What is Word Sense Disambiguation?
Word Sense Disambiguation (WSD) refers to the process of accurately determining the correct meaning of a word when it has multiple possible meanings or senses.
In both human language understanding and natural language processing (NLP) tasks, WSD plays an important role. Ambiguity arises when words carry multiple meanings, which can lead to confusion or misinterpretation.
WSD uses various techniques, such as supervised learning, unsupervised learning, knowledge-based, and hybrid approaches, to resolve these ambiguities.
Why is WSD (Word Sense Disambiguation) needed?
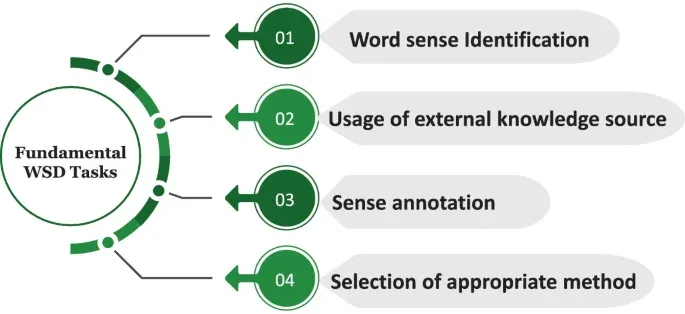
Enhancing Natural Language Understanding
Word Sense Disambiguation (WSD) improves machines' ability to comprehend human language, resulting in better interaction and understanding between humans and AI systems.
Boosting Language Processing Efficiency
Efficient language processing relies on precise meaning interpretation. WSD helps computers quickly determine the correct contextual meaning, enhancing the effectiveness of language processing tasks.
Uplifting User Experience
In AI-powered applications, WSD drives enhanced user experience by leveraging correct meanings, providing more accurate results and relevant content, thus meeting user expectations.
Reducing Ambiguity in Communication
Communication often faces ambiguity challenges, especially in writing. WSD helps identify the intended meaning, reducing ambiguity, and fostering clearer communication between parties.
Fine-Tuning AI-based Solutions
WSD refines various AI-based solutions, like language translation tools or voice assistants, ensuring the delivery of more reliable, context-aware responses, thus yielding higher user satisfaction.
How does WSD work?
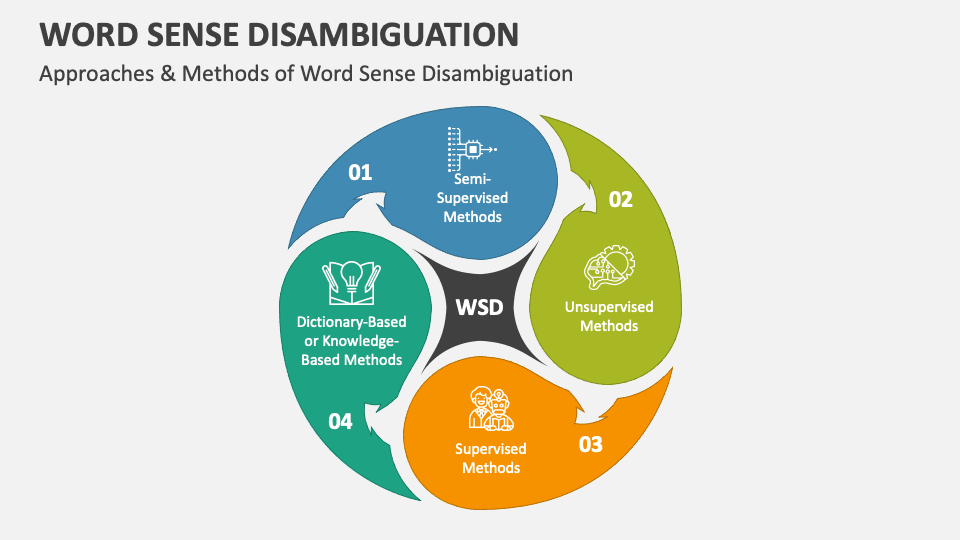
WSD employs various techniques to determine the correct sense of a word in a given context. These techniques can be classified into three main categories: supervised, unsupervised, and knowledge-based.
Supervised WSD
Supervised WSD involves training models on labeled data. Common techniques used in supervised WSD include Decision Trees, Naïve Bayes, Maximum Entropy, and Support Vector Machines (SVMs).
Unsupervised WSD
Unsupervised WSD techniques focus on clustering similar contexts together and assigning senses based on statistical patterns. Examples of unsupervised techniques include Clustering, Topic Modelling, Neural Networks, and Cosine Similarity.
Knowledge-Based WSD
Knowledge-based WSD relies on external resources, such as machine-readable dictionaries, thesauri, and wordnets. It uses the semantic relationships and connections between words to disambiguate their senses.
Hybrid WSD
Hybrid WSD approaches combine multiple techniques, leveraging the best of both supervised and knowledge-based methods. These approaches often incorporate external knowledge with supervised or unsupervised approaches, leading to improved disambiguation accuracy.
Applications of WSD (Word Sense Disambiguation)
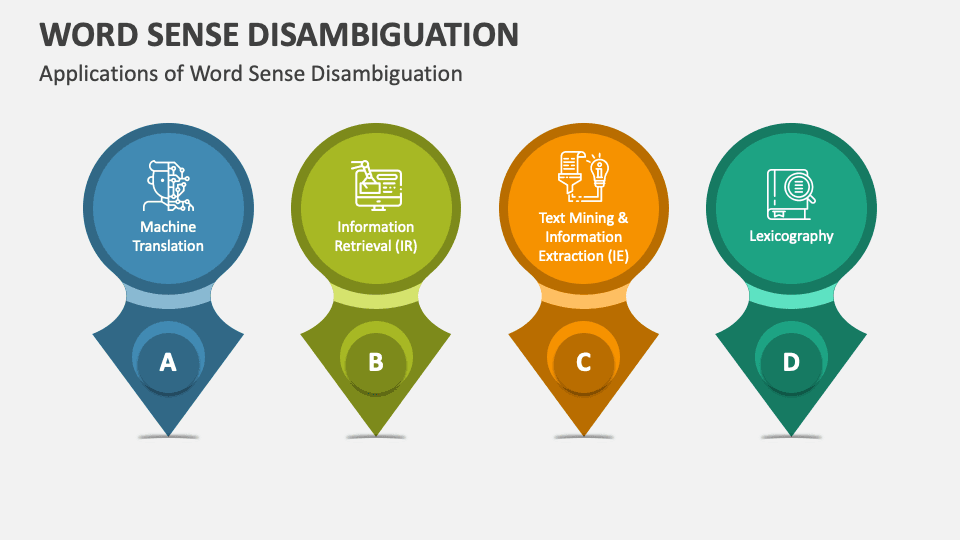
Improving Machine Translation
Word Sense Disambiguation (WSD) plays a pivotal role in enhancing machine translation (MT). By accurately identifying the correct word senses in context, WSD can significantly improve the translation quality, ensuring better understanding and communication.
Enhancing Information Retrieval
WSD can refine information retrieval systems, such as search engines, by basing results on the precise contextual meaning of the text. Accurate disambiguation leads to more relevant search results, increasing the reliability and usefulness of the search system.
Boosting Sentiment Analysis
Sentiment analysis benefits from WSD when interpreting nuanced text, such as reviews and social media posts. By determining the correct sense of a word, WSD enables analysis algorithms to comprehend sarcasm, humor, or double-meanings, providing a more accurate evaluation of user sentiments.
Optimizing Text Classification
Text classification, a fundamental task in Natural Language Processing (NLP), can effectively utilize WSD to categorize documents based on contextually accurate meanings, thus avoiding misinterpretations and yielding more accurate classifications.
Adding Value to Text Summarization
Text summarization, which condenses lengthy documents into shorter versions without losing key information, benefits from WSD when processing ambiguous words or phrases. WSD enables the algorithm to maintain proper semantics, thereby creating cohesive and meaningful summaries.
Challenges in WSD
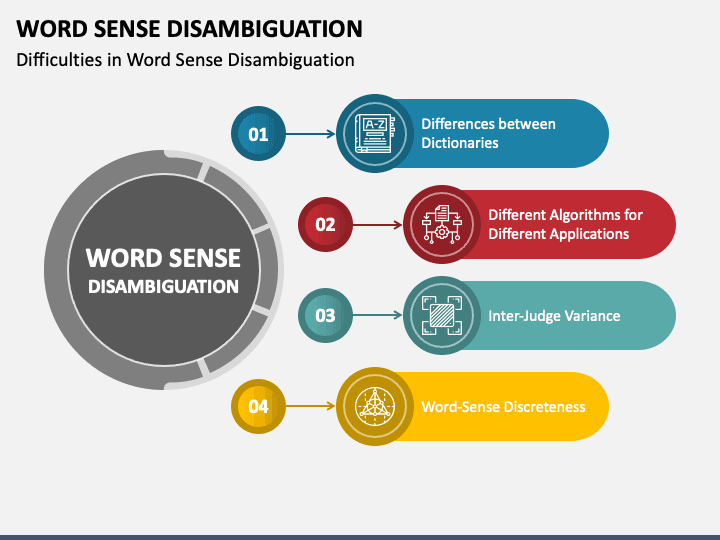
While WSD has proven to be useful, it still faces challenges that researchers and developers are actively working to overcome:
Polysemy and Homonymy
Words with multiple meanings (polysemy) and words that sound alike but have different meanings (homonymy) pose challenges to accurate disambiguation.
Domain Adaptation
WSD models may struggle when applied to different domains due to varying word usages and contexts.
Cross-lingual WSD
Extending WSD to different languages presents additional complexities, such as language-specific sense distinctions.
Low-resource languages
Limited resources and data availability for low-resource languages make accurate WSD more challenging.
Future of WSD
As technology advances, the future of WSD looks promising. Here are a few exciting developments to watch out for:
Advancements in Machine Learning
Improvements in machine learning algorithms and techniques will lead to more accurate and efficient WSD systems.
Integration with other NLP tasks
WSD will continue to integrate with other natural language processing tasks, unlocking new possibilities for enhanced language understanding.
Potential impact on various industries
The accurate disambiguation of words will have a significant impact on industries like healthcare, finance, customer support, and many others.
Frequently Asked Questions (FAQs)
What is Word Sense Disambiguation?
Word Sense Disambiguation (WSD) is the process of identifying the correct meaning of a word in context, when the word has multiple meanings.
Why is Word Sense Disambiguation important?
WSD is crucial for accurate language processing and understanding, as it helps computers interpret human language more effectively.
How is Word Sense Disambiguation used in NLP?
In Natural Language Processing (NLP), WSD aids in text analysis, summarization, translation, and other applications requiring semantic understanding.
Are there specific algorithms for Word Sense Disambiguation?
Yes, various algorithms like supervised, unsupervised, and knowledge-based methods are used to perform WSD.
What are the challenges in Word Sense Disambiguation?
Some challenges include homographs, polysemy, lack of labeled data, and the distinction between senses that are closely related.