Introduction
Don't. Just stop it. You don't need to predict anything. The future is uncertain and always will be. Accepting this will set you free.
But if you insist on gazing into the crystal ball of predictive analytics, at least do it right. Use algorithms and models trained on historical data to uncover subtle patterns.
Combine statistical techniques with the elusive magic of machine learning. Yet even the most sophisticated models are clueless without high quality data.
This is where NLP comes in, allowing predictive analytics to make sense of human language in all its messy, unstructured glory. Customer reviews, social posts, financial reports - NLP transforms these into insights.
But tread carefully, lest data privacy and algorithmic bias lead you astray. The human touch remains essential, to course-correct when needed.
So continue reading as this article talks about predictive analytics and NLP’s role in forecasting trends.
What are Predictive Models?
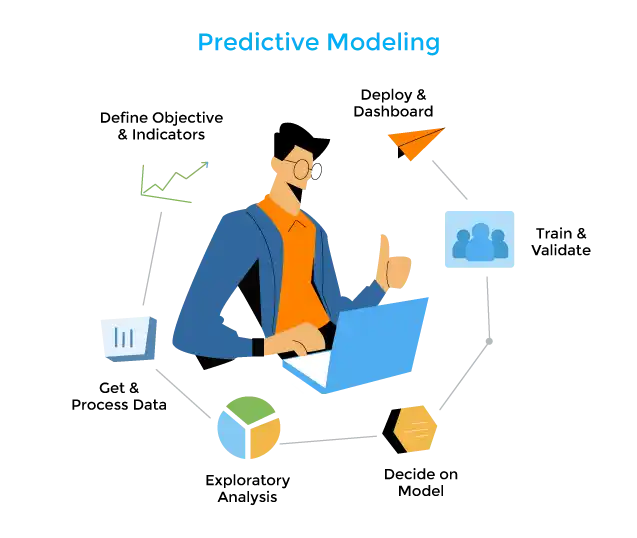
Predictive models are algorithms that are trained on historical data to make predictions about future outcomes. These models are built using statistical techniques and machine learning algorithms. They learn patterns and relationships in the data and use them to make predictions on new, unseen data.
Types of Predictive Analytics
Businesses can use several predictive analytics using NLP techniques, depending on their specific needs and use cases.
Some popular techniques include:
Regression
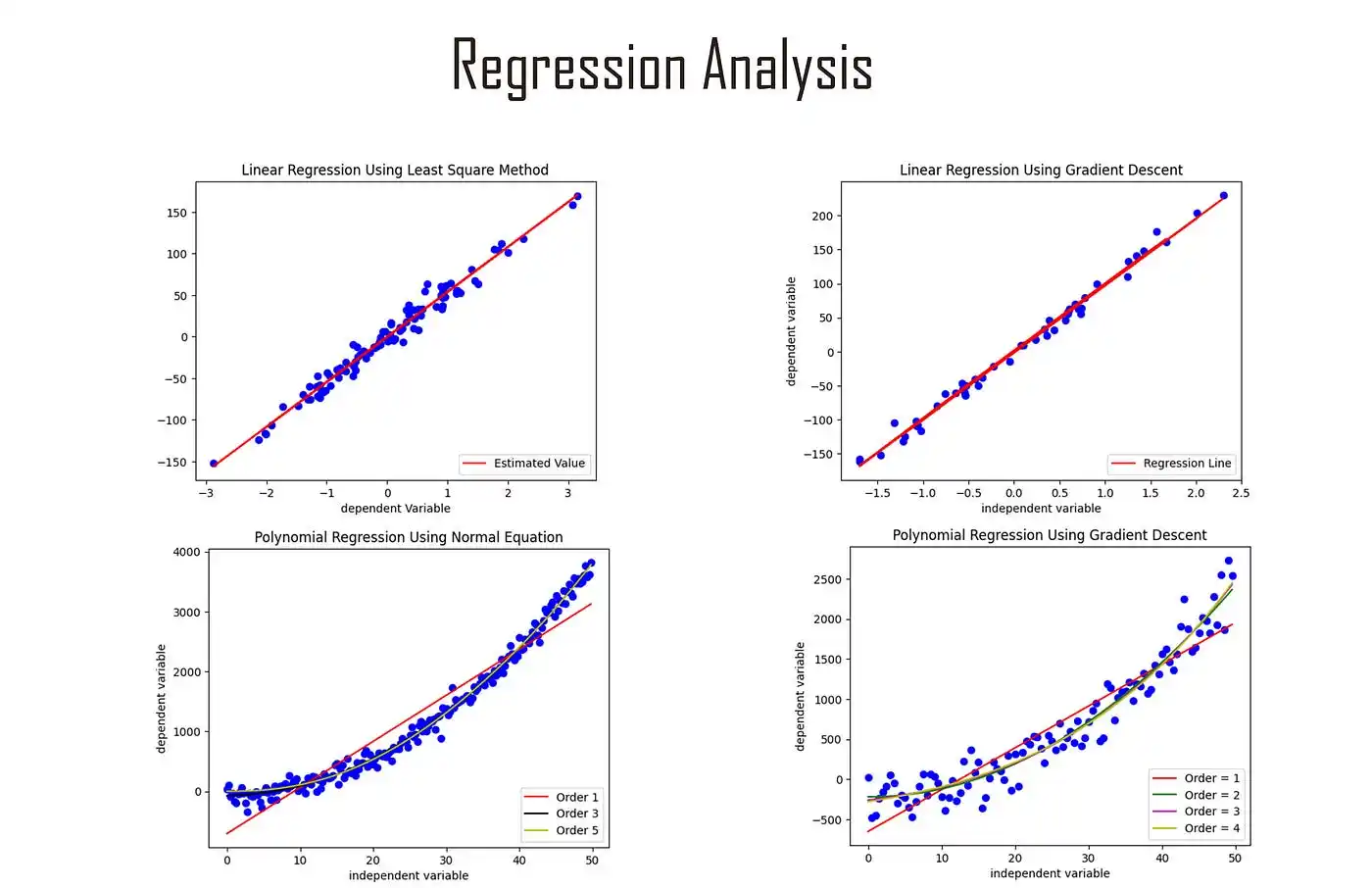
Regression analysis is a statistical technique used to predict a continuous outcome variable based on one or more predictor variables.
It helps businesses understand the relationship between the dependent and independent variables and make predictions based on that understanding.
Decision Trees
Decision trees are a popular machine learning technique used for classification and regression tasks.
They represent decisions and their possible consequences in a tree-like structure. Decision trees are intuitive and easy to interpret, which makes them a valuable tool in predictive analytics.
Neural Networks
Neural networks are a type of machine learning model inspired by the human brain. They consist of interconnected nodes, known as artificial neurons, that process and transmit information.
Neural networks are powerful tools for solving complex problems and are widely used in predictive analytics.
Cluster Analysis
Cluster analysis is a technique used to group similar data points based on their similarities or dissimilarities. It helps identify patterns and structures in data and can be particularly useful in customer segmentation and market analysis.
Applications of Predictive Analytics
Predictive analytics has a wide range of applications across industries. Here are a few examples:
Customer Segmentation
Businesses can segment their customer base into groups with similar characteristics and behaviors by analyzing customer data. This helps personalize marketing campaigns, improve customer experiences, and make targeted recommendations.
Fraud Detection
Predictive analytics using NLP can detect fraudulent activities by identifying anomalous patterns in data. This helps businesses prevent financial losses and protect their customers.
Risk Assessment
Predictive analytics can help businesses assess risks in various domains, such as finance, insurance, and healthcare. Businesses can make informed decisions and manage risks by modeling and analyzing historical data.
Demand Forecasting
Predictive analytics enables businesses to forecast demand for their products and services. This helps optimize inventory management, production planning, and supply chain operations.
Next, we will look into NLP processing.
What is NLP Processing?
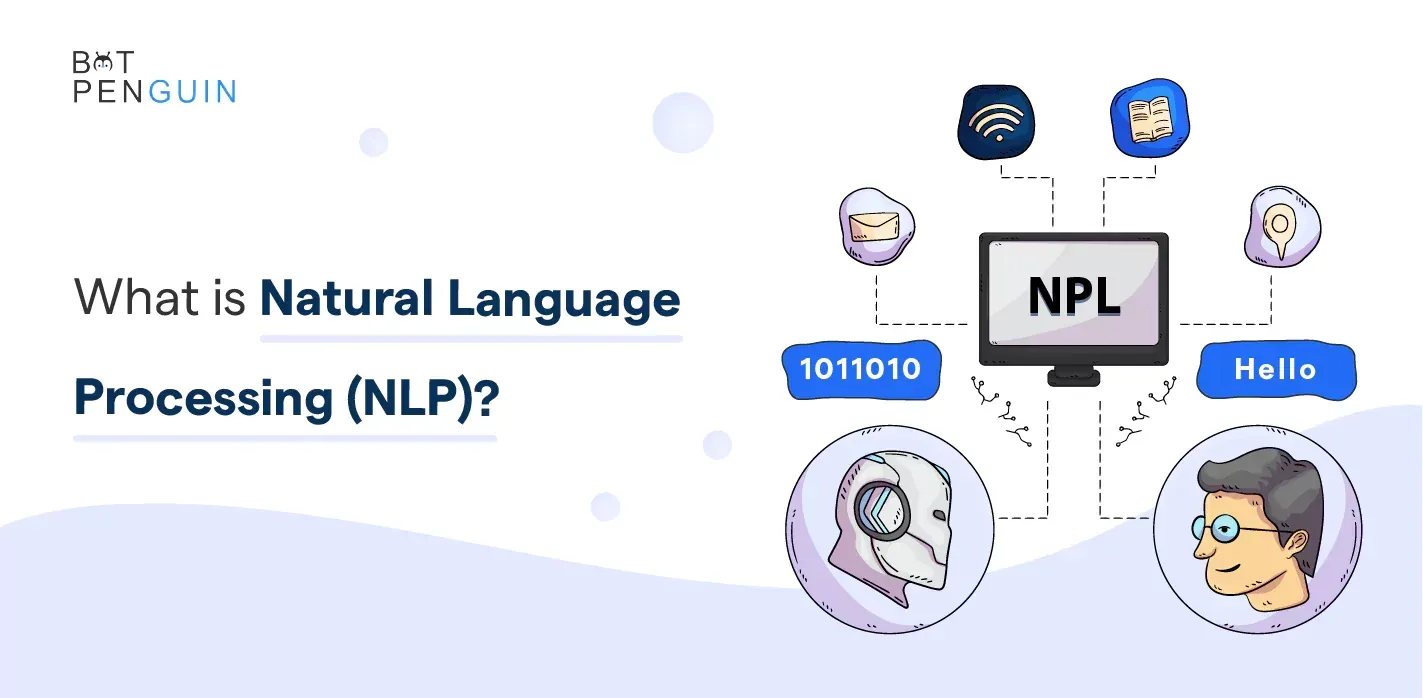
NLP focuses on bridging the gap between human language and machine understanding. It involves building algorithms and models to process and analyze text, speech, and other forms of unstructured data.
NLP techniques empower machines to extract meaning from language, automate tasks, and communicate with humans seamlessly.
Applications of NLP
NLP has numerous applications across industries and domains. Let's take a look at a few examples:
Chatbots
Chatbots are virtual assistants that engage in conversations with users. They use NLP techniques to understand user queries, provide relevant information, and assist with tasks. Chatbots, like BotPenguin, are widely used in customer support, personal assistants, and voice-controlled devices.
With BotPenguin you can easily train your chatbot on custom data, paint them with your logo and branding, and offer human-like conversational support to your customers.
And that's not it. BotPenguin makes sure that you reach your customers where they are by offering AI chatbots for multiple platforms, thus making omnichannel support look easy:
- WhatsApp Chatbot
- Facebook Chatbot
- Wordpress Chatbot
- Telegram Chatbot
- Website Chatbot
- Squarespace Chatbot
- Woocommerce Chatbot
- Instagram Chatbot
Search Engines
Search engines rely heavily on NLP to retrieve and rank web pages based on user queries. By understanding the intent behind a search query and analyzing the content of web pages, NLP helps search engines deliver relevant and accurate results.
Virtual Assistants
Virtual assistants, like Apple's Siri or Amazon's Alexa, use NLP to understand and respond to user commands or questions. They can set reminders, manage calendars, and provide information on various topics.
Social Media Analytics
NLP techniques analyze social media data, such as tweets, posts, and comments, to gain insights into customer sentiment, public opinion, and emerging trends. Social media analytics help businesses understand their audience, manage their brand reputation, and improve customer engagement.
Suggested Reading:
Tailoring User Interface Design with NLP-based Insights
How NLP Enhances Predictive Analytics
NLP brings new dimensions to predictive analytics workflows by enabling machines to understand and analyze unstructured data, such as text, voice, and images. In this section, we'll explain how NLP works with data and provide examples of how it improves predictive analytics.
Extracting insights from unstructured data
NLP can analyze unstructured data sources like customer reviews, social media posts, and support tickets to extract valuable insights into customer preferences, sentiment, and behavior. This enriched data can then be combined with structured data to develop more robust predictive models.
Improving data preprocessing
NLP techniques can help in cleaning and normalizing text data, removing noise and irrelevant information, and preparing it for further analysis. This ensures that predictive models are fed with high-quality data, leading to better predictions.
Enhancing data visualization
NLP can enrich data visualization by generating word clouds, network graphs, heat maps, and other visual representations of language-related insights. These visualizations provide intuitive and easy-to-understand ways to explore and communicate complex information.
Enabling personalized recommendations
By analyzing user behavior, preferences, and interactions with text-based content, NLP can enable personalized recommendations. This can be applied to various domains, such as product suggestions, content curation, and targeted advertisements.
Use Cases of NLP-Enabled Predictive Analytics
NLP-enabled predictive analytics has been successfully applied in various industries and domains. Let's explore some examples:
Healthcare
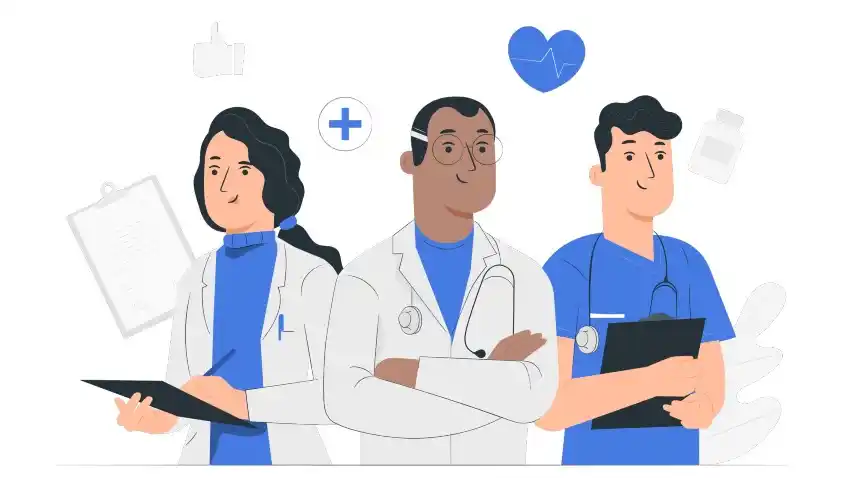
NLP predicts disease outbreaks by analyzing social media posts and online health forums. It is also deployed in diagnosing conditions by analyzing patient records and medical literature. Additionally, NLP helps monitor patient feedback in hospitals and healthcare facilities to detect areas for improvement.
Finance
NLP detects fraud by analyzing text data from financial transactions, emails, and chat logs. It also assesses credit risk by analyzing customer data and credit reports. Additionally, NLP is used for predicting market trends by analyzing news articles, social media feeds, and financial reports.
Marketing
NLP helps analyze customer feedback from surveys, reviews, and social media to identify trends, sentiments, and preferences. This helps in predicting customer churn and launching targeted marketing campaigns. NLP is also used in sentiment analysis of social media data to monitor brand reputation.
E-commerce
NLP enables personalized recommendations by analyzing user preferences, purchase history, and product reviews. It also helps predict product demand by analyzing customer interactions and market trends. Furthermore, NLP is used in improving the customer experience through chatbots and virtual assistants.
Challenges and Risks of NLP-Enabled Predictive Analytics
While NLP-enabled predictive analytics has numerous benefits, there are also some potential drawbacks and risks to consider. Here are some of the drawbacks:
Data Privacy and Security Concerns
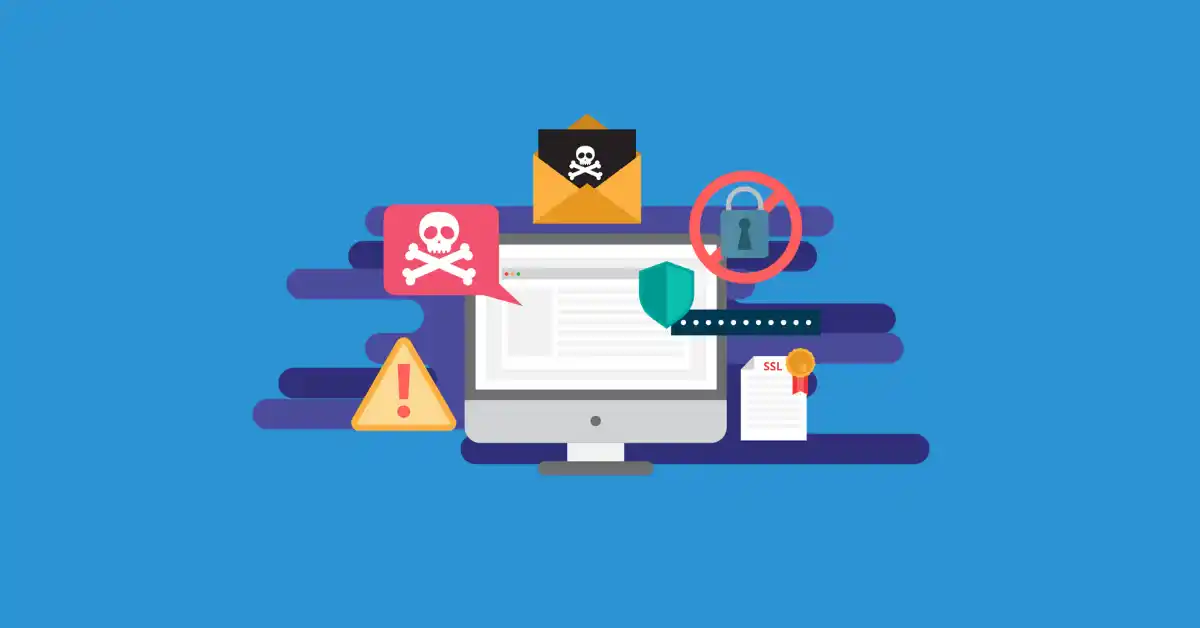
NLP-enabled predictive analytics relies on massive amounts of data, often containing sensitive and personal information. Companies must ensure security and privacy by obtaining informed consent from users, anonymizing data when possible, and protecting against cyber-attacks.
Algorithmic Bias and Discrimination
Predictive analytics using NLP systems can be biased towards specific groups or demographics, leading to discriminatory outcomes. Addressing algorithmic bias requires additional efforts, such as evaluating models against fairness criteria and implementing corrective measures.
Lack of Human Oversight and Control
NLP-enabled predictive analytics systems can sometimes operate with limited human oversight and control, leading to unintended consequences. To address this, companies must establish processes for human review to ensure high-quality and responsible outcomes.
Misinterpretation and Miscommunication of the Results
NLP-enabled predictive analytics systems can sometimes produce complex and difficult-to-interpret results. To ensure effective communication and understanding of the results, companies must consider the intended audience and design visualizations and explanations accordingly.
Conclusion
In conclusion, predictive analytics and NLP play crucial roles in forecasting trends.
With natural language processing, businesses can extract valuable insights from unstructured data. As it would enable them to anticipate market shifts and consumer behavior with greater accuracy.
NLP techniques facilitate the analysis of vast amounts of text data. It allows organizations to uncover patterns, sentiments, and emerging themes that might otherwise remain hidden.
Moreover, the integration of predictive analytics and NLP empowers companies to make informed decisions and stay ahead of the competition in dynamic industries.
By leveraging advanced algorithms and machine learning models, businesses can generate forecasts and predictions based on linguistic cues, social media trends, and other textual sources. This enables proactive strategies, such as targeted marketing campaigns, product innovations, and risk management initiatives.
Furthermore, the continuous advancements in both predictive analytics and NLP technologies promise even more sophisticated capabilities in trend forecasting. As algorithms become more refined and data processing techniques evolve, organizations can expect to achieve higher levels of accuracy and efficiency in predicting future trends.
Overall, the synergy between predictive analytics and NLP represents a powerful force in driving business success and staying responsive to market dynamics.
Suggested Reading:
Top 5 languages for natural language processing
Frequently Asked Questions (FAQs)
How does natural language processing (NLP) contribute to predictive analytics?
NLP plays a crucial role in predictive analytics by enabling analysis of unstructured text data. It helps extract insights and patterns from text, allowing for better forecasting of trends and making more accurate predictions.
What types of text data can NLP analyze in predictive analytics?
In predictive analytics, NLP can analyze various text data types, such as social media posts, customer reviews, surveys, news articles, emails, and other textual sources. It helps uncover valuable insights hidden in these texts.
How can NLP improve trend forecasting accuracy?
NLP enhances trend forecasting accuracy by extracting key information from large volumes of text data. By analyzing sentiments, opinions, and contextual information, it provides a more comprehensive understanding of trends, leading to more accurate predictions.
Can NLP help identify emerging trends before they become mainstream?
Yes, Predictive Analytics using NLP can help identify emerging trends before they become mainstream. By monitoring social media discussions, online forums, and news articles, NLP can detect early signals and patterns, enabling businesses to stay ahead of the curve.
What are the limitations of using NLP in predictive analytics for trend forecasting?
While NLP is powerful in identifying trends, it has limitations. These include biases in the training data, challenges in understanding sarcasm or irony, and difficulty in handling context-dependent language, which might affect the accuracy of predictions.