What is Knowledge Engineering?

Knowledge Engineering is a field of AI that focuses on creating systems capable of emulating the decision-making abilities of human experts in a specific domain. By developing rules and models, it enables computers to process and analyze data just like a human would, leading to intelligent and informed decision-making.
History of Knowledge Engineering
In the early days, Knowledge Engineering solely concentrated on transferring human expertise into computer programs, but it soon became apparent that this approach had limitations.
It didn't capture the nuances of human decision-making, such as intuition and gut feelings.
Additionally, these systems lacked collateral information that human experts possess.
Over time, Knowledge Engineering evolved into a modeling process that aimed to achieve expert-level results using different approaches and information sources.
What is the Purpose of Knowledge Engineering?
In this section, we'll explore the essential elements involved in knowledge engineering, a multidisciplinary field concerned with designing and implementing knowledge-based systems.
Knowledge Acquisition
Knowledge acquisition is the process of gathering domain-specific expertise from various sources, including domain experts, databases, and documents. This often involves interviewing experts, conducting surveys, and studying existing literature.
Knowledge Representation
This element covers the methods and techniques used to store and structure the acquired knowledge. Common techniques include semantic networks, rule-based systems, frames, ontologies, and decision trees, all aimed to allow efficient retrieval and reasoning.
Reasoning and Inference
Reasoning and inference involve using the represented knowledge to deduce new information or draw conclusions. Common types of reasoning include deductive reasoning, inductive reasoning, and abductive reasoning. Inference engines are crucial components of knowledge-based systems.
Validation and Verification
Verification ensures that the knowledge-based system functions correctly, whereas validation aims to confirm that the correct problem is being solved. Techniques like simulation, prototyping, and expert validation are used to assess the efficacy and functionality of the system.
Maintenance and Refinement
Knowledge engineering is an iterative process, which requires regular maintenance and refinement. This involves updating the knowledge base to account for new information, correcting errors, and optimizing the system's behavior.
Benefits of Knowledge Engineering
Knowledge Engineering offers several benefits, including:
- Creating Better Expert Systems: Knowledge Engineering helps in building smarter and more effective expert systems that can make informed decisions.
- Handling Complicated Issues: By leveraging knowledge from various domains, Knowledge Engineering enables expert systems to tackle complex problems.
- Robustness of Expert Systems: Expert systems built using the Knowledge Engineering process tend to be more robust and reliable.
- Natural Language Programming (NLP) Integration: When combined with NLP, expert systems built with Knowledge Engineering can understand queries and provide answers and solutions, similar to the behavior of chatbots.
Knowledge Engineering Process
The Knowledge Engineering process involves several crucial steps:
Task Definition
In this phase, the initial task is defined and clarified. The goal is to understand the problem that needs to be solved or the question that needs to be answered. This includes identifying the specific user requirements and objectives.
Knowledge Gathering
Once the task is defined, the next step is to gather relevant knowledge and information. This involves researching existing sources such as articles, books, databases, or other experts in the domain. The information gathered should be reliable and up-to-date to ensure accurate knowledge encoding.
Roadmap Development
Creating a roadmap is crucial to outline the steps and milestones that need to be achieved throughout the knowledge engineering process. This phase includes identifying the key concepts, dependencies, and relationships between different elements of knowledge.
Knowledge Encoding
Knowledge encoding involves representing the gathered knowledge in a structured and machine-readable format. This can be done using various techniques such as ontologies, semantic networks, rules, or machine learning models. The encoded knowledge should be easily consumable and usable by the system.
Evaluation and Debugging
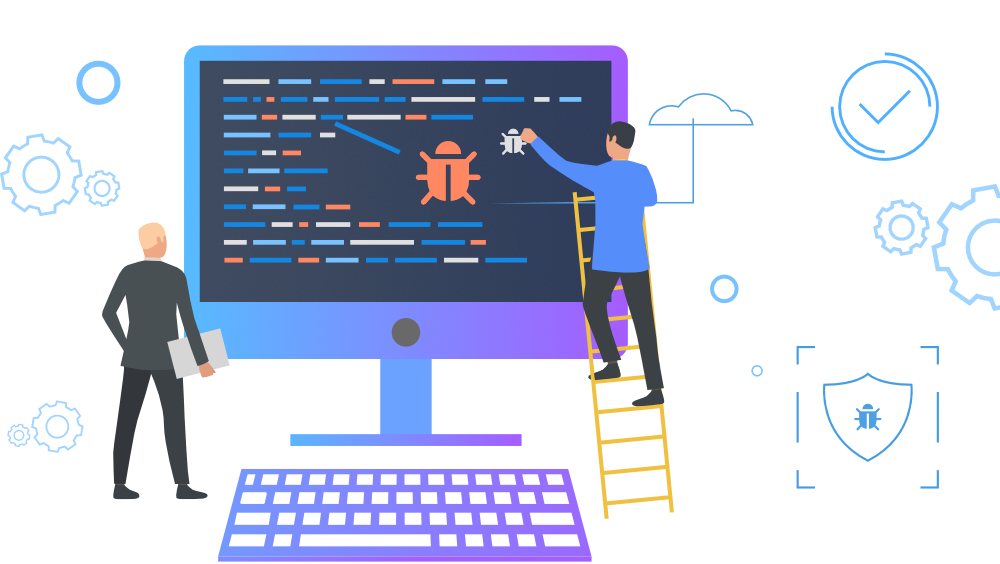
Once the knowledge is encoded, it needs to be evaluated and tested for accuracy and performance. This phase involves verifying the encoded knowledge against test cases or user queries. Any errors or inconsistencies are identified and debugged to improve the quality of the knowledge base.
What are the applications of Knowledge Engineering?
Among the earliest applications of knowledge engineering was in the field of medical diagnosis, with systems such as MYCIN that used knowledge-based approaches to identify diseases and recommend treatment.
Knowledge Management Systems
Knowledge Engineering is instrumental in the development of knowledge management systems. These systems enable organizations to capture, categorize, and utilize knowledge generated within.
Information Retrieval Tools
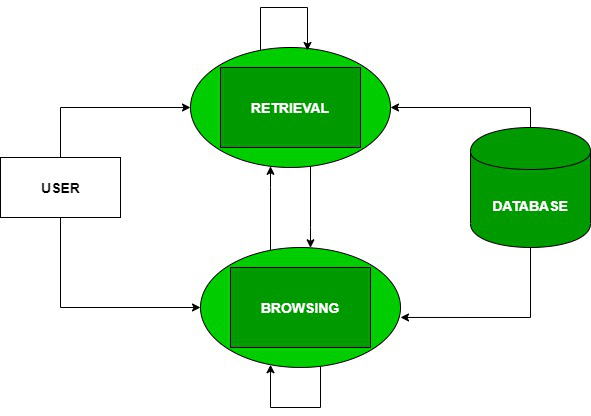
Information retrieval systems leverage knowledge engineering to quickly and efficiently respond to queries. These systems, whether semantic or non-semantic, significantly benefit from the principles of knowledge representation and reasoning encapsulated in knowledge engineering.
Decision Making and Problem Solving
Knowledge engineering can enhance decision-making and problem-solving processes by creating logical deductions from the existing knowledge base. This assistance can be invaluable in a variety of contexts including business strategy, scientific research and healthcare.
Design
Design is another area where knowledge engineering finds application. Knowledge management techniques help build collective knowledge representations, contributing greatly to improving and enriching the design process.
These examples only touch upon a fraction of the potential use-cases for knowledge engineering, underlining the profound influence this field has across diverse domains.
Role of Knowledge Engineering in AI
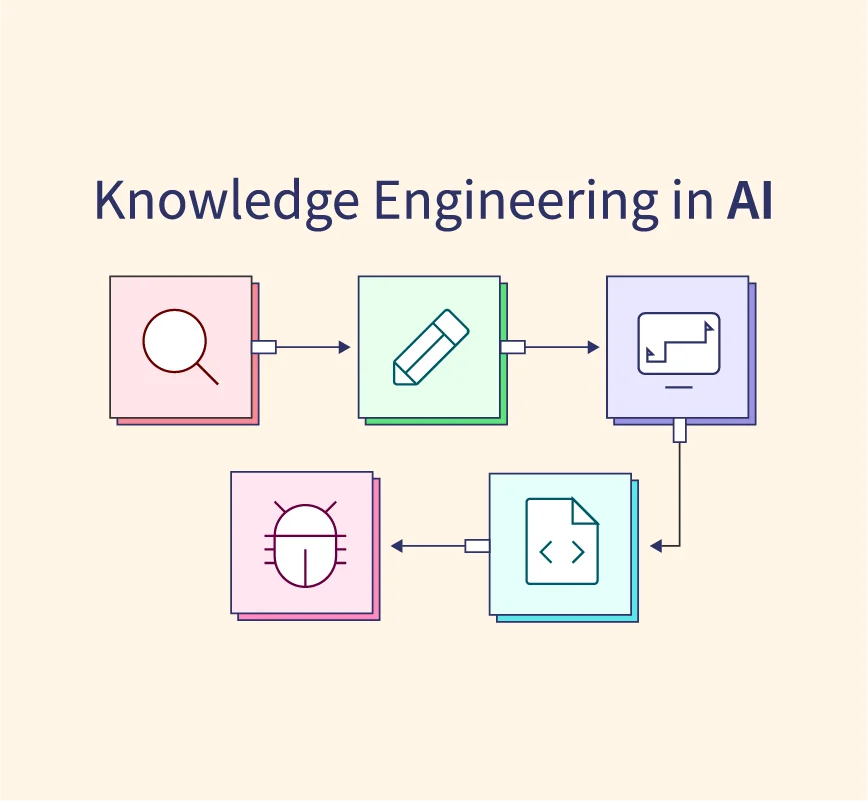
Knowledge engineering plays a pivotal role in AI by coding vast chunks of human knowledge into systems, thus enabling them to mimic human-like decision-making. It forms the backbone of AI systems, providing a structure for data that allows machines to understand and learn from it.
Techniques Deployed in Knowledge Engineering
Techniques employed in knowledge engineering range from rule-based systems and decision trees to neural networks. These allow AI systems to analyze data, learn patterns, reason, and make decisions akin to human intelligence.
Knowledge Engineering in Action
Whether it's natural language processing used in virtual assistants or creating expert systems that help doctors diagnose diseases, knowledge engineering powers numerous AI applications. It is the force behind machine learning that enables systems to learn from data and improve over time.
The Road Ahead for Knowledge Engineering and AI
As AI continues to become increasingly sophisticated, the role of knowledge engineering will keep intensifying. Harnessing human knowledge and embedding it into machines will continue leading the advancement of AI, making systems more intelligent and effective.
Key Elements of Knowledge Engineering
Three crucial elements in the advancement of knowledge engineering are the semantic web, cloud computing, and open datasets:
- Semantic web: The semantic web provides a standardized and well-defined framework for organizing and linking information on the internet. This standardization allows knowledge engineers to give meaning to data, facilitating effective knowledge representation and retrieval. Semantic web technologies such as RDF (Resource Description Framework) and OWL (Web Ontology Language) are key tools for knowledge engineering.
- Cloud computing: Cloud computing has revolutionized the storage, processing, and access to computational resources. It offers scalability and flexibility, enabling knowledge engineers to harness immense computing power for knowledge engineering tasks. The cloud provides a cost-effective and efficient platform for building and deploying expert systems.
- Open datasets: The availability of open datasets has significantly contributed to the advancement of knowledge engineering. These datasets, freely accessible to the public, provide a wealth of information that can be used for training and validation purposes. Leveraging open datasets enhances the accuracy and performance of expert systems, ensuring they are trained on diverse and representative data.
Frequently Asked Questions (FAQs)
What are some popular tools used in knowledge engineering?
Popular tools used in knowledge engineering include ontology development tools, knowledge graph frameworks, rule-based systems, and machine learning platforms.
How is knowledge engineering related to artificial intelligence?
Knowledge engineering is closely related to artificial intelligence as it involves designing systems that can mimic human intelligence by utilizing expert knowledge and reasoning capabilities.
Can knowledge engineering be applied to any industry or domain?
Yes, knowledge engineering can be applied to any industry or domain that requires expertise and decision-making. It has been successfully implemented in fields such as healthcare, finance, manufacturing, and customer service.
What is the difference between knowledge engineering and data engineering?
While data engineering focuses on collecting, storing, and processing large amounts of data, knowledge engineering is concerned with capturing and utilizing expert knowledge to solve complex problems.
Is knowledge engineering a rapidly evolving field?
Yes, knowledge engineering is constantly evolving due to advancements in artificial intelligence and machine learning. New techniques and tools are being developed to improve knowledge representation, acquisition, and utilization.