What is Collaborative Filtering?
Collaborative Filtering is a method utilized in recommendation systems, rooted as early as the mid-1990s.
When e-commerce started to boom, it quickly became evident that personalized recommendations were crucial to improving customer experiences and, ultimately, sales. This was the birthplace of the concept.
In its essence, Collaborative Filtering (CF) predicts the interests of a user by collecting preferences from many users.
The principle is straightforward: if person A has the same preference as person B, A is more likely to have B's preference for a different product.
Collaborative Filtering in real-world applications
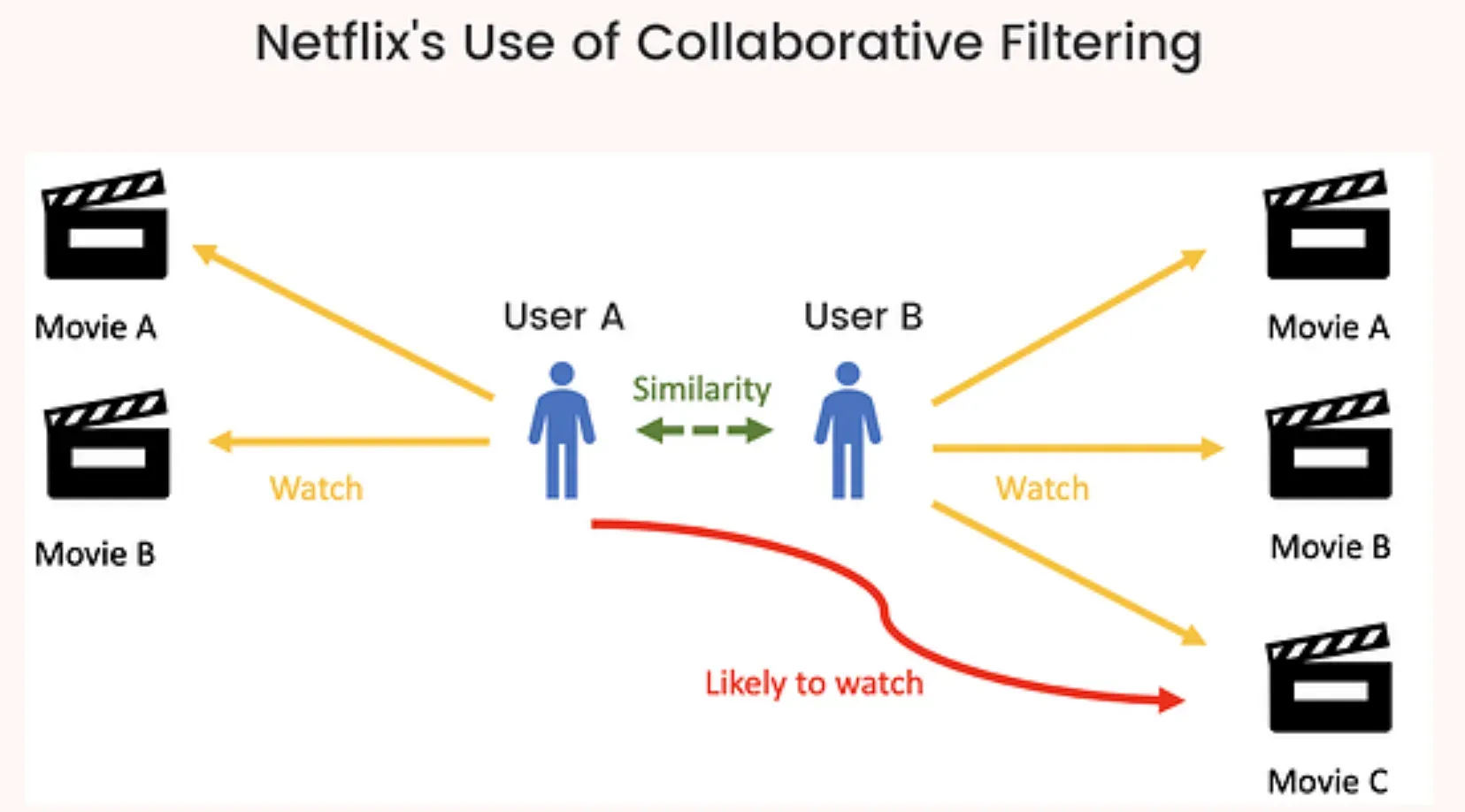
You probably interact with CF more than you realize. Ever notice how Netflix suggests shows you might like, or Amazon recommends products based on your shopping behavior?
That's CF at work, helping you discover new content or items based on your and other user's preferences.
With the internet's vast array of choices, it would be nearly impossible to vet every potential movie, item, or article out there.
CF helps solve this problem by narrowing down options based on collective behavior, making our online experiences more personalized and efficient.
Types of Collaborative Filtering
Following is a detailed look at the types of Collaborative filtering:
User-User Collaborative Filtering
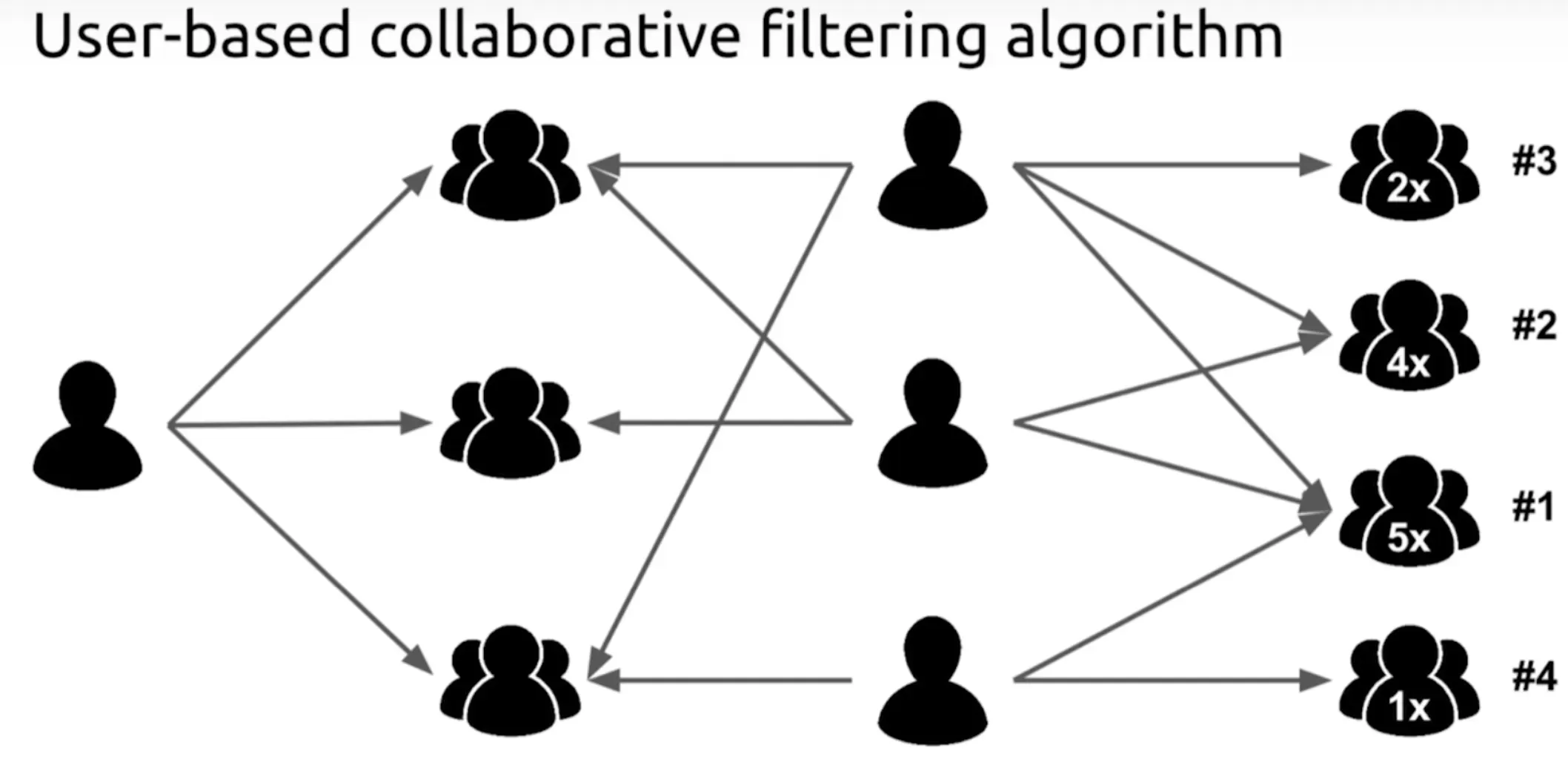
This form of CF involves predicting an item's rating or preference for a user based on ratings or preferences from "neighboring" users who have similar tastes.
It's like asking your friend for a movie suggestion because they often have similar movie preferences.
Item-Item Collaborative Filtering
Here, product recommendations for a user are made by comparing items based on user ratings, rather than user-user relationships.
This is akin to saying 'Customers who bought this item also bought...,' an often-encountered prompt in online shopping.
Hybrid Collaborative Filtering
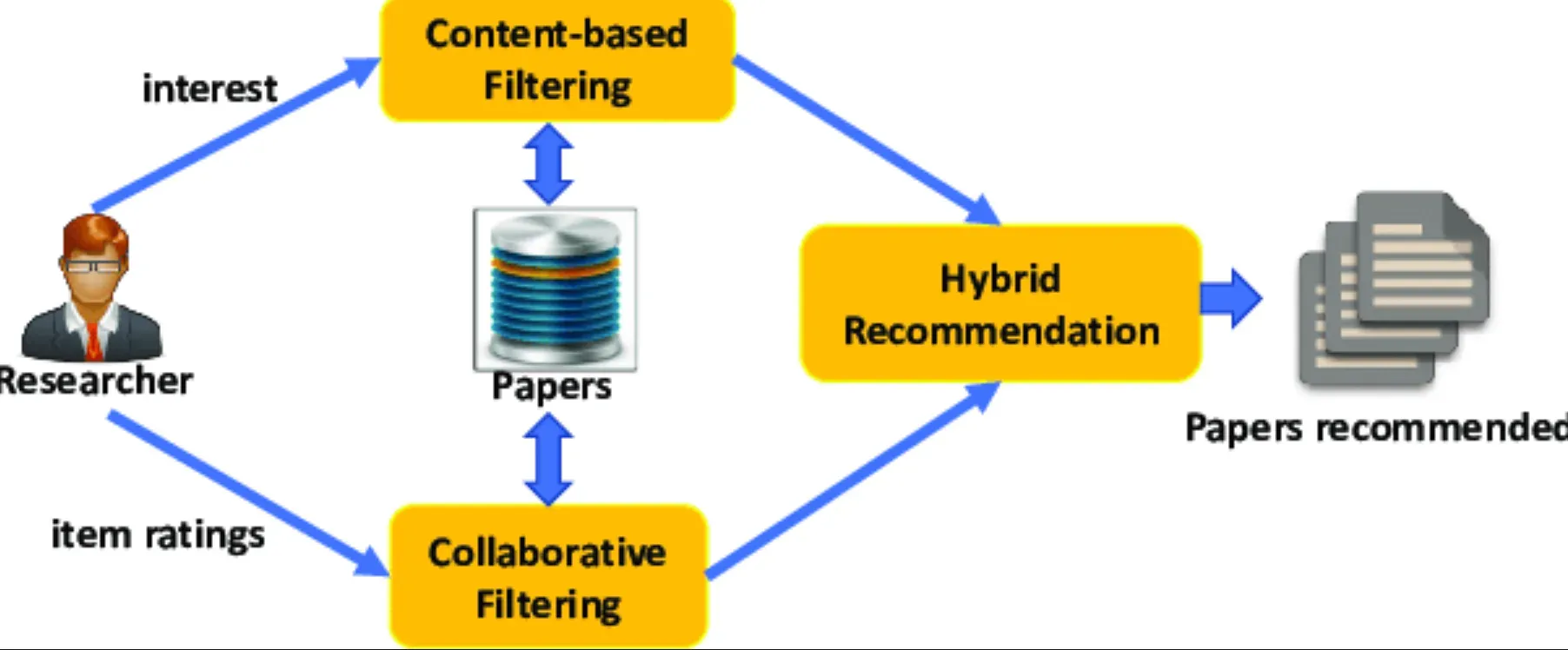
Combining the strengths of user-user and item-item filtering, hybrid filtering aims to benefit from both to overcome their individual drawbacks.
For instance, it might employ user-user filtering and switch to item-item when suitable “neighbors” for a user aren’t available.
Model-based Collaborative Filtering
When patterns in user-item matrices are modeled using machine learning algorithms, it's referred to as model-based CF.
This can counteract scalability issues inherent in the previous methods, as it provides more manageable, reduced representations of the user-item matrix.
Advantages of Collaborative Filtering
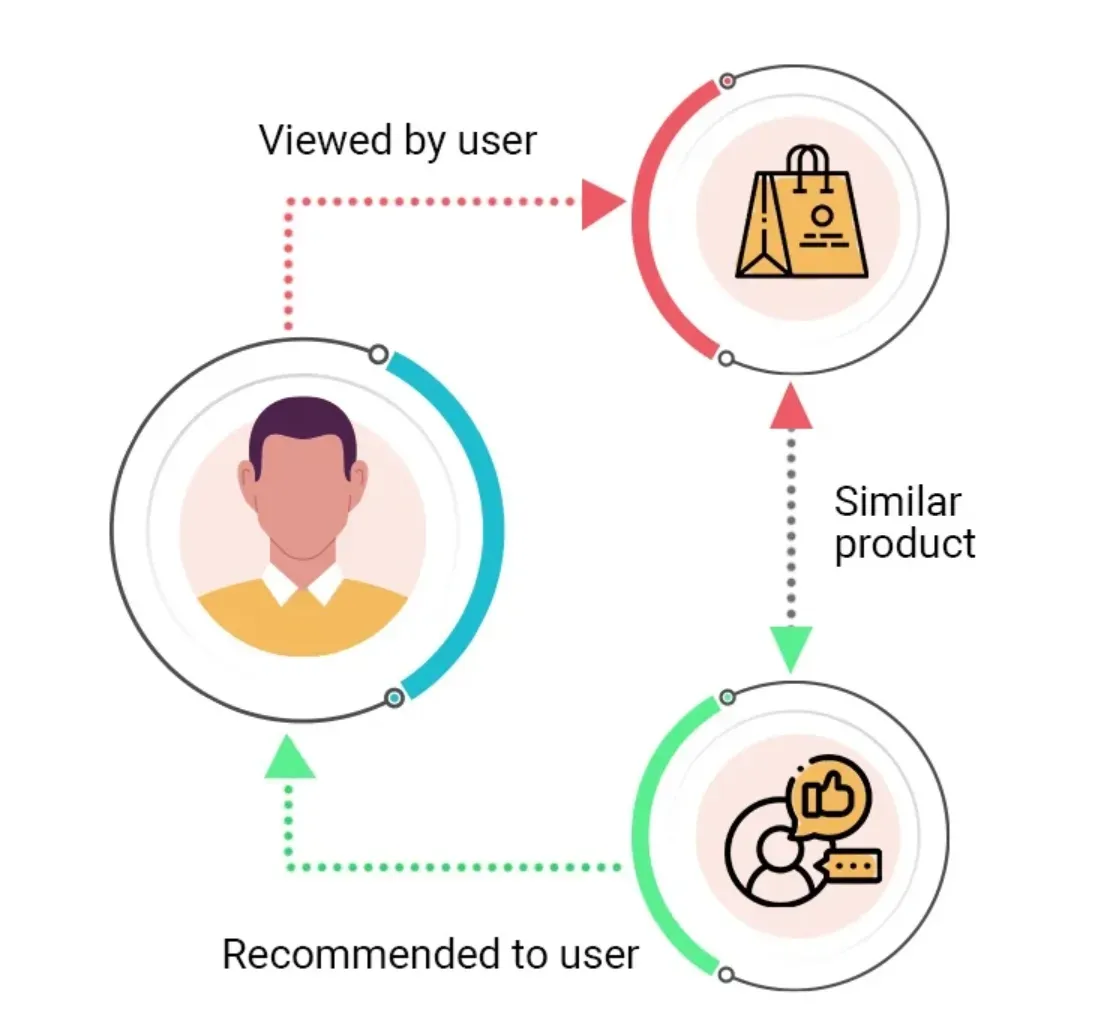
CF operates entirely on user behavior data. This user-centric approach means that the system doesn't need to understand anything about the items being recommended - it recommends based on observed interactions only.
Can make unexpected recommendations
Unlike content-based filtering (which merely suggests similar items to those a user has liked in the past), CF can suggest items that are totally different from a user's previous interests, but liked by similar peers.
For instance, if your movie-watching habits plot you as a sci-fi fan, content-based filtering would recommend more such movies.
But CF might suggest a highly-rated art-house film because users similar to you also happen to like it. This can expand your horizons!
Works for any kind of item
Whether your platform deals in films, music, products, or even news articles, collaborative filtering can work. All it needs is some user feedback (like ratings or viewing history) to operate.
Learn and Adapt
As users continue to provide feedback, the system continually learns, updates, and adapts to changes in user behavior. Over time, the system's recommendations become more accurate.
Challenges with Collaborative Filtering
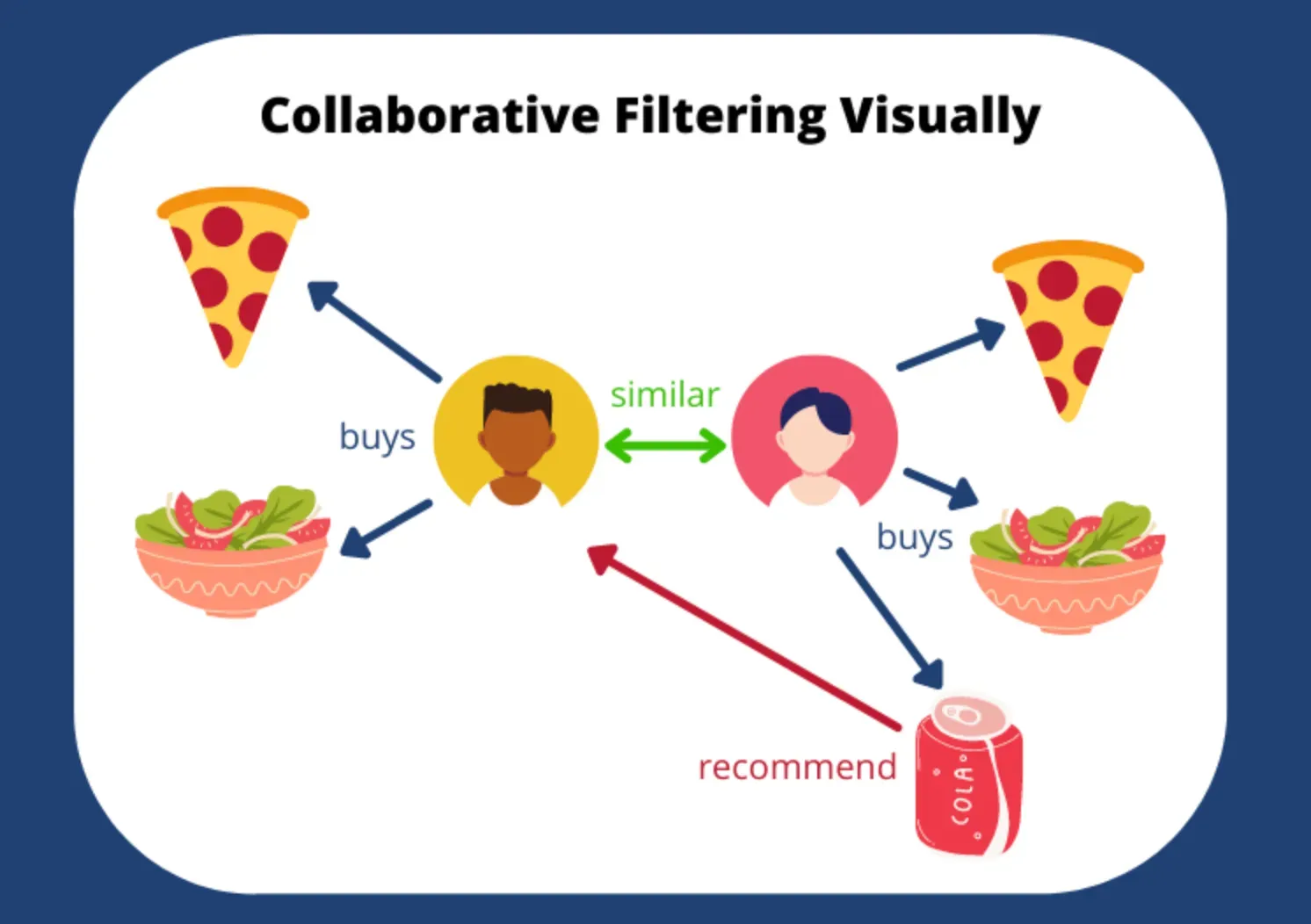
Cold Start
A new user who hasn’t yet provided any feedback poses a challenge for CF. Without any behavior to analyze, how can the system make recommendations?
This is the 'cold start' problem. The same applies when adding a new item to the system without any interactions tied to it.
Sparsity
As the number of products and users grows, the user-item interaction matrix used by CF systems becomes increasingly sparse (most items are unrated).
This can make it hard to find neighbors with similar behavior, particularly in a user-user CF system.
Scalability
The computation cost of CF grows with both the number of users and items. In large-scale systems, predicting preferences across millions of users and items can be both time-consuming and resource-intensive.
Popularity Bias
CF has a tendency to suggest popular items, potentially overlooking niche items that could be relevant to the user.
This is because it's much easier to find correlations between popular items than those that have been interacted with less.
Overcoming Challenges in Collaborative Filtering
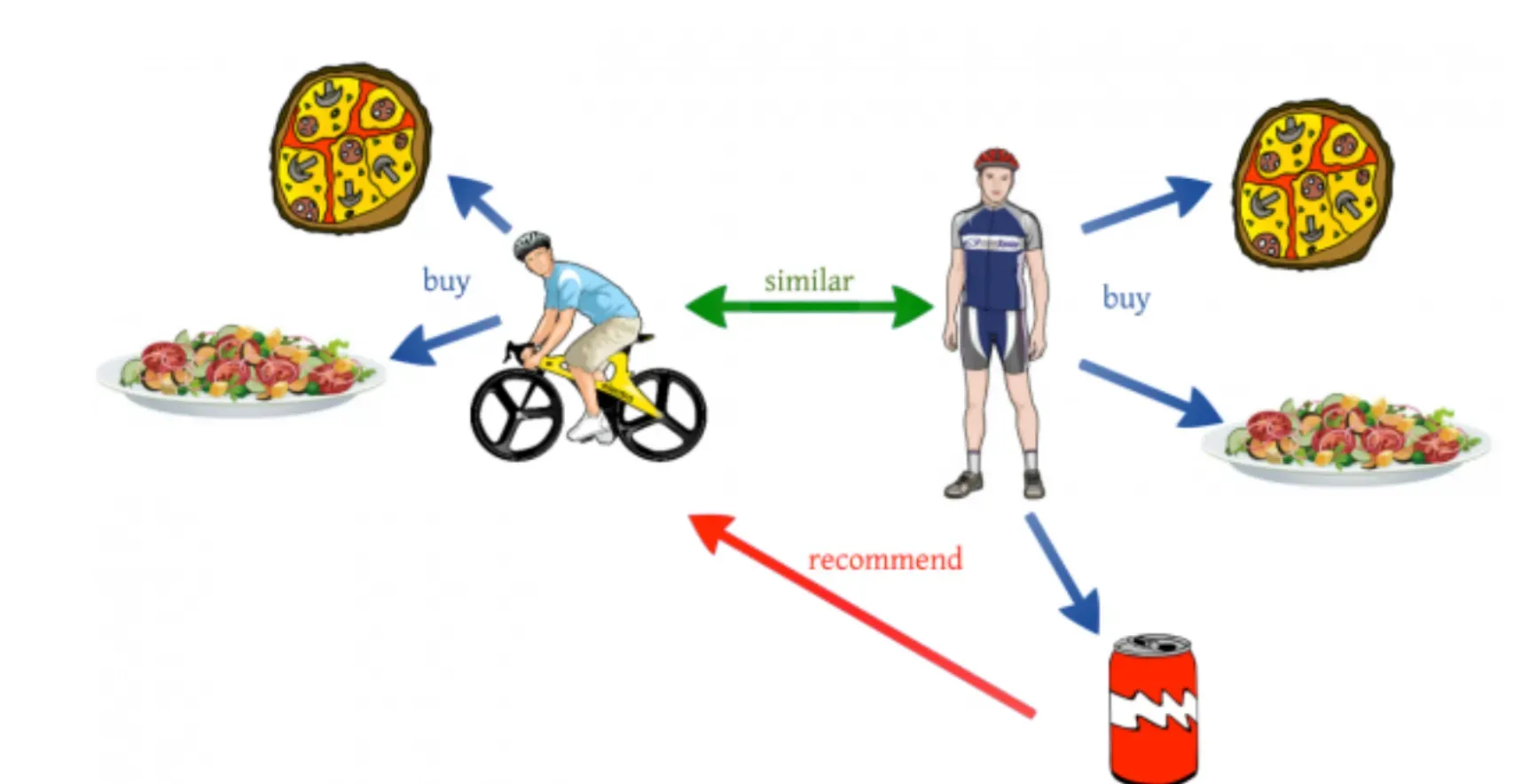
- Addressing Cold Start: Hybrid filtering methods, which combine CF with other techniques (like content-based filtering), can help solve the cold-start problem, providing a level of personalization to new users and helping promote new items.
- Tackling Sparsity: Model-based CF, using machine learning methods, can deal with the issue of sparsity. These methods can uncover latent factors that explain observed ratings and help predict missing ones.
- Downscaling Computation: Using dimensionality reduction techniques and optimizing the algorithms can help to handle the scalability issues.
- Balancing Popularity Bias: To avoid favoring only popular items, methods like diversity enhancement and adjusting the ratio favoring long-tail items can be used.
Example Use Cases of Collaborative Filtering
- E-commerce: One of the most prominent users of CF is e-commerce. It leverages user behavior to suggest products and thereby improve cross-selling and upselling.
- Media and Entertainment: Whether it is music or video streaming, CF abilities to personalize media consumption are revolutionizing the industry.
- Social Media: Social media platforms use CF to suggest friends, posts, or pages based on user behavior. It helps improve user engagement on the platform.
- News and Article Recommendation: CF finds its benefit here as well, personalizing user reading experiences by suggesting articles in line with their interests or reading patterns.
Frequently Asked Questions (FAQs)
What is the primary purpose of Collaborative Filtering (CF)?
The main objective of CF is to predict and recommend items that a user might like based on the historical behavior of similar users.
Why is Collaborative Filtering so essential in a world with advancing AI technology?
CF provides a robust and successful approach to personalizing user experiences across various platforms.
Its importance grows with wider adoption of AI, as personalization is seen as a key differentiator in modern AI technologies.
How does Collaborative Filtering differ from Content-based Filtering?
While content-based filtering recommends based on the similarity of items a user has already interacted with, CF uses the behavior of other users to recommend items.
What are some common applications of Collaborative Filtering?
CF is widely used in recommendation systems, like those in Netflix, Amazon, and YouTube, to suggest movies, products, videos, songs, etc., to a user.
What are some challenges faced using Collaborative Filtering?
CF faces issues like the cold start problem, sparsity, scalability problems and popularity bias, but there are methods available to overcome these challenges.