What is Regression Analysis?
At its heart, Regression Analysis is a statistical technique that explores the relationship between a dependent variable (what you're trying to predict) and one or more independent variables (what you think will influence the prediction).
Understanding the Basics
Imagine you run a shop. You want to figure out how your daily sales are affected by factors like the day of the week, the weather, and the price per cup. Regression analysis helps quantify just
The Role of Data
Good data is key. The quality and quantity of your data directly impact the reliability of your regression analysis. Garbage in, garbage out, as they say.
Calculations and Coefficients
Through a series of calculations, regression analysis provides coefficients, which are values that tell us about the strength and direction of relationships between our variables.
The Goal
Ultimately, regression analysis is all about making predictions. By understanding relationships, we can make well-informed guesses about future outcomes.
Why Use Regression Analysis?
Why bother with regression? Well, because it gives clarity about the connections within your data, which is crucial for informed decision-making.
Predictive Power
Want to know what's likely going to happen tomorrow, next month, or next year? Regression analysis helps you peer into the future by making educated predictions based on past and present data.
Understanding Relationships
It tells you not just that things are related, but how closely they're related. You get to understand which factors matter most and which you can ignore.
Decision Making
Armed with insights from regression analysis, businesses and researchers can make smarter, data-driven decisions.
Identifying Trends
Regression helps in spotting trends over time. It's like having a time machine that doesn't break the laws of physics.
Quantifying Effects
Imagine knowing exactly how much sales would jump if you lowered your prices. Regression analysis can give you that level of detail.
How Does Regression Analysis Work?
Let's break down the magic behind the curtain, showing how regression analysis turns raw data into insights.
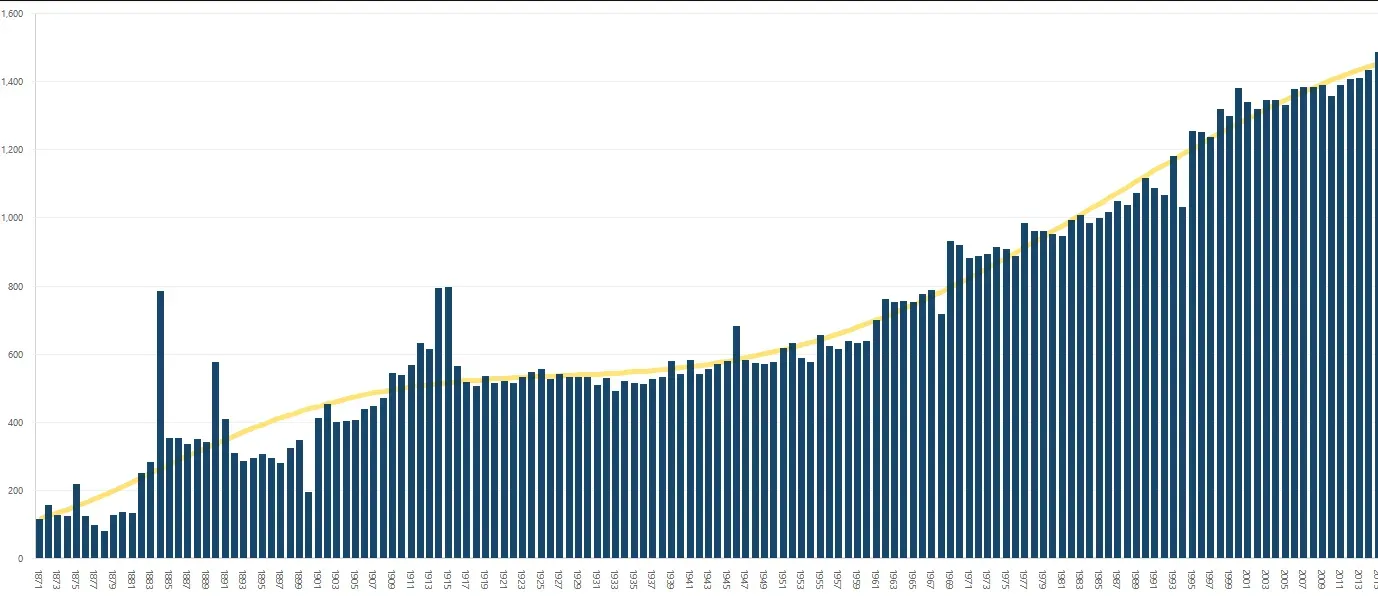
Data Collection and Preparation
First off, you gather and clean your data. This step is about making sure the data is accurate, complete, and relevant.
Model Selection
Next, you choose the right regression model based on your data and what you're trying to predict. It's like selecting the right lens to view your data through.
Assumption Checking
Regression comes with certain assumptions (like a linear relationship between variables). You need to check these assumptions to ensure your analysis stands on solid ground.
Estimation of Coefficients
This is where the computational heavy lifting happens. Using statistical software, the model estimates the coefficients that best fit your data.
Model Evaluation
Finally, you evaluate the model's performance by checking how well it predicts outcomes on new, unseen data. It's a bit like a dress rehearsal before opening night.
When Should You Use Regression Analysis?
Timing and context are key. Here are scenarios where regression analysis shines bright.
Exploring New Markets
Before plunging into a new market, regression can help predict potential success based on factors like market size and demographics.
Product Pricing Strategies
Pondering over pricing? Regression can quantify how different price points might affect sales.
Demand Forecasting
Businesses use regression to estimate future demand for their products, helping in planning and resource allocation.
Risk Management
In finance, regression helps in assessing risk and return profiles, aiding in investment decisions.
Healthcare Predictions
From predicting disease outbreaks to patient recovery times, regression is a vital tool in healthcare analytics.
Who Provides Regression Analysis?
Know who's likely to wield this statistical sword.
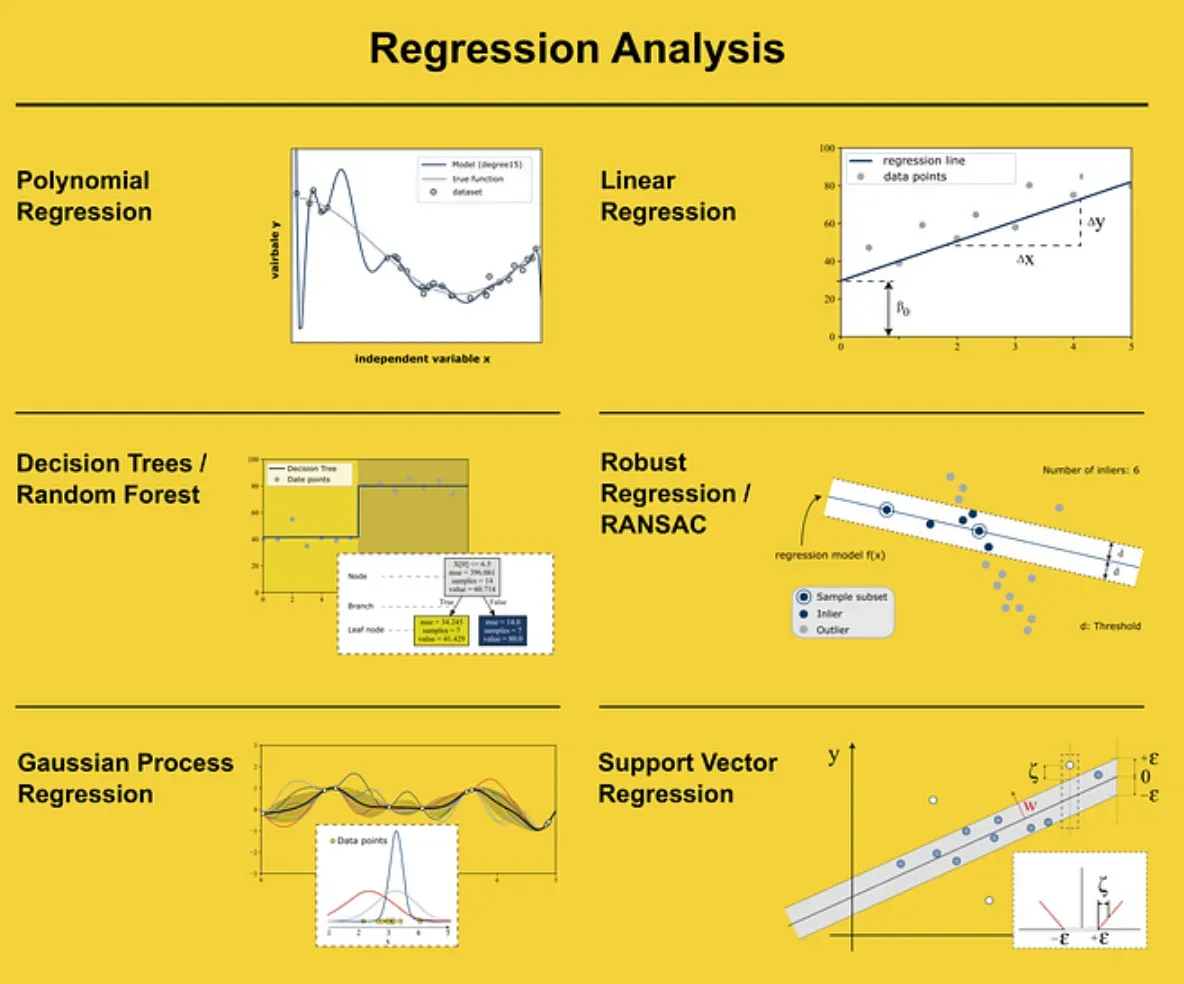
Data Scientists
These folks are the ninjas of data, often found using regression analysis to solve complex problems across various domains.
Statisticians
The original gangsters of the regression analysis world, statisticians use this method across a plethora of research fields.
Business Analysts
They apply regression to understand market dynamics, optimize operations, and enhance business strategies.
Economists
Economists use regression to analyze and predict economic outcomes based on historical data.
Academic Researchers
Whether it's psychology, sociology, or environmental science, researchers use regression to test hypotheses and validate theories.
Where is Regression Analysis Used?
Regression analysis isn't picky about where it lends a hand. Let's explore its hangout spots.
Business and Finance
From predicting stock prices to customer lifetime value, regression is the go-to tool for many business and financial analysis tasks.
Healthcare
In the realm of medicine, regression helps in predicting patient outcomes, understanding disease progression, and more.
Education
Educators and policymakers use regression to understand factors influencing student performance and educational quality.
Environmental Science
Here, regression predicts things like pollution levels and the impact of human activities on climate change.
Sports Analytics
Even in sports, regression is used to predict game outcomes, player performance, and to evaluate strategies.
Best Practices in Regression Analysis
To wield regression analysis effectively, it helps to keep some best practices in mind.
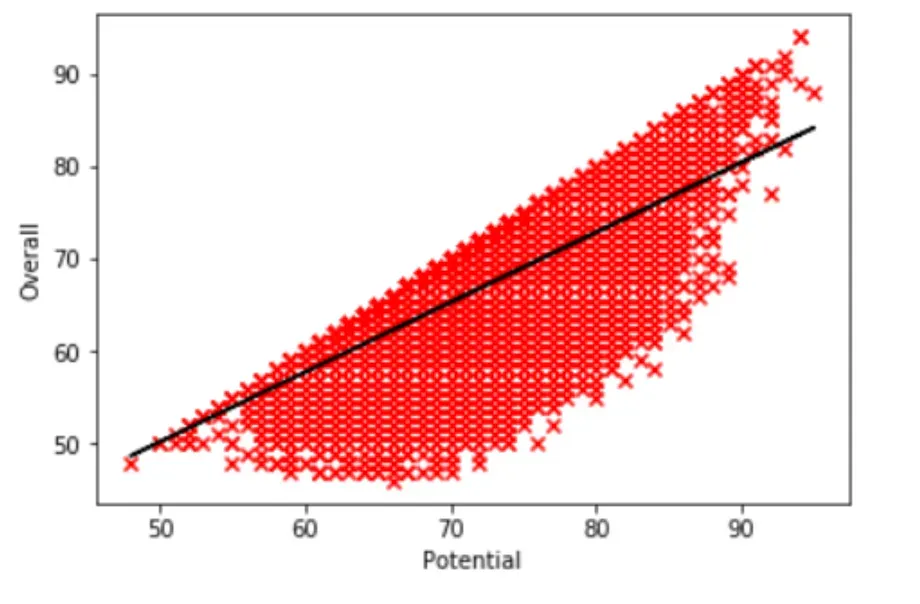
Understand Your Data
Knowing your dataset inside out isn’t just good practice; it’s essential. This familiarity can help in choosing the right model and making accurate predictions.
Choose the Correct Model
Not all data sets are created equal. Selecting the appropriate regression model is crucial for obtaining meaningful results.
Don’t Ignore Assumptions
Regression models come with underlying assumptions. Ignoring these can lead to incorrect conclusions.
Validate Your Model
Always test your model on a separate dataset to ensure its validity. It's like proofreading your work before submission.
Interpret with Caution
Regression coefficients and predictions provide a wealth of information. However, correlation doesn’t imply causation, and external factors not included in your model can influence results.
Challenges in Regression Analysis
Despite its utility, regression analysis is not without its hurdles.
Data Quality
Poor quality data can lead to misleading results. Ensuring data accuracy and completeness is a constant challenge.
Choosing the Right Model
With an array of regression models available, selecting the most appropriate one can be daunting.
Multicollinearity
When independent variables in a regression model are correlated, it can muddy the waters, making it hard to discern their individual effects.
Overfitting
Creating a model that too closely fits the data can make it perform poorly on new, unseen data. It's like memorizing answers without understanding the concepts.
Interpretation Mistakes
Misinterpreting regression outputs can lead to incorrect conclusions. This is where a solid understanding of both the domain and statistical principles is crucial.
Recent Trends in Regression Analysis
The field of regression analysis is not static. New trends shape its application and development.
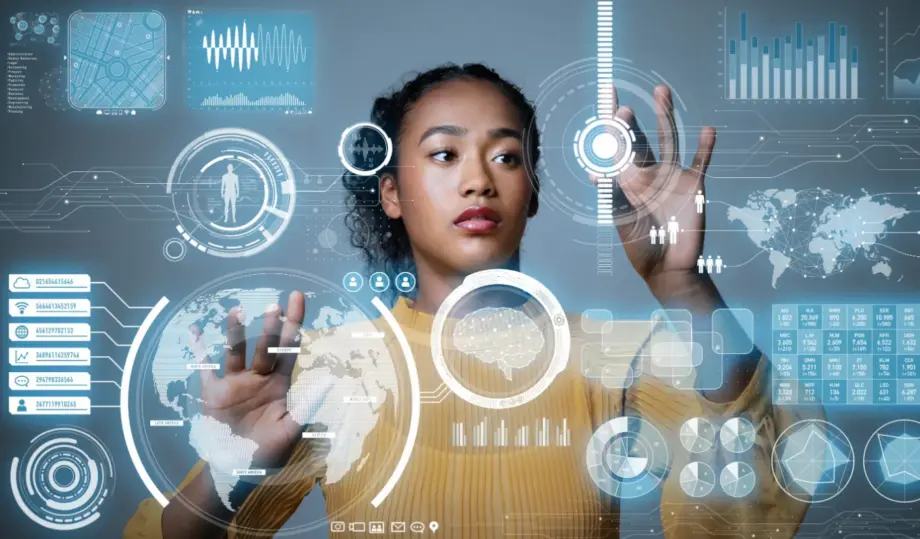
Machine Learning Integration
Machine learning models are being combined with traditional regression techniques to enhance prediction accuracy and handle complex datasets.
Big Data
The advent of big data has expanded the scope and scale of regression analysis, allowing for more detailed and comprehensive studies.
Advances in Software
Improvements in statistical software make regression analysis more accessible and powerful, broadening its user base.
Focus on Interpretability
As complex models become more common, there’s a growing emphasis on making their outputs understandable and actionable.
Cross-disciplinary Applications
Regression analysis is venturing beyond its traditional realms, finding uses in fields like genomics, cybersecurity, and even in the analysis of social media trends.
Regression analysis stands as a cornerstone of statistical analysis and data science, bridging the gap between data and decision-making. Its versatility and power make it an invaluable tool across a wide range of disciplines and industries. Like any tool, though, its effectiveness depends not just on the technique itself but on the skill and understanding of the person wielding it.
Frequently Asked Questions (FAQs)
How does Regression Analysis help in Prediction?
Regression analysis estimates relationships between variables, allowing predictions for one variable based on the known values of others. It's central in forecasting scenarios like sales based on advertising spend.
Can Regression Analysis Determine Cause and Effect?
While regression can identify correlations, it cannot conclusively establish causality without further experimental validation. Other influencing factors might not be accounted for.
What's the difference between Linear and Nonlinear Regression?
Linear regression predicts outcomes with a straight-line relationship between variables, while nonlinear regression is used when the relationship involves curves or more complex mathematical functions.
Why is Overfitting a Concern in Regression Analysis?
Overfitting occurs when a model is too complex, capturing noise in the data instead of the underlying pattern. This leads to poor performance on new, unseen data.
How important are assumptions in Regression Analysis?
Assumptions in regression are crucial; violations, such as non-linearity, autocorrelation, or heteroscedasticity, can lead to biased estimates, making careful diagnostics and corrections important for reliable results.