Introduction
Language models are transforming how we communicate. Recent breakthroughs allow AI to generate human-like text and understand natural language. But training these powerful models comes with big challenges.
How do we make them fair, safe, and affordable? This post explores the frontiers of language model research. We'll look at innovations like GPT-3 that push boundaries in language generation. And consider issues like bias.
Conversational agents, machine translation, and more could shape our future. But first researchers must grapple with risks like misuse of fake news.
Join us as we dive into the opportunities and unknowns ahead in language AI. So, let us start our blog by learning about the recent trends and advancements in large language training models.
Recent Trends and Advancements in Large Language Models
In recent years, there have been significant advancements in training large language models, including:
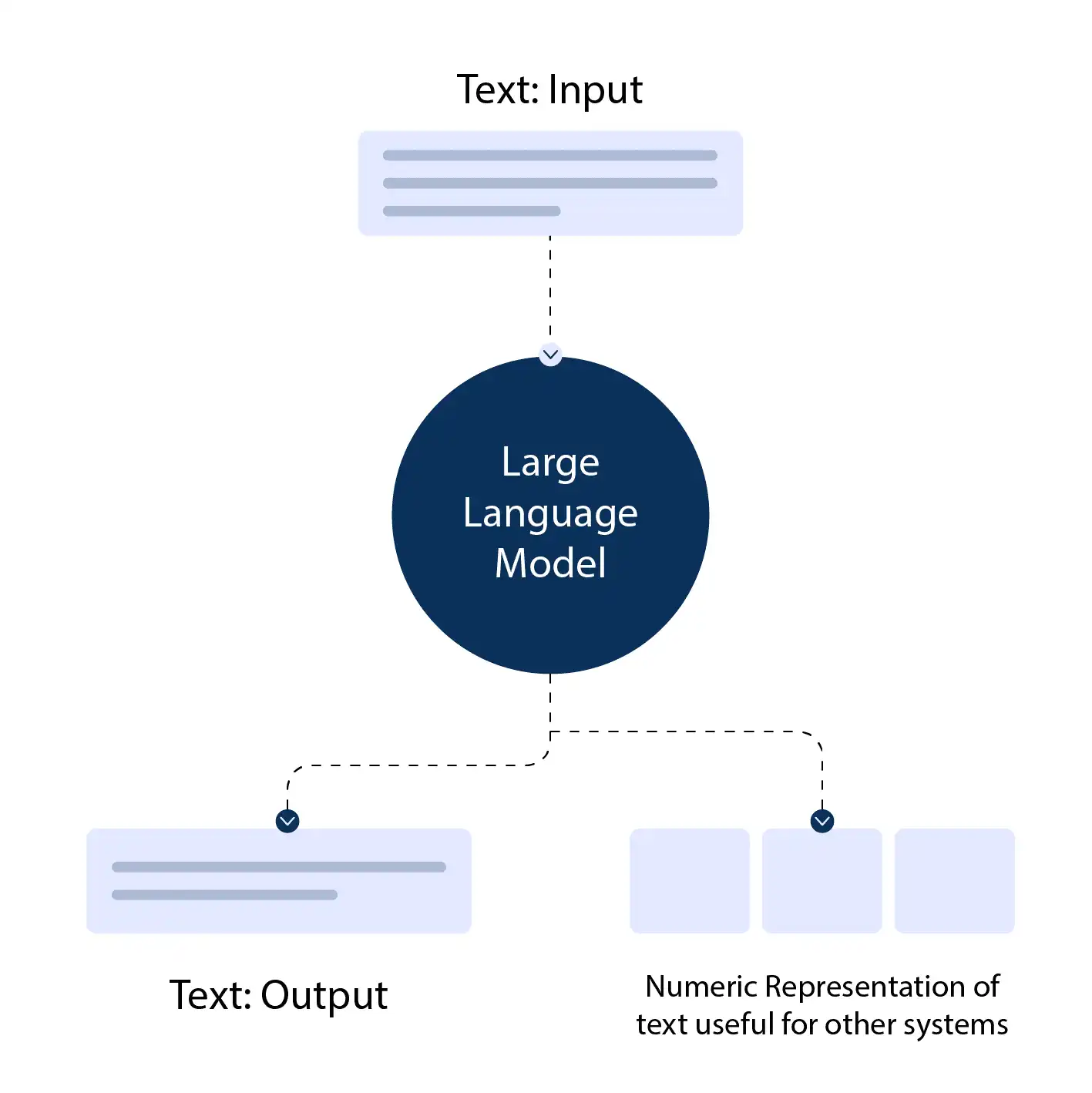
Pre-Training and Fine-Tuning Techniques
Pre-training is a process where a model is trained on a large and diverse text corpus, such as the entire internet, to learn general language representations.
The model is then fine-tuned for a specific task, like sentiment analysis or text classification. This approach has shown impressive results in improving the performance of large language models.
- Integration of domain-specific knowledge: Large language models can be further trained on a particular domain, like legal texts or medical documents, to improve their performance on specific tasks.
This approach, known as domain adaptation, has been shown to improve the accuracy of large language models on certain tasks.
- Use of unsupervised learning algorithms: Unsupervised learning algorithms like clustering or autoencoding can be used to train large language models on unlabeled data.
These algorithms allow the models to learn the underlying structures of the language. Thus making them more adept at generating coherent and accurate language.
- Advancements in language modeling architectures: Language modeling architectures like GPT and BERT have shown impressive results in generating human-like text.
These architectures have been constantly evolving, with new variants like GPT-3, Turing-NLG, and T5 pushing the boundaries of language generation and understanding.
Challenges Facing Large Language Model Training

These challenges have led to several calls for responsible NLP research and development.
Addressing these challenges will require contributions and collaborations across various fields, such as computer science, ethics, and law.
High Computational Costs
Training large language models requires significant computational resources, making it a costly and time-consuming process. Training models such as GPT-3 require a multi-million dollar investment in infrastructure.
This can lead to difficulties for smaller research teams and organizations with limited resources, effectively excluding them from contributing to the latest breakthroughs in the NLP field.
Data Privacy and Bias Issues
Large language models learn from vast amounts of data, but this data can contain biases.
These biases can manifest as stereotypes or prejudices in the language generated by the model, which could have harmful consequences if the model were used in real-world applications such as hiring or lending.
Moreover, the data used to train these models can sometimes be private, and data breaches could compromise the privacy of individuals.
Ethical Concerns Surrounding the use of Large Language Models
There are ethical concerns surrounding how large language models are used, as their capabilities could be used for malicious purposes.
For example, the creation of convincing fake news, cyberbullying bots, and deep fakes.
Limited White-Box Interpretability of the Model's
Large language models can be opaque, making it difficult to understand how they generate or interpret language.
This limits their potential for examining and understanding subtle issues such as algorithmic bias or errors.
This also makes it difficult to verify their safety or appropriateness for certain applications, such as medical diagnosis or legal decision-making.
Potential Solutions to Overcome the Challenges
These potential solutions address various aspects of the challenges facing large language model training.
Distributed and Parallelized Training Approaches
To tackle the high computational costs of training large language models, researchers have been exploring distributed and parallelized training approaches.
The training time can be significantly reduced by distributing the workload across multiple machines or using parallel processing techniques. This approach requires specialized infrastructure and efficient data processing techniques.
Hybrid Architectures for Training with Fewer Data

Training large language models require vast amounts of data. However, in many domains, labeled data might be scarce.
One potential solution is a hybrid approach where the model is initially pre-trained on a large generic dataset and then fine-tuned on a smaller task-specific dataset.
This allows the model to benefit from the large corpus of pre-training data while adapting to the specific task requirements.
Suggested Reading:
Custom LLM Models: Is it the Right Solution for Your Business?
Adversarial Training for Improving Model Robustness and Fairness
Adversarial training involves training models using both "positive" and "negative" examples, where the negative examples are generated to challenge the model's performance specifically.
This approach can help improve the robustness of large language models by exposing them to various adversarial scenarios.
Adversarial training can also mitigate bias in the models by generating biased examples and training the model to be less influenced by them.
Techniques for Exploring and Mitigating Bias in the models
Addressing bias in large language models is critical for ensuring fairness and avoiding discriminatory outcomes.
Researchers are developing techniques to detect and mitigate bias in models, such as analyzing the training data for biases, using debiasing algorithms during training, or augmenting the training data with diverse examples.
Regular audits and public participation in the design and training process can further help identify and mitigate bias.
Future Directions and Opportunities for Large Language Models
These future directions and opportunities point towards significant growth in the capabilities of large language models and the potential impact they could have in various technical and business fields.
However, issues of privacy, security, ethics, and legal considerations should be taken into account while applying these technologies in these areas.
Multimodal language Models
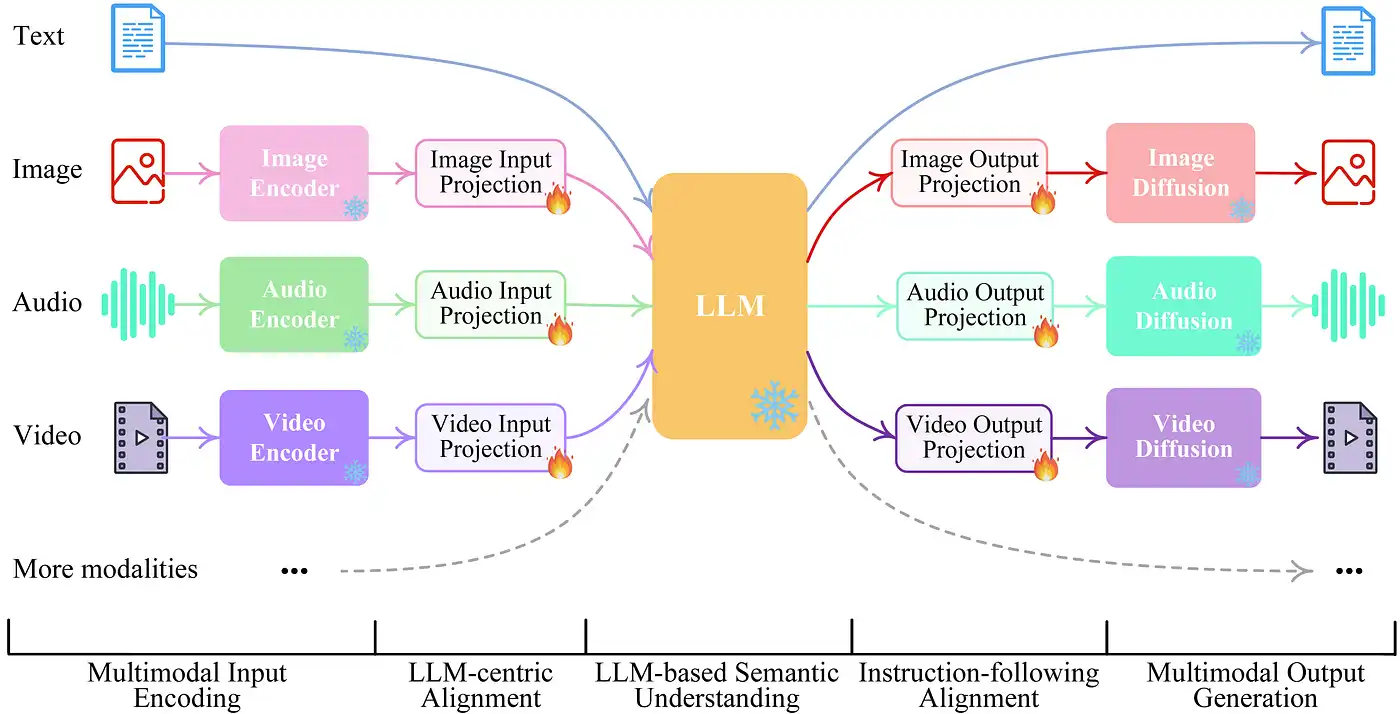
Multimodal language models can understand and generate language in conjunction with other forms of sensory input, such as images, audio, and video.
By incorporating visual cues and contextual information, these models can improve the accuracy, relevance, and comprehensibility of language generation and understanding tasks.
Multimodal models can have applications in areas such as augmented reality, self-driving cars, and virtual assistants.
Conversational Agents with Enhanced Capabilities
Large language models have already shown impressive performance in generating human-like dialogue.
However, there is still a long way to go in creating truly intelligent conversational agents with natural language understanding and reasoning capabilities.
By combining large language models with other AI techniques such as reinforcement learning, deep reinforcement learning, and memory networks, conversational agents could be created with enhanced capabilities and better mimic real human conversation.
Suggested Reading:
Things You Need to Know About Training Large Language Models
Neural Machine Translation
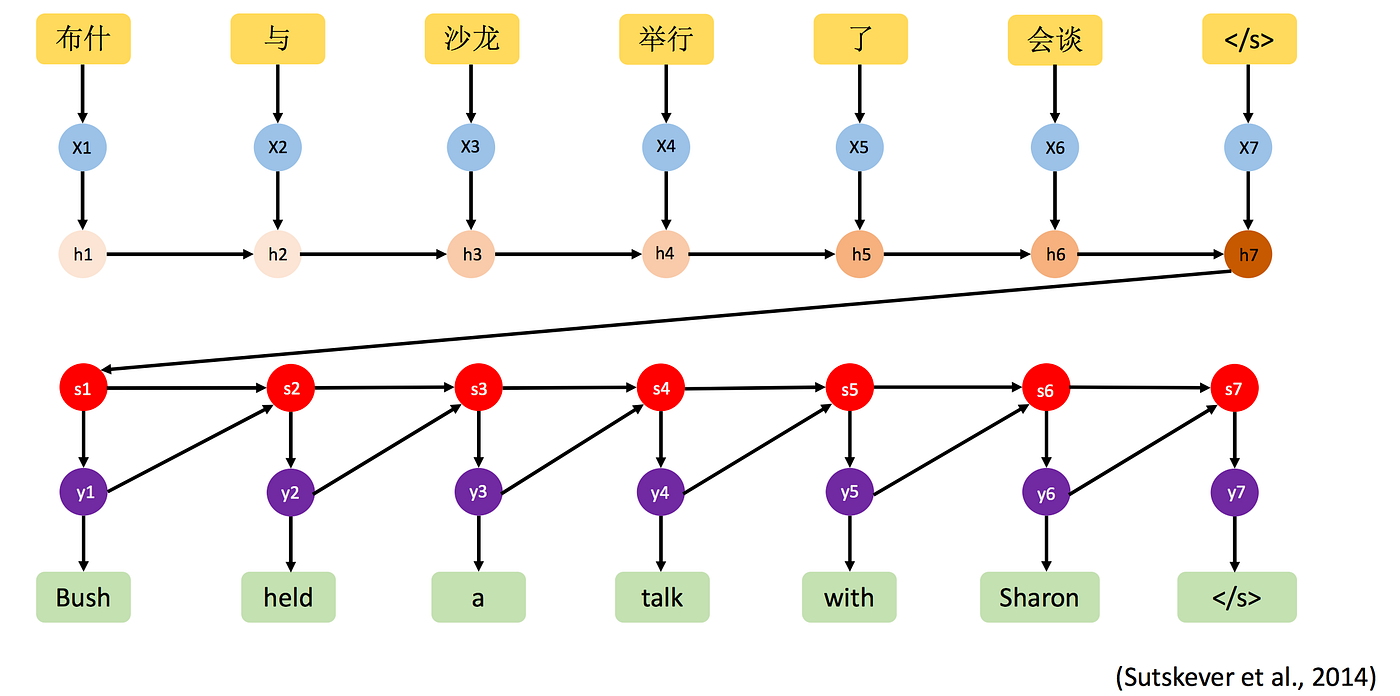
Neural machine translation (NMT) involves the use of neural networks to improve the accuracy and fluency of machine translation. Large language models can enhance NMT systems by improving their ability to handle syntax, semantics, and context.
The resulting models could enable easier communication and collaboration across different languages and cultures, opening up more opportunities for cross-border trade, tourism, and social interaction.
Applications in Healthcare and Finance
Large language models can also be applied in areas such as healthcare and finance. For example, text-generation tools could generate medical reports, discharge summaries, and physician notes, making medical documentation easier and faster.
In finance, natural language processing and generation could provide personalized recommendations to investors or automate manual processes such as fraud detection and loan underwriting.
Conclusion
The future of language AI holds endless possibilities. But we must innovate responsibly. Thoughtful research and collaboration will allow us to unlock the full potential of models like GPT-3.
Using pre-training and fine-tuning techniques, domain-specific knowledge, and unsupervised learning algorithms, researchers and developers can train models that generate high-quality and coherent text. It can understand natural language, and perform various NLP tasks accurately.
Our society stands to gain immensely from multilingual chatbots to lifesaving medical insights. Yet we cannot ignore the risks, from misinformation to biases.
So collaboration among researchers, policymakers, and stakeholders is crucial to ensure responsible and sustainable development of large language models.
Researchers can work towards making large language models more efficient, fair, and interpretable by exploring distributed training approaches, hybrid architectures, adversarial training, and bias mitigation techniques.
With careful stewardship, we can steer this technology towards creativity, compassion and the betterment of all. The path forward requires diligence. If we succeed, more powerful, equitable language models await.
But we must build them with care. The first step? Keep reading, learning, and engaging. Together we'll shape the uplifting future of AI.
Frequently Asked Questions (FAQs)
What are large language models and how do they work?
Large language models are AI-driven systems designed to understand and generate human language. They use deep learning algorithms, sophisticated language models, and massive training data.
What are the benefits and potential applications of training large language models?
Large language models can improve language translation, natural language processing, and other AI applications. They have potential use cases in healthcare, finance, and more.
What are the current limitations and challenges associated with training large language models?
Large language models require massive training data and computational resources, which can be costly. Additionally, there are concerns about fairness, bias, and privacy issues in training data.
What ethical considerations should be considered while developing and deploying large language models?
Ethical concerns include potential bias in training data, avoiding perpetuating harmful stereotypes, ensuring responsible and inclusive development, creating transparency in the models' decision-making process etc.
What advancements are expected to propel the future of large language models?
Advancements in federated learning, natural language generation and reasoning, multimodal models and collaboration among researchers, policymakers, and stakeholders are expected to boost the potential of large language models.
How can large language models contribute to the future of AI?
Large language models can be critical in advancing artificial intelligence in natural language processing, speech recognition, language translation, and other AI applications by improving accuracy, relevance, and comprehensibility.