What is Content-Based Filtering?
Content-based filtering is a recommendation system algorithm that suggests items similar to what a user has liked before, based on the content of the items themselves.
Mechanism of Action
This technique analyzes a set of descriptors for each item, then constructs a profile of the user's preferences based on the content of items they have interacted with previously.
Usage Context
Commonly used in environments like streaming services, e-commerce, and news aggregators, it helps to personalize user experience according to individual tastes.
Comparison with Other Systems
Unlike collaborative filtering which relies on the wisdom of crowds, content-based filtering strictly sticks to a user's past behavior and the attributes of the content.
Strengths and Weaknesses
The strength lies in its ability to offer personalized recommendations independent of the user community, while its weakness is not capturing the serendipity of discovery beyond content traits.
Who Relies on Content-Based Filtering?
Zooming in on who uses content-based filtering reveals its widespread utility.
Streaming Services
Streaming giants like Netflix harness content-based algorithms to recommend movies and shows similar to what you've watched.
E-Commerce Retailers
Online stores like Amazon use content-based recommendation systems to suggest products based on shoppers' previous browsing and purchasing history.
News Outlets and Aggregators
News platforms like Flipboard offer personalized reading material by learning from the articles you interact with.
Online Advertisements
Ad platforms use content-based filtering to display ads relevant to the content you're already engaging with.
Job and Dating Platforms
Sites like LinkedIn and OKCupid utilize content-based systems to recommend jobs and potential matches by aligning your profile with listings and user attributes.
When is Content-Based Filtering Most Effective?
Delving into the timing of content-based filtering applications can help in understanding its effectiveness.
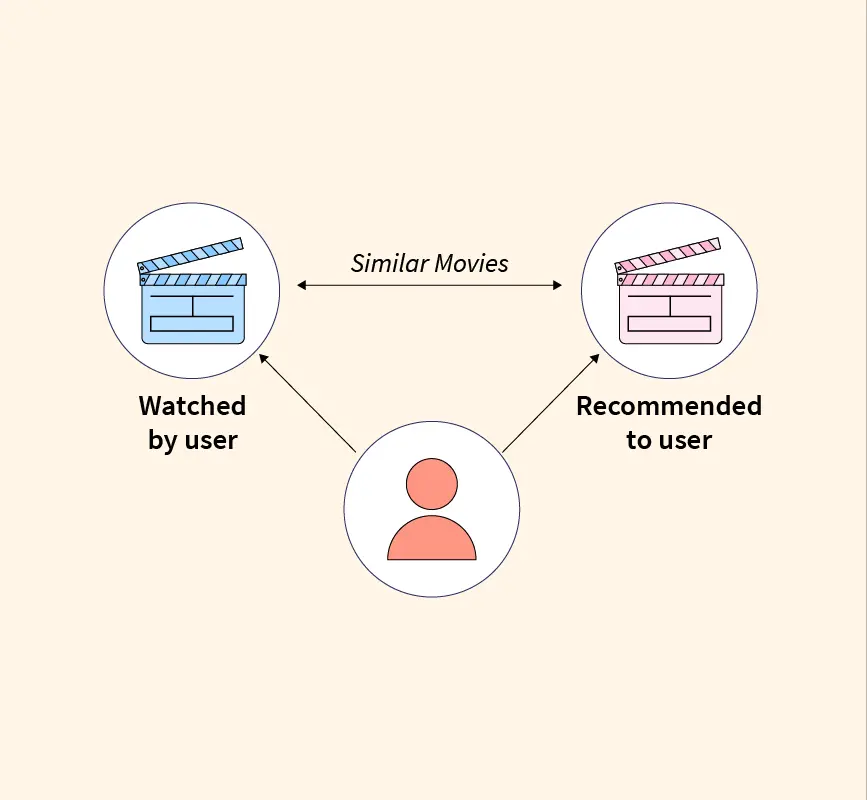
Upon Establishing User Preferences
After a user has interacted with enough content to establish a clear preference profile, content-based filtering shines.
In Niche Markets
For items with specific and detailed attributes, like books or music, content-based systems can be particularly adept at finding the perfect recommendations.
For New Items
When new content is added to a platform, content-based filtering can quickly integrate these items into recommendations based on their attributes.
With Sparse Data
In situations with limited user interaction data, content-based filtering still works well as it doesn't require user-user comparison.
Personal Privacy Focus
When user privacy concerns are paramount, content-based filtering requires no data on other users, thereby better preserving individual privacy.
Where is Content-Based Filtering Applied?
The application sites of content-based filtering are as varied as the digital landscape itself.
Personalized Website Content
Websites employ content-based filtering to personalize your viewing experience based on your browsing behavior.
Music Recommendation Services
Services like Spotify use content-based algorithms to create playlists featuring music with similar qualities to tracks you've liked.
Online Bookstores
Book recommendation systems often rely on analyzing the characteristics of books you enjoy, such as genre or author.
Movie and Video Platforms
Movie services advocate films and series to watch by understanding the genres, directors, actors, and plot descriptions you prefer.
Educational Resources
Online educational platforms may recommend courses or materials by matching your previous learning patterns with content attributes.
How is Content-Based Filtering Implemented?
Rolling up our sleeves, let's dig into the nitty-gritty of how content-based filtering is put into practice.
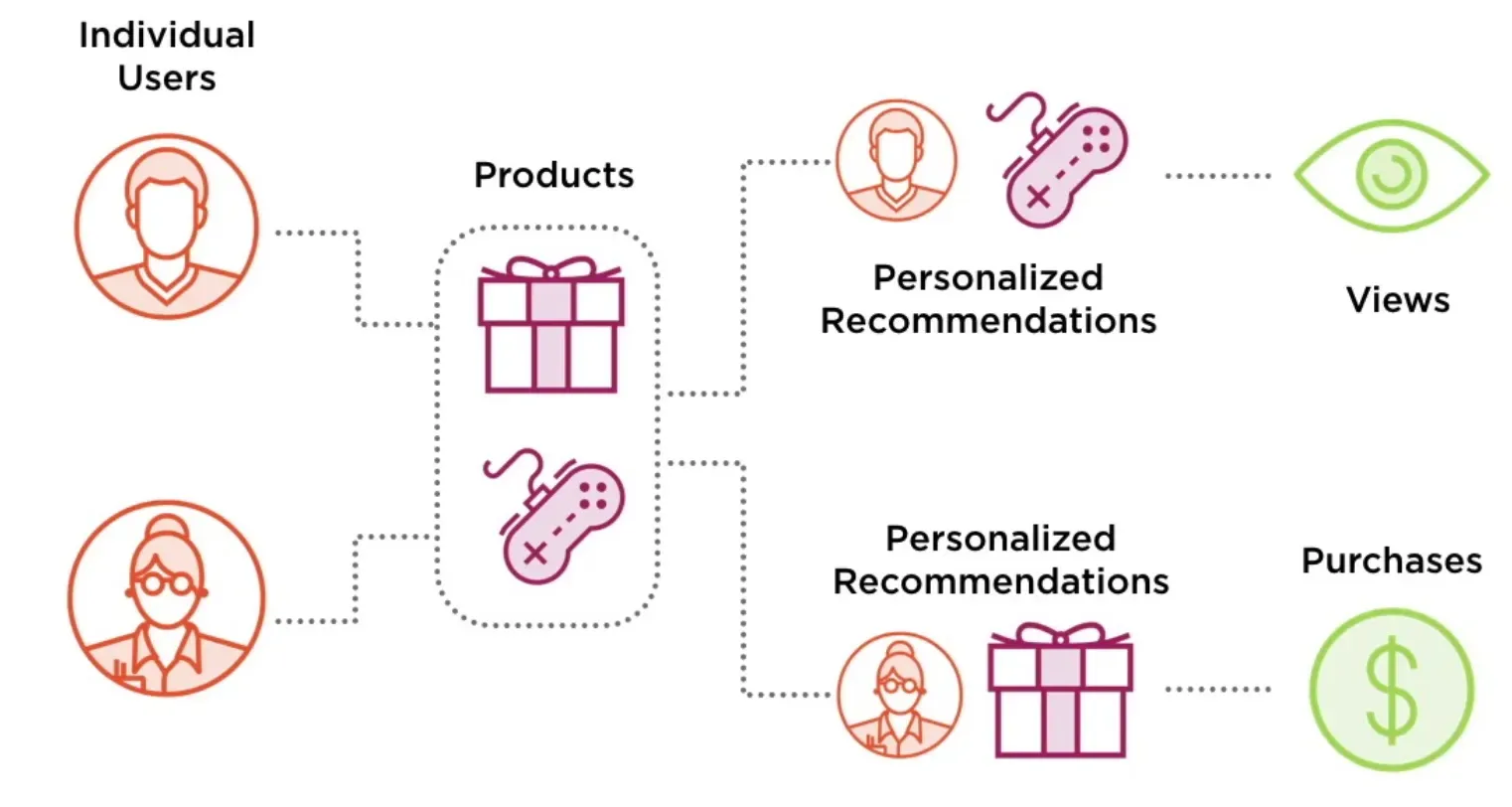
Item Attribute Identification
The first step is choosing clear, relevant attributes for each item that can be used for comparison, such as genre, tags, or descriptions.
User Profile Creation
Building a user profile involves collecting data on the specific attributes of items that a user has interacted with and liked.
Recommendation Generation
Recommendations are generated by comparing the item attributes with the user's profile and predicting the user's affinity for new items.
Feedback Incorporation
Content-based systems often include a feedback loop, allowing users to give a thumbs up or down, refining their profile and improving future recommendations.
Continuous Learning
Over time, the system learns and adapts to subtle shifts in the user's tastes, leading to more sophisticated and accurate recommendations.
Why is Content-Based Filtering Important?
Let's explore the compelling reasons behind the growing importance of content-based filtering.
Personalization
Content-based filtering offers deep personalization, providing users with content tailored specifically to their interests and past behavior.
Direct Relationships
This technique relies on the direct relationship between user preferences and content attributes, making recommendations explainable.
Independence from User Base
Content-based filtering doesn't need a large cohort of users, making it applicable for new or niche platforms.
Content Discovery
It helps users discover content that aligns with their tastes, which might otherwise be overshadowed by popular trends.
Customer Engagement
Providing relevant recommendations increases user engagement and satisfaction, leading to higher retention rates for platforms.
Challenges in Content-Based Filtering
Indeed, content-based filtering isn't a bed of roses; it comes with its own set of thorns to navigate through.
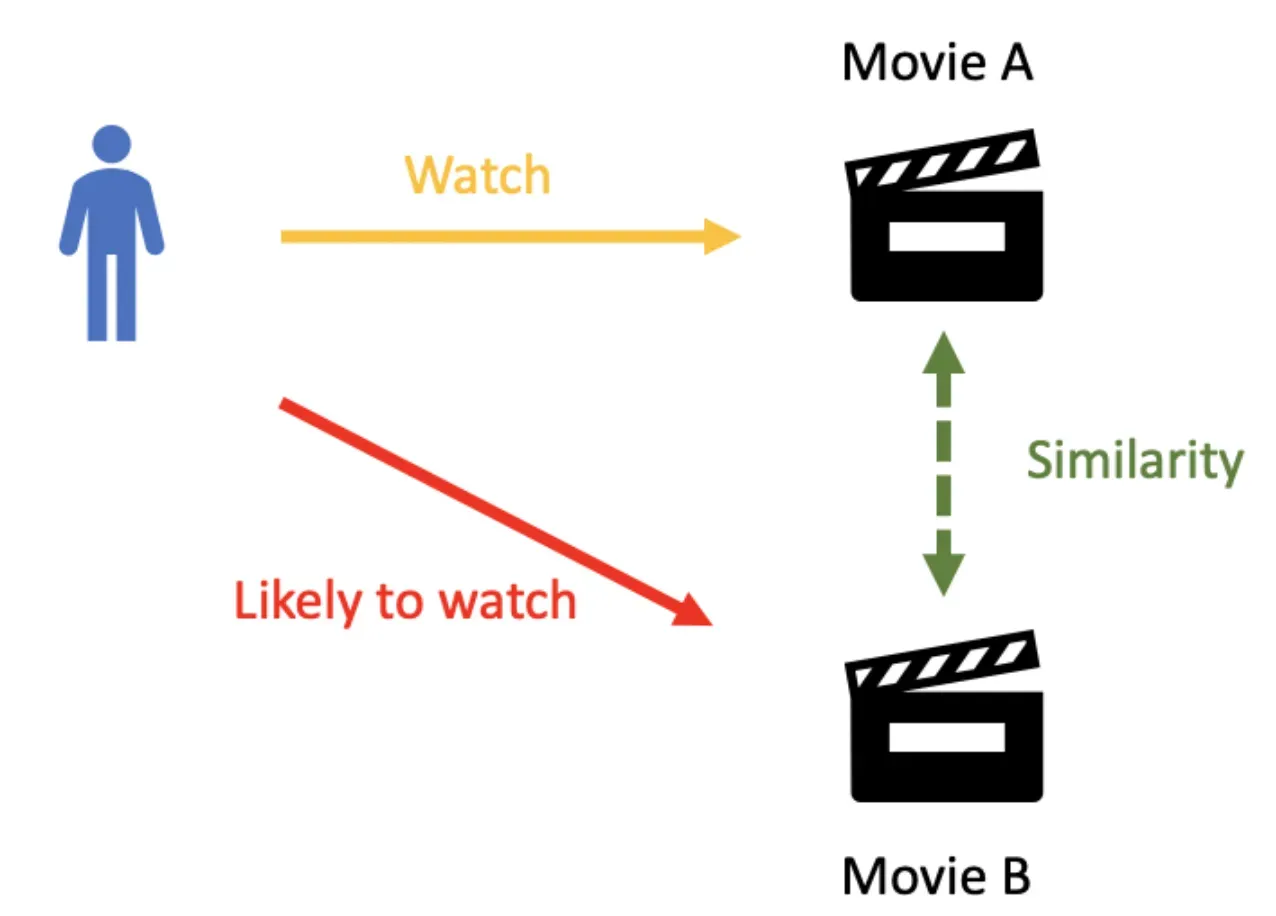
Over-Specialization
A major critique is the "filter bubble" where users are repeatedly offered similar items, potentially limiting diverse exposure.
New User Problem
For new users with limited interaction history, generating accurate recommendations can be a challenge.
Limited Serendipity
Content-based filtering may not offer serendipitous recommendations, potentially missing out on expanding a user's taste.
Attribute Limitations
The effectiveness hinges on the quality and depth of item attributes included, which might not always capture the essence of the content.
Dynamic Preferences
User preferences can evolve, requiring the system to promptly adapt to remain effective.
Best Practices for Content-Based Filtering
To harness the utmost potential of content-based filtering, here's a roundup of best practices.
Comprehensive Attribute Selection
Select item attributes comprehensively to ensure a rich basis for comparison and recommendation.
Balancing Novelty and Relevance
Introduce a blend of familiarity and novelty in recommendations to keep users engaged and exploring.
Dynamic Profile Updates
Continuously update user profiles to capture the evolving nature of their preferences and behaviors.
Transparency
Be transparent about how recommendations are determined to build trust and offer control to the user.
A/B Testing
Regularly A/B test different attributes and algorithms to optimize the performance of the recommendation system.
Trends in Content-Based Filtering
Staying attuned to the latest trends can chart the path forward for content-based filtering.
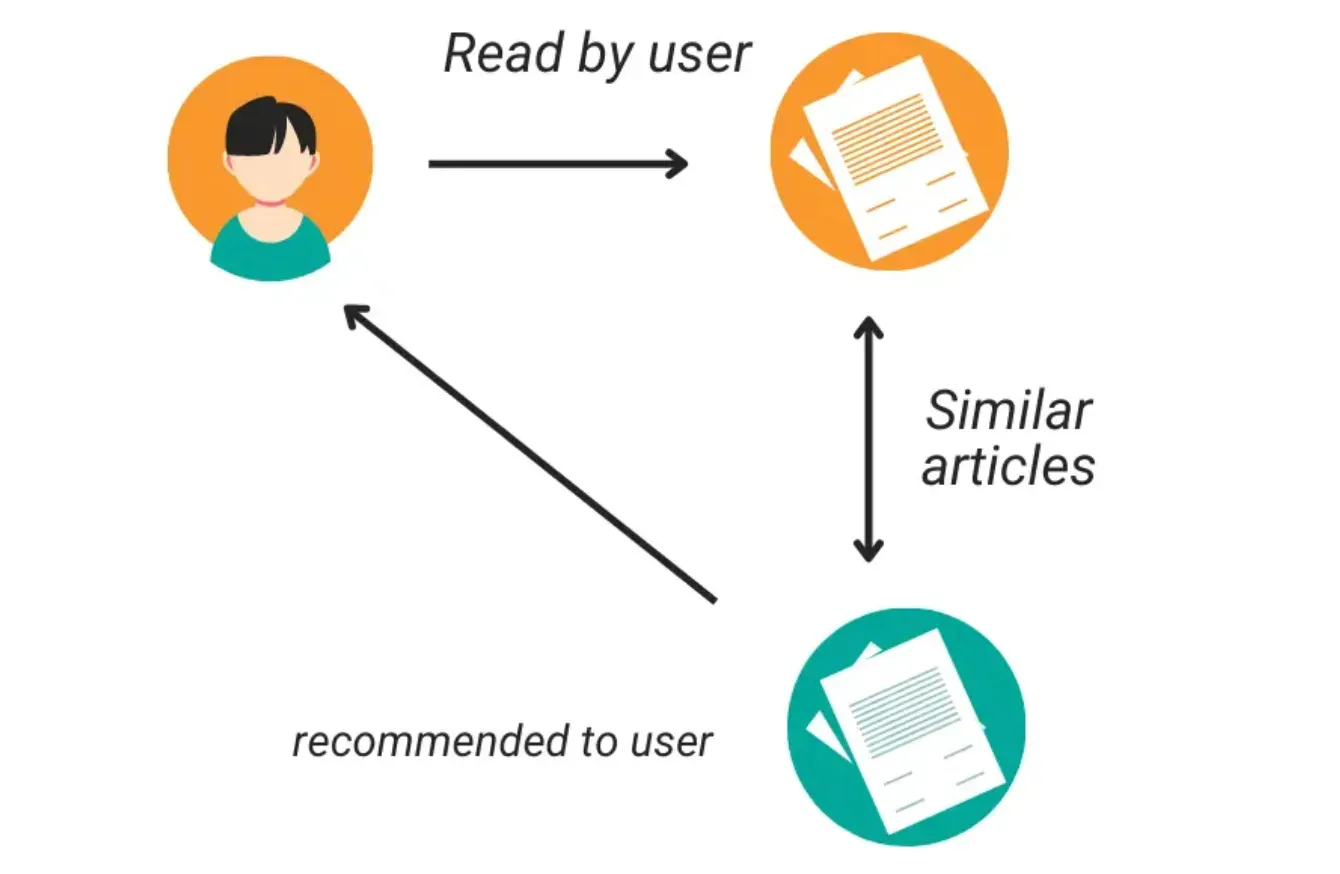
Leveraging AI and ML
Artificial intelligence and machine learning are being increasingly intertwined with content-based filtering, enhancing accuracy and personalization.
Integration with Other Recommendation Systems
Hybrid systems that integrate content-based filtering with collaborative and other methods provide a more rounded approach to recommendations.
Use of Deep Learning
Deep learning allows for the extraction of complex features and patterns from content, offering more nuanced recommendations.
Emphasis on Context
Incorporating contextual information, such as the time of day or device used, further refines recommendation accuracy.
Ethical and Privacy Considerations
There's a growing emphasis on ethical algorithms and privacy-preserving recommendation systems in response to data consent concerns.
In the mosaic of recommendation systems, content-based filtering holds its unique tile, colored by the intricate details of content and the nuanced preferences of users.
It's a continual dance between offering the comfort of the familiar and the thrill of the new, pushing the boundaries of how content meets the user - in the personalization spotlight, orchestrating experiences one recommendation at a time.
Frequently Asked Questions (FAQs)
How Does Content-Based Filtering Personalize User Experience?
Content-based filtering recommends items by comparing content of user's previously liked items to those they haven't interacted with, thus personalizing experience.
Can Content-Based Filtering Handle Cold Start Problem?
Since recommendations rely on user's past behavior, content-based filtering doesn't require other user data, effectively handling the cold start problem.
Does Content-Based Filtering Require Item Characteristics/Features?
Yes, it necessitates the availability of item characteristics or metadata to make accurate and relevant recommendations.
Can Content-Based Filtering Create an Echo Chamber Effect?
By only suggesting similar items, content-based filtering can potentially limit exposure to diverse content, sometimes leading to an echo chamber effect.
How Does Content-Based Filtering Mesh with Other Recommendation Systems?
Often, it's coupled with collaborative filtering in hybrid systems to balance the strengths and weaknesses of both techniques, improving recommendation diversity and relevance.