive Analysis is a powerful tool that can help businesses make data-driven decisions, improve operational efficiency, and gain a competitive edge. By understanding the basics of Predictive Analysis and addressing the challenges involved, businesses can leverage this technology to achieve their desired outcomes and stay ahead in today's fast-paced digital landscape.
Frequently Asked Questions
What is predictive analysis?
Predictive analysis uses historical data, statistical algorithms, and machine learning techniques to forecast future events, trends, or behaviors based on patterns in the data.
How does predictive analysis work?
Predictive analysis involves collecting data, preprocessing it, selecting relevant features, building predictive models, and evaluating their performance to make accurate future predictions.
What are the benefits of predictive analysis?
Predictive analysis helps businesses make informed decisions, optimize processes, identify opportunities, mitigate risks, and improve overall efficiency and effectiveness.
In which industries is predictive analysis used?
Predictive analysis is widely used in industries such as finance, healthcare, retail, manufacturing, marketing, and transportation for various decision-making purposes.
What are some common predictive analysis techniques?
Common predictive analysis techniques include regression analysis, time series forecasting, decision trees, neural networks, and clustering algorithms.