What is Visual Recognition?
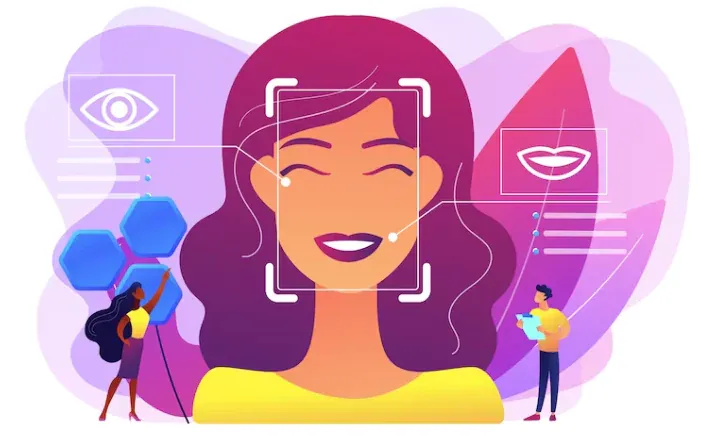
Visual recognition empowers computers to identify and understand objects, scenes, and activities within images and videos. It's like gifting your computer a pair of high-tech glasses that allows it to see and make sense of the world.
Types of Visual Recognition Technologies
Various types of visual recognition technologies exist, including image classification, object detection, facial recognition, and scene understanding. Each technology focuses on a specific aspect of visual recognition, making computers smarter and more perceptive.
Applications and Use Cases
Visual recognition has oodles of applications, from tagging your friends in social media photos to helping autonomous cars navigate safely. Other use cases include medical imaging, retail analytics, and even wildlife conservation.
Why is Visual Recognition Important?
Advantages of Visual Recognition
Visual recognition offers several perks, such as automating tasks, improving accuracy, and enhancing user experiences. It's like having a tireless and detail-oriented assistant that can analyze images and videos with incredible precision.
Industries Benefiting from Visual Recognition
Visual recognition is revolutionizing various industries, including healthcare, retail, manufacturing, and security. It's like a Swiss Army knife that can be adapted and customized to meet the unique needs of different sectors.
Impact on Human-Computer Interaction
Visual recognition is transforming how we interact with computers, making them more intuitive, engaging, and accessible. It's like teaching computers a new language, allowing them to understand and respond to visual cues just like humans.
How does Visual Recognition work?
Image Processing and Feature Extraction
Visual recognition starts with image processing and feature extraction, which involves breaking down an image into its essential elements and identifying key features. It's like giving your computer a magnifying glass to examine and analyze images in detail.
Machine Learning and Deep Learning Techniques
Machine learning and deep learning techniques are the backbone of visual recognition, enabling computers to learn from experience and improve their performance over time. It's like training your computer to become an expert in recognizing patterns and making predictions.
Training and Evaluation of Visual Recognition Models
Visual recognition models are trained using large datasets of labeled images and evaluated based on their accuracy and performance. It's like sending your computer to school, where it learns from examples and gets graded on its ability to recognize visual content.
Visual Recognition Algorithms and Techniques
Convolutional Neural Networks (CNNs)
Convolutional Neural Networks (CNNs) are a type of deep learning algorithm specifically designed for visual recognition tasks. They're like the brain's visual cortex, enabling computers to process and understand images in a hierarchical and efficient manner.
Object Detection Algorithms
Object detection algorithms, such as YOLO and Faster R-CNN, help computers identify and locate objects within images and videos. It's like playing a game of "Where's Waldo?" with your computer, where it learns to spot and track objects of interest.
Image Classification Techniques
Image classification techniques, such as k-Nearest Neighbors and Support Vector Machines, enable computers to categorize images based on their content. It's like teaching your computer to sort and organize a photo album based on the pictures' subjects.
Popular Visual Recognition Tools and Platforms
Open-Source Libraries and Frameworks
Numerous open-source libraries and frameworks, such as TensorFlow and OpenCV, provide tools and resources for developing visual recognition applications. They're like DIY kits that help you build and customize your own visual recognition solutions.
Commercial Visual Recognition Solutions
Commercial visual recognition solutions, such as IBM Watson Visual Recognition and Clarifai, offer pre-built models and APIs for various use cases. They're like ready-to-use solutions that can be easily integrated into your applications.
Cloud-Based Visual Recognition Services
Cloud-based visual recognition services, such as Amazon Rekognition and Google Cloud Vision, provide scalable and cost-effective solutions for processing and analyzing large volumes of visual data. They're like powerful remote servers that can handle your visual recognition needs on-demand.
Implementing Visual Recognition in Applications
Integration with Existing Systems
Visual recognition can be integrated with existing systems and applications, providing new capabilities and enhancing user experiences. It's like adding a new set of skills to your computer's repertoire, making it more versatile and powerful.
Design Considerations and Best Practices
When implementing visual recognition in applications, it's essential to consider factors such as user experience, performance, and accessibility. Following best practices and guidelines can help ensure a successful and delightful visual recognition experience.
Performance Optimization and Scalability
Optimizing the performance and scalability of visual recognition applications is crucial for handling large volumes of data and ensuring a smooth user experience. It's like fine-tuning your computer's engine, making it more efficient and powerful.
Privacy and Security in Visual Recognition
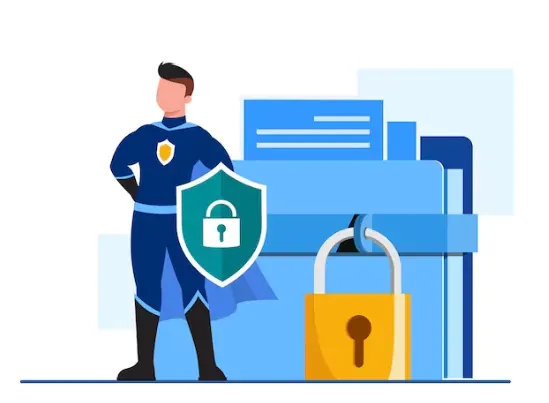
Data Collection and Storage
Visual recognition often involves collecting and storing sensitive visual data, such as images and videos. Ensuring the privacy and security of this data is essential for building trust and maintaining compliance with regulations.
User Consent and Control
Users should be informed about how their visual data is being used and have the option to opt-out of data collection or delete their data. Providing users with control over their information is crucial for respecting their privacy and autonomy.
Mitigating Risks and Ensuring Security
Implementing robust security measures, such as encryption and secure authentication, can help protect visual data and maintain privacy. Regularly updating and monitoring visual recognition applications can also help identify and address potential vulnerabilities.
Challenges and Limitations of Visual Recognition
Accuracy and Robustness
While visual recognition has made significant advancements, challenges related to accuracy and robustness still exist, especially when dealing with complex scenes, varying lighting conditions, and occlusions. It's like teaching your computer to recognize objects even when they're partially hidden or in the shadows.
Computational Complexity and Resource Requirements
Visual recognition can be computationally intensive and require significant resources, especially for large-scale applications and real-time processing. Balancing performance and resource requirements is essential for ensuring a smooth and responsive user experience.
Bias and Fairness in Visual Recognition
Visual recognition algorithms can sometimes exhibit bias and unfairness, particularly when trained on unbalanced or biased datasets. Addressing these issues is crucial for ensuring that visual recognition applications are fair and unbiased.
Future of Visual Recognition
Emerging Trends and Innovations
- Deep Learning: A subset of machine learning that uses artificial neural networks to enable computers to learn from large amounts of data, making it a driving force behind the advancements in visual recognition.
- Convolutional Neural Networks (CNNs): A type of deep learning architecture specifically designed for image recognition tasks, enabling computers to recognize objects, scenes, and even facial expressions.
- Generative Adversarial Networks (GANs): A type of deep learning model that generates new images by pitting two neural networks against each other, with one network generating images and the other evaluating them. GANs have the potential to revolutionize visual recognition by creating more diverse and realistic training data.
- Transfer Learning: A technique that allows a pre-trained neural network to be fine-tuned for a new task, reducing the amount of training data and time required for visual recognition tasks.
- Edge Computing: The practice of processing data closer to the source, such as on a smartphone or IoT device, instead of sending it to a centralized server. This trend has the potential to make visual recognition more efficient and accessible, especially in real-time applications.
Potential Applications and Opportunities
- Autonomous Vehicles: Visual recognition is a key component of self-driving cars, enabling them to perceive and understand their environment in order to navigate safely.
- Smart Cities: Visual recognition can be used to monitor traffic, optimize public transportation, and enhance public safety through intelligent surveillance systems.
- Healthcare: From diagnosing diseases based on medical images to assisting surgeons during operations, visual recognition has the potential to revolutionize healthcare.
- Retail: Visual recognition can be used to create personalized shopping experiences, automate inventory management, and even prevent shoplifting through smart surveillance systems.
- Agriculture: Farmers can use visual recognition to monitor crop health, identify pests, and optimize irrigation, leading to increased efficiency and sustainability.
Ethical Considerations and Implications
- Privacy: As visual recognition becomes more widespread, concerns about privacy and surveillance will need to be addressed, especially in public spaces.
- Bias: Visual recognition models can perpetuate existing biases if they are trained on biased data, leading to unfair or discriminatory outcomes.
- Job Displacement: As visual recognition automates tasks previously performed by humans, there may be concerns about job displacement and the need for re-skilling.
- Transparency and Accountability: Ensuring that visual recognition systems are transparent and accountable is essential for building trust and preventing misuse.
Frequently Asked Questions
How does visual recognition technology work?
Visual recognition technology utilizes deep learning algorithms to analyze and interpret images or videos. It identifies objects, scenes, faces, and other visual elements by comparing them to a database of known patterns.
What are the applications of visual recognition?
Visual recognition has various applications, including facial recognition for authentication, object recognition for e-commerce, content moderation for social media platforms, and image search for finding similar images online.
Can visual recognition detect emotions?
Yes, visual recognition can detect emotions by analyzing facial expressions, body language, and other visual cues. It can identify emotions like happiness, sadness, anger, surprise, and more, enabling applications in sentiment analysis and user experience optimization.
Is visual recognition technology accurate?
Visual recognition technology has achieved significant accuracy levels in recent years, but it may still have limitations. Accuracy depends on factors such as the quality of the input data, training data diversity, and the complexity of the recognition task.
How can visual recognition benefit businesses?
Visual recognition can benefit businesses in various ways. It enables automated image analysis, streamlines processes like inventory management and quality control, enhances customer experiences, and enables personalized marketing based on visual data.