Machine learning has become a driving force behind many technological advancements we witness today, revolutionizing various industries and shaping how we live and work. As the demand for intelligent systems and data-driven solutions grows, understanding and leveraging machine learning development in real-world scenarios has become paramount.
According to a recent report by MarketsandMarkets, the global machine learning market is expected to grow from $21.17 billion in 2022 to $209.91 billion by 2029, at a Compound Annual Growth Rate (CAGR) of 38.8% during the forecast period. This staggering growth is fueled by the increasing adoption of machine learning across diverse sectors, including healthcare, finance, retail, manufacturing, and transportation.
Machine learning development in action encompasses a wide range of practical applications transforming industries and creating new opportunities for innovation. From predictive maintenance in manufacturing facilities to personalized recommendations in e-commerce platforms, from fraud detection in financial services to disease diagnosis in healthcare, machine learning algorithms are revolutionizing decision-making processes and enabling data-driven insights.
In this blog, I will explore machine learning development in action, showcasing real-world examples and success stories from various industries. Through these practical use cases, we aim to inspire and empower readers to harness the power of machine learning and drive innovation within their respective domains.
Fraud Detection in Financial Transactions
Fraud detection is a critical concern for financial institutions worldwide. With the advancement of machine learning, detecting and preventing fraudulent activities has become more effective than ever before.
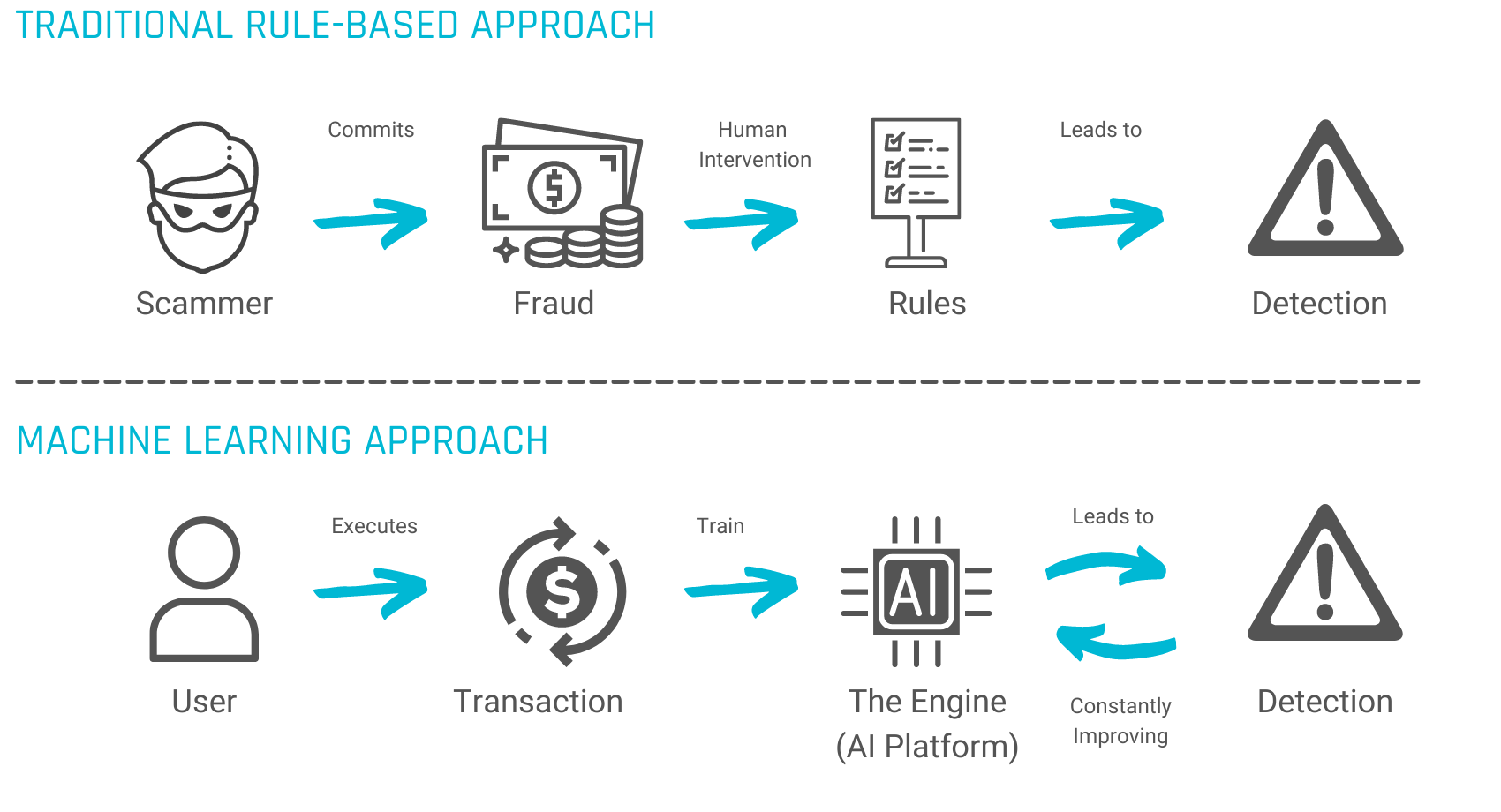
What is Fraud Detection?
Fraud detection refers to identifying and preventing fraudulent activities in financial transactions. This involves monitoring and analyzing vast amounts of data in real-time, looking for anomalous patterns or suspicious behavior.
Role of Machine Learning in Detecting Fraud
Machine learning plays a pivotal role in fraud detection. It analyzes patterns and trends in data to accurately identify fraudulent transactions. It can adapt and learn from new data, making it more effective in detecting complex and evolving fraud techniques.
Machine learning algorithms can detect fraud by examining various factors such as transaction amount, location, and customer behavior. They can identify anomalies that might indicate fraudulent activities.
For instance, if a customer's credit card is suddenly used for transactions in two different countries within a short time frame, the algorithm can flag this as potentially fraudulent behavior.
Real-world Examples and Success Stories
For example, in 2019, PayPal implemented a machine learning system to analyze its vast transaction data. This system considers purchase amount, location, device used, and historical spending habits.
The system can flag suspicious transactions in real-time by analyzing these variables and identifying deviations from a user's typical behavior, significantly reducing fraudulent activity. This approach has helped PayPal maintain a low fraud loss rate, protecting both the company and its customers.
Personalized Recommendations in E-commerce
Providing personalized customer recommendations is essential for improving user experience and driving sales.
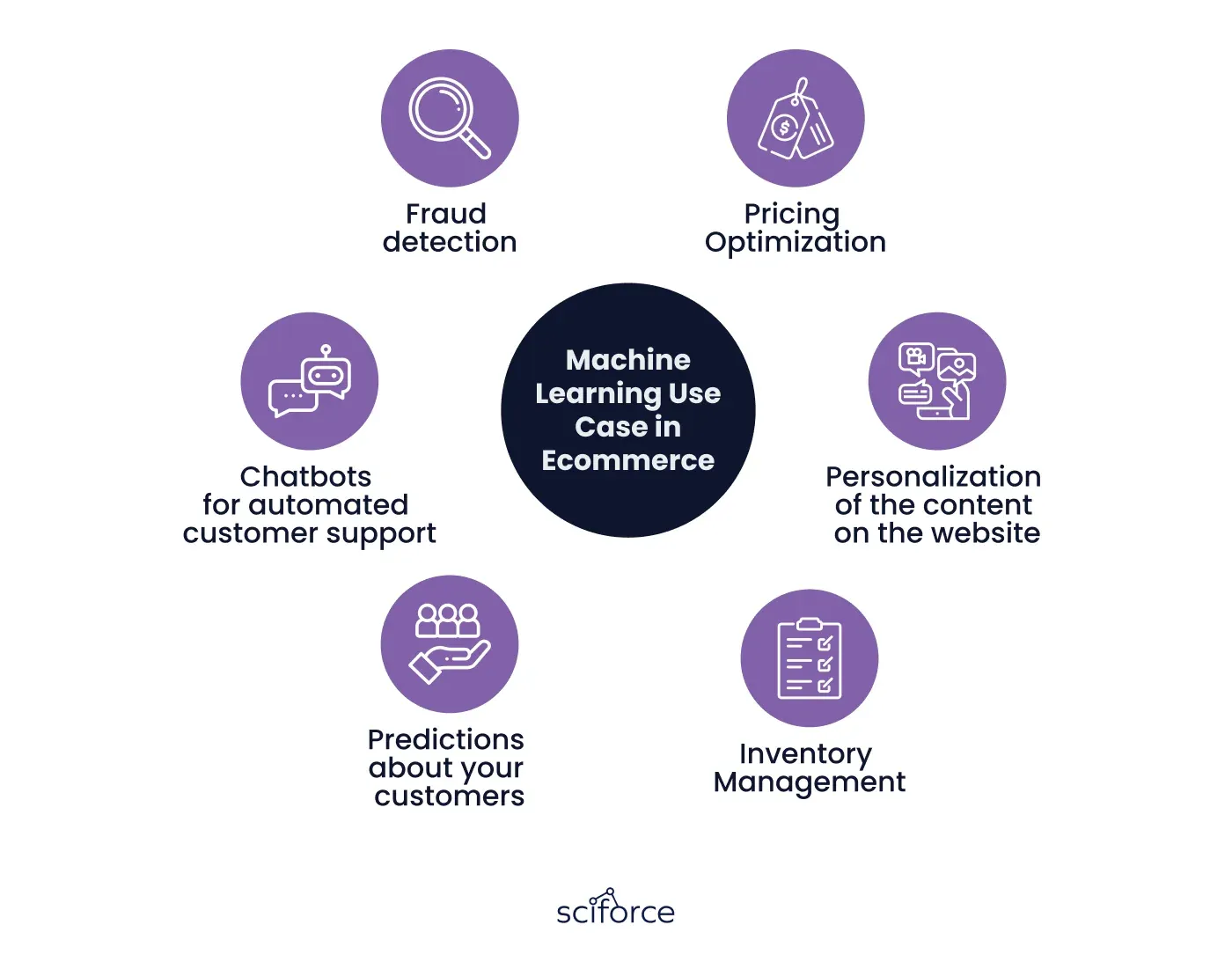
Importance of Personalized Recommendations
Personalized recommendations offer a tailored shopping experience, guiding customers toward products that align with their preferences and interests. This leads to increased customer satisfaction and, ultimately, higher conversion rates.
Role of Machine Learning in Recommendation Systems
Machine learning is at the core of developing effective recommendation systems. By analyzing customer behavior, purchase history, and preferences, machine learning algorithms can generate personalized recommendations more likely to resonate with individual customers.
These recommendation systems employ collaborative filtering techniques, content-based filtering, and hybrid approaches. Collaborative filtering analyzes the behavior and preferences of similar users to make recommendations. Content-based filtering focuses on the attributes of products and matches them with users' preferences. Hybrid approaches combine these methods for even more accurate recommendations.
Case Studies and Notable Examples
Leading e-commerce giants like Amazon and Netflix have mastered the art of personalized recommendations. Amazon's recommendation engine analyzes extensive customer data, including browsing history and purchase patterns, to suggest products customers are likely to buy. Netflix recommends relevant movies and TV shows based on user viewing history and ratings.
For example, if a customer frequently purchases skincare products, the recommendation system can suggest new skincare releases, complementary products, or popular items within that category. By personalizing recommendations, e-commerce platforms can enhance customer engagement, increase sales, and foster customer loyalty.
Challenges in Implementing Personalized Recommendations
Implementing personalized recommendations can pose challenges. One major hurdle is collecting and storing vast amounts of customer data. Organizations must ensure they have robust data management systems to handle and protect this data securely. Ensuring data privacy and building trust with customers is crucial, requiring transparency about how their data is used and offering opt-out options.
Additionally, striking the right balance between personalization and privacy is a delicate challenge that needs careful consideration. It is essential to respect customers' privacy while still providing them with relevant recommendations. Organizations must avoid being too invasive or appearing overly intrusive in their personalization efforts.
Suggested Reading:
Predictive Maintenance in Manufacturing
Predictive maintenance has gained significant importance in the manufacturing industry due to its ability to reduce downtime, optimize maintenance schedules, and save costs. Machine learning is crucial in predicting equipment failures and enabling proactive maintenance.
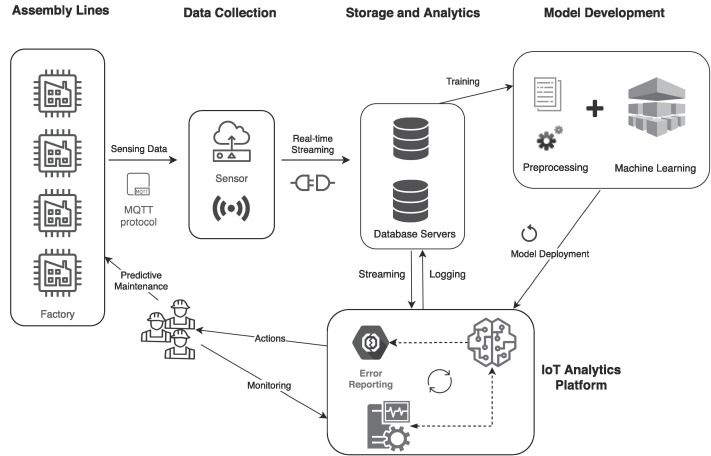
Concept and Significance of Predictive Maintenance
Predictive maintenance involves using data and analytics to predict when machinery or equipment will fail. This approach departs from traditional preventive maintenance, which relies on fixed schedules or reactive maintenance when equipment breaks down.
Role of Machine Learning in Predicting Equipment Failures: Machine learning algorithms are fundamental to predictive maintenance. They analyze large volumes of machine sensor data, looking for patterns and anomalies that indicate potential equipment failures.
Examples from Manufacturing Industries
Predictive maintenance has been successfully implemented in various manufacturing industries. For example, in the automotive sector, machine learning algorithms predict maintenance needs in production lines. Maintenance teams can proactively address potential equipment failures by monitoring real-time sensor data, minimizing downtime, and maximizing productivity.
In the energy sector, predictive maintenance is utilized in power plants to predict failures in turbines, boilers, and other critical equipment. Maintenance activities can be planned more efficiently by accurately predicting the remaining useful life of components, reducing costs, and preventing unplanned downtime.
Challenges and Considerations for Successful Implementation
Implementing predictive maintenance in manufacturing comes with specific challenges. One challenge is data quality and availability. Accurate and reliable sensor data are essential to train machine learning models effectively. Proper data collection and integration processes must be established to ensure high-quality data for analysis.
Another challenge is the complexity of the machinery and equipment involved. Different types of equipment may have distinct failure patterns, making it necessary to develop specialized machine-learning models for each type. Additionally, machines and their components may exhibit varying failure modes, requiring diverse approaches to predictive maintenance.
Suggested Reading:
Natural Language Processing in Customer Service
Natural Language Processing (NLP) revolutionizes customer service by enabling automated interaction between customers and businesses. By understanding and interpreting human language, NLP systems enhance customer experiences, improve response times, and streamline support processes.
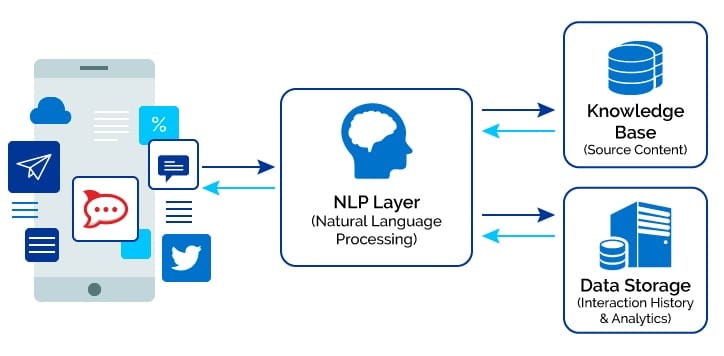
Understanding NLP
NLP is a subfield of artificial intelligence that focuses on the interaction between computers and human language. It involves techniques and algorithms that enable computers to understand, interpret, and generate human language.
NLP systems utilize various techniques, including natural language understanding (NLU) and natural language generation (NLG). NLU allows computers to comprehend and interpret human language, while NLG enables computers to generate human-like responses.
Enhancing Customer Service with NLP
NLP revolutionizes customer service by automating interactions traditionally handled by human agents. Chatbots and virtual assistants powered by NLP can understand customer queries, provide relevant information, and assist in solving problems.
NLP systems can analyze and extract valuable insights from large volumes of customer feedback, surveys, and social media posts. This enables businesses to understand customer sentiments and preferences, improving products, services, and customer experiences.
Now, if you want to begin with machine learning development to enhance customer service for your business but have no clue about how to do it, then check out the NO-CODE chatbot platform, named BotPenguin.
Real-world Applications
NLP has found widespread application in various industries, transforming customer service interactions. For example, e-commerce platforms use NLP-powered chatbots to handle customer inquiries, assist in product searches, and provide personalized recommendations.
In the banking sector, NLP systems automate routine customer service tasks, such as balance inquiries and transaction history requests. This allows human agents to focus on more complex customer needs, improving efficiency and satisfaction.
Potential Limitations and Ethical Considerations
While NLP brings significant benefits to customer service, there are potential limitations and ethical considerations to be mindful of. NLP systems may not always accurately understand the nuances of human language, leading to misinterpretation of customer queries or generating inappropriate responses.
Ensuring the privacy and security of customer data is of paramount importance. Organizations must handle and protect sensitive customer information responsibly, adhering to privacy regulations and obtaining appropriate consent for data usage.
Ethical considerations also arise in the context of NLP-powered chatbots. Transparency is key, and customers should be informed when they are interacting with an automated system rather than a human agent. Additionally, customers should always be able to escalate their queries or concerns to a human representative when necessary.
Medical Diagnosis and Treatment Planning
Machine learning has had a significant impact on healthcare, revolutionizing the field of medical diagnosis and treatment planning. By leveraging data and advanced algorithms, machine learning techniques have the potential to enhance accuracy, efficiency, and patient outcomes.
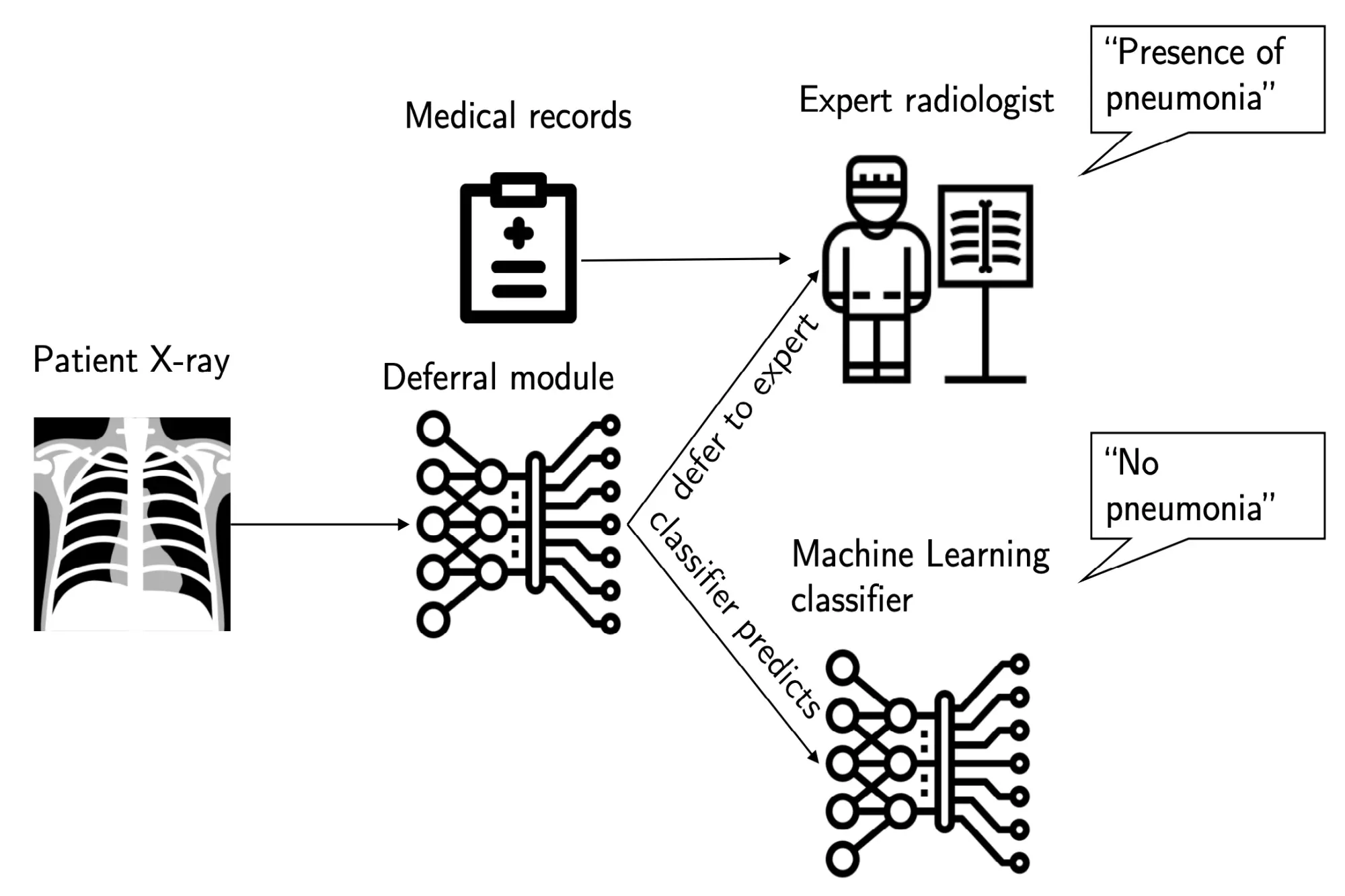
Machine Learning's Impact on Healthcare
Machine learning has opened up new avenues in healthcare by analyzing large volumes of data and extracting valuable insights. It can process complex medical information, including patient records, diagnostic images, and genomic data, to assist in diagnosis, treatment planning, and prediction of disease outcomes.
Applications in Medical Diagnosis and Treatment
Machine learning has been successfully applied to various areas of medical diagnosis and treatment planning. For example, in radiology, machine learning algorithms can analyze medical images and assist radiologists in detecting abnormalities, such as tumors or fractures. This can lead to earlier detection and more accurate diagnoses.
In personalized medicine, machine learning models can analyze an individual's genetic and clinical data to predict the likelihood of developing certain diseases or respond to specific treatments. This enables healthcare providers to personalize treatment plans, minimizing adverse effects and optimizing outcomes.
Notable Examples and Advancements
Several case studies and advancements highlight the potential of machine learning in medical diagnosis and treatment planning. For instance, researchers have developed machine learning models specifically focused on dermatology, capable of accurately diagnosing skin cancer by analyzing images of skin lesions. This technology could significantly improve early detection rates and reduce unnecessary biopsies.
Ethical and Privacy Concerns in Healthcare AI
While applying machine learning in healthcare brings numerous benefits, ethical and privacy concerns must be addressed. Patient data, including electronic health records and genomic information, are sensitive and require strict protection to ensure privacy and maintain patient trust.
There is also a need for transparency and interpretability in machine learning models used in healthcare. Understanding how these models arrive at their predictions is crucial for healthcare professionals to make informed decisions and provide appropriate care.
Additionally, bias in algorithmic decision-making must be carefully addressed to ensure fair and equitable treatment for all patients. Machine learning models should undergo rigorous testing and validation to mitigate any potential biases that could result in unequal access to healthcare services.
Conclusion
In conclusion, machine learning development in action has demonstrated its transformative potential across numerous industries, driving innovation and unlocking new opportunities for growth and efficiency. As the demand for intelligent systems and data-driven solutions continues to surge, the real-world applications of machine learning will only become more prevalent and diverse.
According to a survey conducted by Deloitte in 2022, 79% of respondents reported that their organizations have already implemented or are currently implementing machine learning technologies. Additionally, a report by the World Economic Forum estimates that by 2025, machine learning could contribute up to $15.7 trillion to the global economy.
These statistics underscore the widespread adoption and significant impact of machine learning development. As organizations continue to embrace these technologies, developers, data scientists, and decision-makers must stay abreast of the latest advancements and best practices.
By leveraging machine learning's power and staying informed about real-world use cases, businesses can gain a competitive edge, drive innovation, and unlock new opportunities for growth and success.
Suggested Reading:
Frequently Asked Questions (FAQs)
How is machine learning applied in healthcare for real-world benefits?
Machine learning in healthcare improves diagnostics, personalized treatment plans, and patient outcomes by analyzing large datasets and identifying patterns.
Can machine learning development enhance personalized experiences in marketing?
Yes, machine learning enables personalized marketing by analyzing customer behavior, predicting preferences, and optimizing targeted campaigns for better engagement and conversion rates.
How does machine learning development impact e-commerce, and what are the notable use-cases?
Machine learning enhances e-commerce through recommendation systems, fraud detection, and demand forecasting, improving user experience and operational efficiency.
What role does machine learning play in optimizing supply chain management?
Machine learning optimizes supply chain management through demand forecasting, inventory optimization, and logistics planning, improving efficiency and reducing costs.
How is machine learning transforming customer service in real-world applications?
Machine learning improves customer service by implementing chatbots, predictive support, and sentiment analysis, enhancing responsiveness and satisfaction in real-world customer interactions.