Machine learning development is rapidly evolving. New trends emerge constantly, shaping the future of AI technologies.
From advancements in deep learning to the integration of natural language processing, the landscape is dynamic. As data volumes soar, algorithms become more sophisticated..
With massive improvements in compute capabilities, availability of data and algorithms, machine learning is driving innovation and growth.
Machine learning has become integral to building intelligent systems and software applications across sectors. Its ability to handle large volumes of data and adapt to changing environments makes it a powerful tool for solving complex problems and driving innovation in numerous fields.
So what’s the next trend of machine learning?
Continue reading, as this article explores the latest trends shaping machine learning development and offers predictions for the near future.
What is Machine Learning?
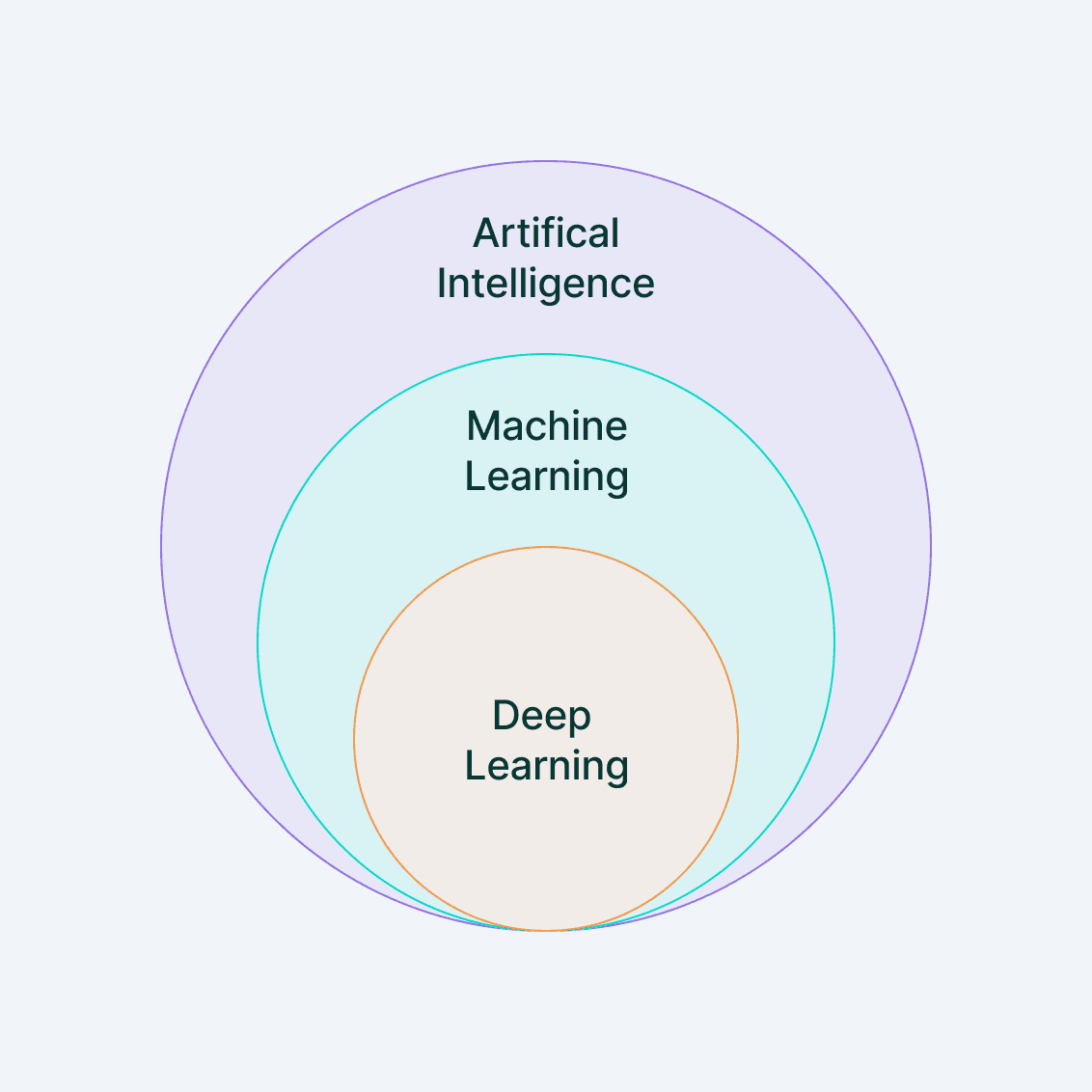
Machine learning is a branch of artificial intelligence (AI) that focuses on enabling computers to learn from data and improve their performance over time without being explicitly programmed. It involves algorithms that analyze and interpret large sets of data to identify patterns, make predictions, or provide insights.
There are several types of machine learning approaches, including supervised learning, unsupervised learning, and reinforcement learning. In supervised learning, algorithms are trained on labeled data, where the correct answers are provided, to learn the relationship between inputs and outputs. Unsupervised learning involves finding patterns and structures within unlabeled data. Reinforcement learning teaches machines to make decisions by trial and error, learning from feedback in the form of rewards or penalties.
Machine learning is widely used in various applications such as image and speech recognition, natural language processing, recommendation systems, medical diagnosis, financial forecasting, and autonomous vehicles.
Current Trends in Machine Learning Development
Machine learning is rapidly evolving, and understanding the current trends is vital. In this section, we will explore the three major trends in machine learning: deep learning, natural language processing (NLP), and reinforcement learning.
Deep Learning
Deep learning is a subset of machine learning that mimics the human brain's neural networks. It has gained immense significance in machine learning development due to its ability to process vast amounts of complex data. Deep learning has propelled advancements in speech recognition, image recognition, and natural language understanding.
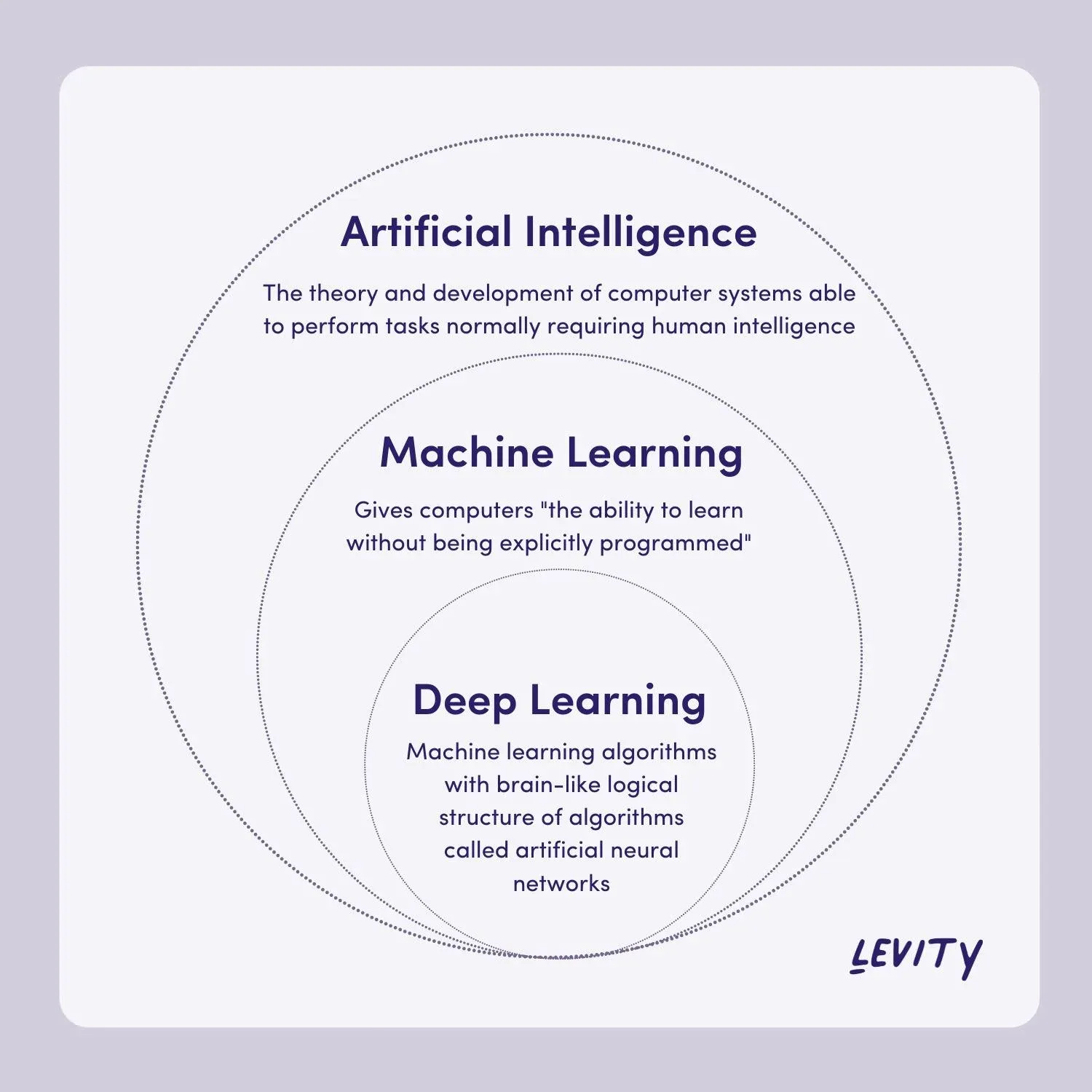
The impact of deep learning can be seen in various successful applications. For instance, it has revolutionized the field of computer vision, enabling accurate object detection and image classification. Deep learning models have also demonstrated exceptional performance in language translation and sentiment analysis applications.
However, deep learning faces challenges and limitations. It requires large amounts of labeled data for training, which can be time-consuming and costly. Additionally, deep learning models can be black boxes, making their interpretability and explainability a challenge. These limitations need to be addressed to fully harness the potential of deep learning in machine learning development.
Suggested Reading:
Why Machine Learning Development is Pivotal for Your Business?
Natural Language Processing (NLP)
NLP is a branch of artificial intelligence that focuses on the interaction between computers and human language. It plays a crucial role in machine learning development by enabling machines to understand and process human language.
In various industries, NLP has found real-life applications that bring significant value. For instance, in healthcare, NLP is used to extract important information from medical records and aid in diagnosis.
In customer service, NLP powers virtual assistants and chatbots, enhancing customer interactions. Furthermore, NLP has revolutionized sentiment analysis in social media monitoring and sentiment-based marketing.
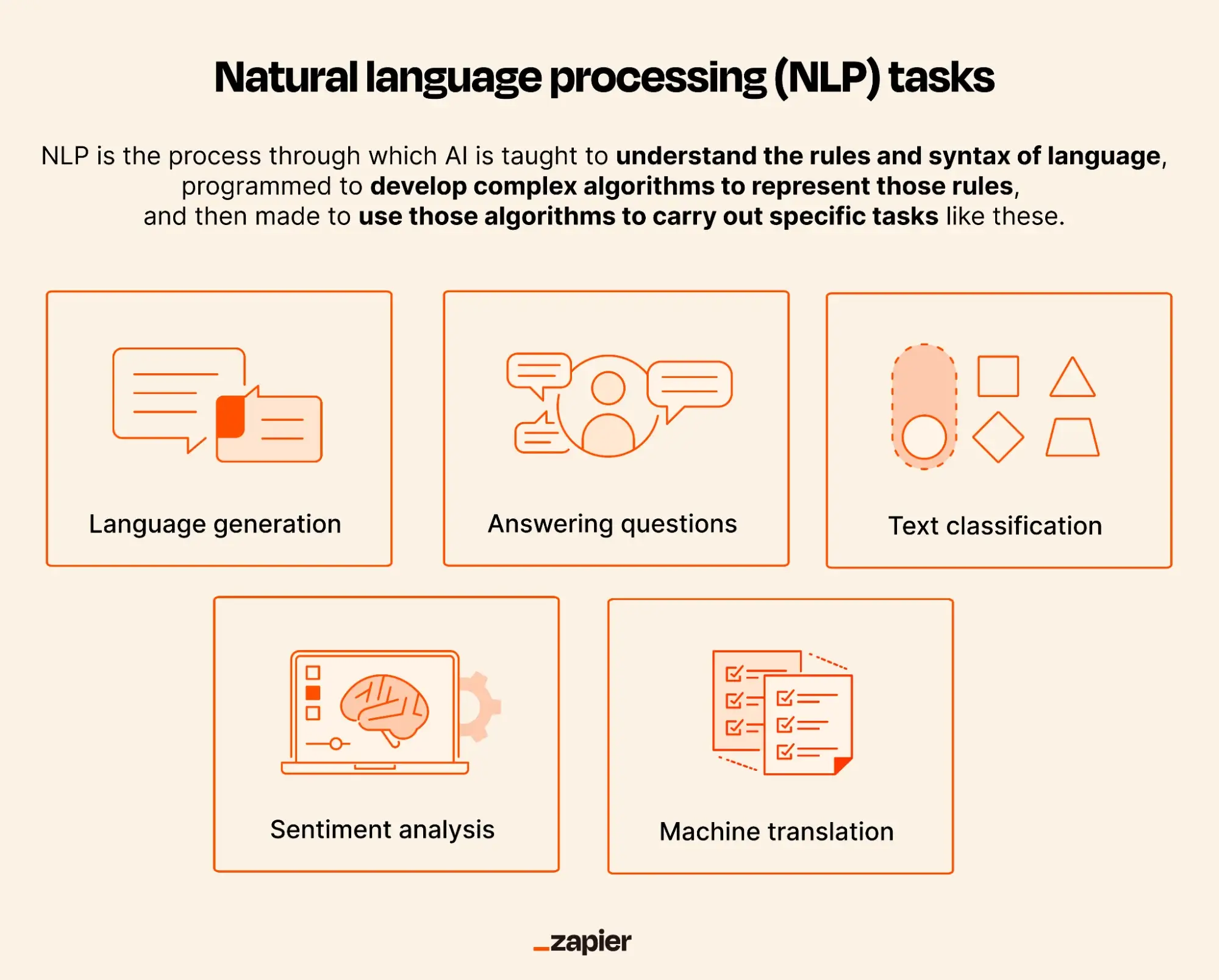
The future of NLP holds immense potential. Advancements in NLP models, such as transformers, have led to significant improvements in language understanding and generation. As technology progresses, we can anticipate more accurate language translation, speech recognition, and even more sophisticated chatbots.
Now, if you want to begin with NLP to enhance your customer service but have no clue about how to do it, then check out the NO-CODE chatbot platform, named BotPenguin.
With all the heavy work of chatbot development already done for you, BotPenguin lets you easily train your chatbot on custom data, paint them with your logo and branding, and offer human-like conversational support to your customers.
And that's not it!
BotPenguin allows users to integrate some of the prominent NLP models like GPT 4, Google PaLM, and Anthropic Claude to create AI-powered chatbots for platforms like:
- WhatsApp Chatbot
- Facebook Chatbot
- WordPress Chatbot
- Telegram Chatbot
- Website Chatbot
- Squarespace Chatbot
- woocommerce Chatbot
- Instagram Chatbot
Reinforcement Learning
Reinforcement learning is a type of machine learning where an agent learns to interact with an environment to maximize rewards. It takes inspiration from behavioral psychology and has become essential in solving complex decision-making problems.
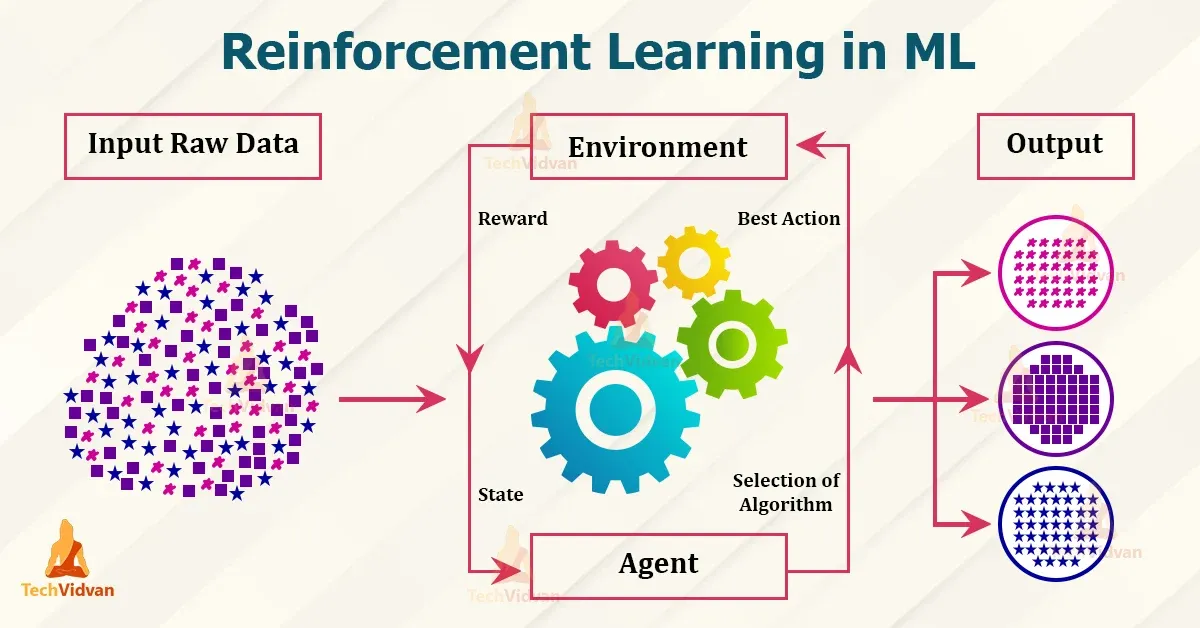
Successful applications of reinforcement learning span various domains. In robotics, reinforcement learning has enabled robots to learn tasks through trial and error, leading to advancements in areas like autonomous driving and industrial automation. Game-playing algorithms, such as AlphaGo, have also demonstrated the capabilities of reinforcement learning in outperforming human players.
Looking to the future, reinforcement learning holds immense promise. However, challenges such as sample inefficiency, scalability, and safety need to be addressed. As researchers work towards overcoming these challenges, reinforcement learning has the potential to revolutionize fields like healthcare, finance, and resource management.
Key Predictions for Future Machine Learning Development
As machine learning continues to advance, the future of this technology holds numerous promising predictions. In this section, we will explore three key predictions for future machine learning development.
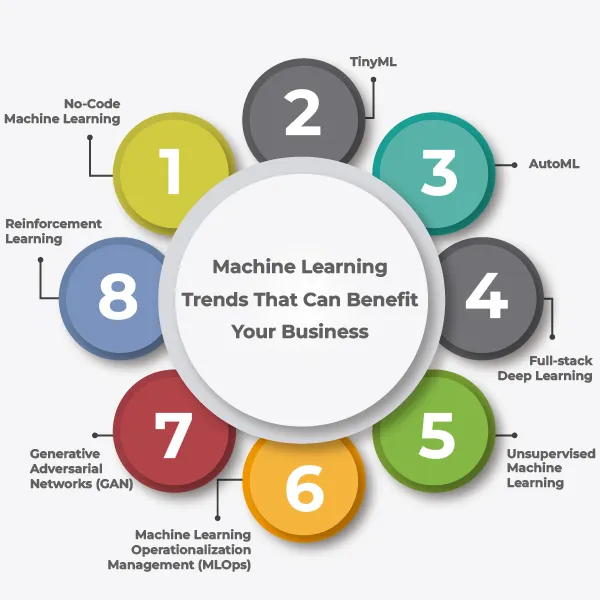
Advanced Automation and Optimization
The future of machine learning development is expected to witness increased automation and optimization. As algorithms and tools become more sophisticated, tasks that previously required manual intervention will be automated, leading to significant time and cost savings. Additionally, optimization techniques will enable more efficient and accurate model training and hyperparameter tuning.
While advanced automation brings several benefits, such as increased productivity and scalability, there are potential drawbacks to consider. Overreliance on automation may lead to a reduction in human involvement and decision-making, which could impact transparency and accountability. Moreover, the job market may undergo significant changes as certain roles become automated, necessitating the acquisition of new skills.
Enhanced Interpretability and Explainability
The need for better interpretability and explainability in machine learning models is expected to grow in the future. As algorithms become increasingly complex, understanding and explaining their decision-making processes become paramount, particularly in critical domains like healthcare and finance. Interpretability enables stakeholders to trust, validate, and comprehend the outputs of machine learning models.
However, achieving enhanced interpretability poses challenges. Deep learning models, for example, often lack interpretability, appearing as black boxes. Researchers are exploring methods like model distillation and rule extraction to address this issue. Emphasizing the interpretability of machine learning models is crucial to maintaining trust and accountability while leveraging the possibilities of this technology.
Suggested Reading:
What Are the Application of Machine Learning in Different Sectors
Ethical and Responsible Machine Learning
The rise of ethical and responsible machine learning practices is a significant prediction for the future. As machine learning applications become more pervasive, concerns regarding privacy, bias, fairness, and discrimination arise. Adhering to ethical principles and ensuring responsible practices will be essential to mitigate risks and protect individuals' rights.
Discussions on ethical considerations related to machine learning development are vital. The adoption of frameworks and guidelines, like fairness and transparency by design, can help prevent biased decision-making. Incorporating diverse perspectives during the development process can also prevent the reinforcement of existing biases. Furthermore, promoting transparency in machine learning systems, including clear explanations of data sources and decision processes, fosters accountability and responsible usage.
Conclusion
In conclusion, machine learning development is experiencing rapid advancements. Deep learning, NLP, and reinforcement learning are three significant trends in machine learning development. These trends have revolutionized various industries and opened up new possibilities for solving complex problems
The future of machine learning development is marked by advanced automation and optimization, enhanced interpretability and explainability, and the rise of ethical and responsible practices. Embracing these predictions will ensure continued progress while addressing challenges such as accountability, fairness, and transparency.
As we navigate these trends and predictions, it is important to strike a balance between leveraging the power of machine learning and addressing challenges such as transparency, fairness, and accountability.
By doing so, we can maximize the potential of machine learning while ensuring its responsible and ethical implementation.
Suggested Reading:
Machine Learning vs Deep Learning vs AI: Which has the most potential?
Frequently Asked Questions (FAQs)
How is natural language processing evolving in machine learning development?
Natural language processing is evolving with transformer models like GPT-4, enabling machines to understand and generate human-like text. This trend is vital for applications such as chatbots, language translation, and sentiment analysis.
What role does edge computing play in machine learning development?
Edge computing in machine learning allows processing to occur closer to the data source, reducing latency and enhancing real-time decision-making. This trend is crucial for applications in IoT devices, autonomous vehicles, and other edge-based scenarios.
What impact does machine learning have on cybersecurity development?
Machine learning contributes significantly to cybersecurity by detecting anomalies, identifying threats, and enhancing overall security measures. This trend is essential as cyber threats continue to evolve, requiring advanced and adaptive defense mechanisms.
What are the emerging applications of machine learning in healthcare?
Machine learning is revolutionizing healthcare with applications such as disease diagnosis, personalized treatment plans, and drug discovery. This trend holds promise for improving patient outcomes, reducing costs, and advancing medical research.
How is machine learning contributing to the development of autonomous systems?
Machine learning is crucial for the development of autonomous systems, enabling vehicles, drones, and robots to navigate and make decisions independently. This trend is driving advancements in industries like transportation, logistics, and manufacturing.
How is the integration of machine learning and the Internet of Things (IoT) evolving?
The integration of machine learning and IoT is evolving to create intelligent, data-driven ecosystems. This trend enhances the capabilities of IoT devices, enabling them to analyze and respond to data in real-time, leading to more efficient and smart applications.