What is Unstructured Data?
Unstructured data refers to data that does not fit neatly into traditional rows and columns found in relational databases or spreadsheets. It is typically text-heavy but may also contain data like dates, numbers, and facts. Some common examples include audio recordings, video content, social media posts, emails, web pages, reports, images, and many more. According to IDC, around 80% of the world's data will be unstructured by 2025, emphasizing the significance and ubiquity of such data.
Who Generates Unstructured Data?
As a matter of fact, virtually everyone generates unstructured data. Every time a person writes an email, records a video, posts on a social media platform, or takes a picture with their phone, they create unstructured data. Companies also generate massive amounts of unstructured data through customer interactions, transaction records, and documents. Moreover, machines and devices connected to the internet, generating logs and sensor data, also create enormous amounts of unstructured data.
When is Unstructured Data Created?
Given the digital age we're living in, unstructured data is being created constantly. Every second of every day, unstructured data is created through various sources. As long as individuals interact online, companies conduct business, or connected machines operate, unstructured data will continuously be generated.
Where is Unstructured Data Stored & Processed?
Unstructured data can be stored in a plethora of locations. These can range from traditional databases to modern distributed data storage systems. Most commonly, it's stored in NoSQL databases, data lakes, or object storage. Processing unstructured data, however, often requires advanced tools and technologies. Techniques like text analytics, natural language processing (NLP), machine learning (ML), and artificial intelligence (AI) are frequently employed to extract valuable insights from unstructured data.
Why is Unstructured Data Important?
The importance of unstructured data lies in the value it holds. While it's more challenging to analyze, the insights that can be gleaned from it are often deeper and more informative compared to structured data. Unstructured data can reveal patterns, correlations, and customer sentiments that might otherwise go unnoticed. Companies are increasingly realizing the potential of unstructured data as a source of competitive advantage and are investing in technologies to harness its power.
When effectively managed and processed, unstructured data can provide invaluable insights, contribute to strategic decisions, and fuel AI algorithms, unlocking a wealth of opportunities for businesses and organizations.
How to Manage Unstructured Data?
Here are some key ways to effectively manage unstructured data:
- Categorize and tag data - Add metadata like tags and descriptors to unstructured content to make it more findable and organized. Facilitate discovery by taxonomy and ensure consistent vocabulary.
- Centralize storage - Store unstructured data assets in a consolidated data lake, cloud storage or purpose-built repositories rather than siloed across systems. Makes accessing and analyzing data easier.
- Establish governance - Define policies for data retention, access permissions, security, compliance and lifecycle management. Important for maintaining control over decentralized data.
- Leverage AI for insights - Use AI techniques like NLP, image recognition and ML-powered analytics to extract value from unstructured data at scale. Helps deal with sheer data volumes.
- Modernize processing - Implement big data platforms like Hadoop and Spark for scalable processing of unstructured data vs traditional relational databases. Enables real-time analysis.
- Create unified views - Aggregate and connect structured and unstructured data sources through data virtualization. Get a single unified view for easy access to all information.
- Automate workflows - Automate repetitive processes like data ingestion, classification, quality checks and more for operational efficiency. Minimizes manual overhead.
- Maintain data quality - Use validation, error checking, deduplication and cleansing to ensure quality of ingested unstructured data. Bad data leads to poor analytics.
- Monitor and report - Track usage, trends and metrics around unstructured data platforms. Helps identify issues and optimize performance.
- Modernize skills - Train and hire data scientists, analysts and engineers skilled in newer unstructured data technologies and techniques. Key for long-term success.
Types of Unstructured Data
In this section, we will explore various types of unstructured data, a kind of data that does not adhere to a predefined model or is not organized in a predefined manner.
Textual Data
Textual data is one of the most prevalent types of unstructured data. This includes emails, documents, social media posts, and web content. While rich in information, its lack of structure can make extracting insights challenging.
Audio Data
This refers to sound or speech data. From voice recordings to podcasts, and even to music files, audio is a substantial part of unstructured data that requires specialized techniques (like speech recognition and processing) to analyze.
Video Data
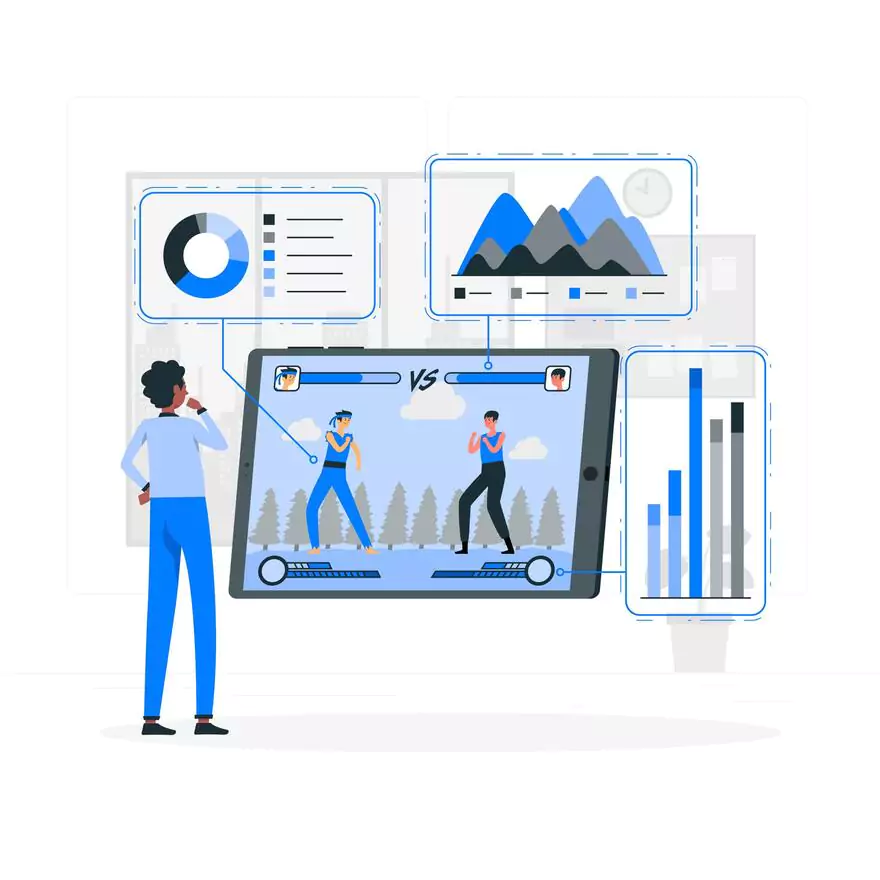
Increasingly essential in our digital age, video data is complex given its combination of visual and audio content. It can range from surveillance footage to online streaming videos, and requires advanced tools (like computer vision and deep learning techniques) for proper analysis.
Image Data
Image data includes any digital representation of visual information. Medical scans, photographs, graphs, etc., fall into this category. Techniques such as image recognition and object detection are often used to extract valuable information from image data.
Social Media Data
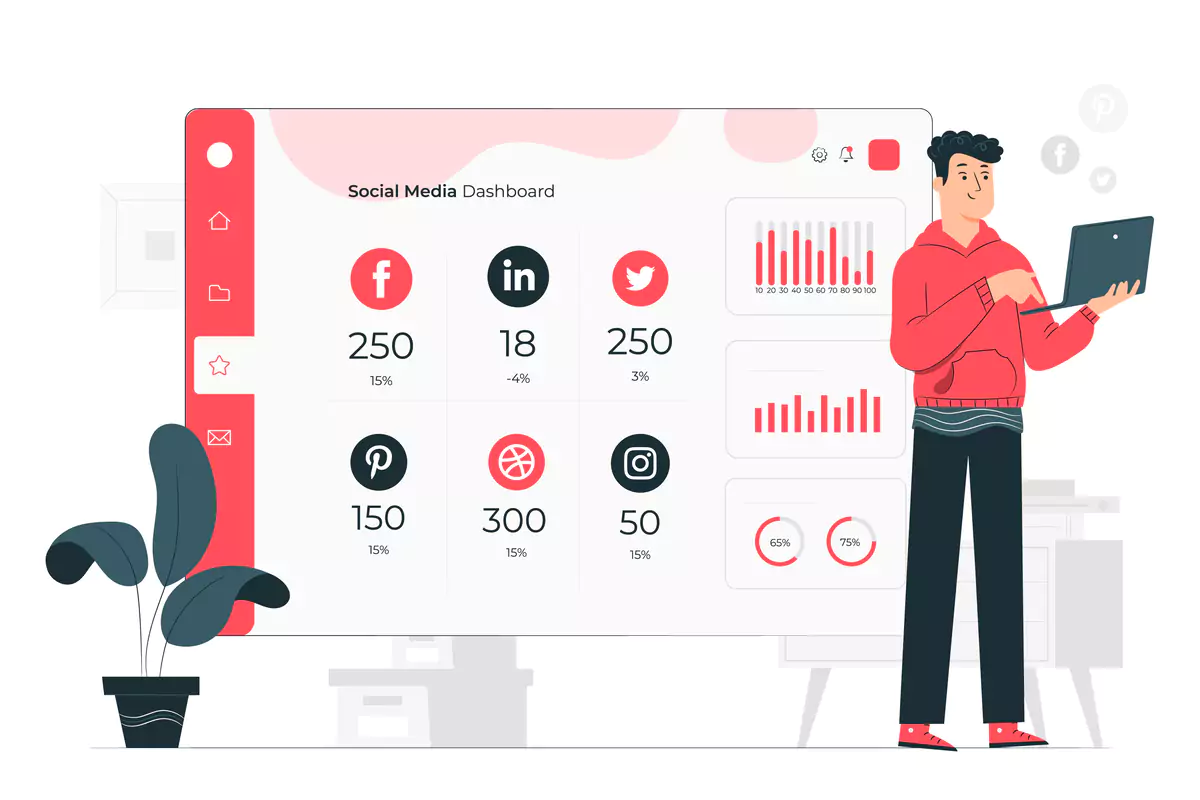
This is a class of textual data with added complexity due to varied formats (short tweets, long-form blogs, etc.), multimedia content (images, videos, audio), and semantic nuances (slang, emojis, etc.). Understanding this data involves elements of text analysis, sentiment analysis, and more.
Mastering the analysis of these unstructured data types can hold the key to unlocking valuable insights from an expansive sea of information.
Structured data vs Unstructured data
In this section, we'll discuss the key differences between structured and unstructured data, two different types of information frequently encountered in data analysis.
Definition and Organization
Structured data is well-organized, adheres to a fixed format, and is easily stored and queried in relational databases. It typically comprises data that can be arranged in rows and columns, like addresses, dates, or product information.
On the other hand, unstructured data lacks a predefined schema or structure, making it more difficult to analyze. It consists of data such as text, images, videos, and other complex formats that cannot be easily organized in traditional databases.
Storage Mechanisms
Structured data is typically stored in relational databases, like SQL, which are designed to handle structured information efficiently. Queries can be performed using SQL syntax, providing efficient access to stored data.
Unstructured data can be stored in various ways based on the specific use case. NoSQL databases, data lakes, and object storage are common storage options for handling unstructured data, often accommodating data with a flexible schema or no schema at all.
Data Analysis and Processing
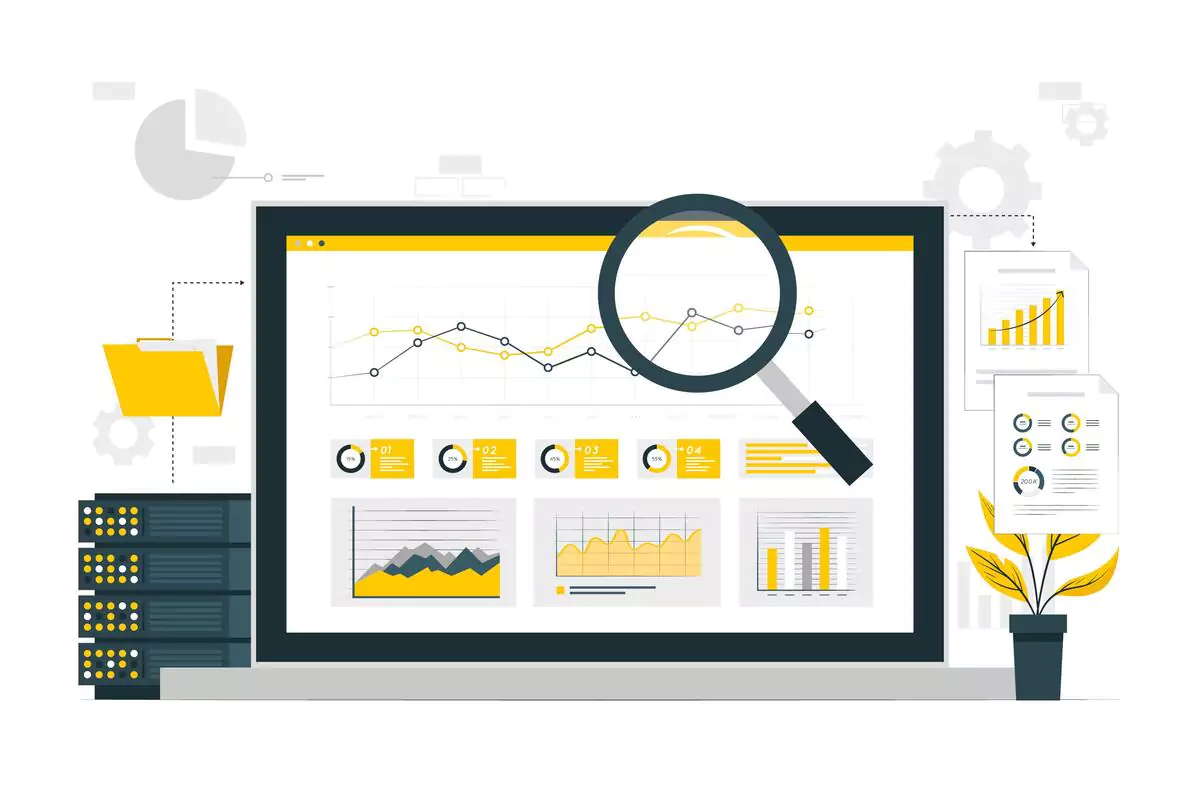
Since structured data is organized and formatted uniformly, traditional analytical methods and tools can be directly employed. It is easy to extract, manipulate and report structured data using tools like business intelligence software or SQL querying.
In contrast, unstructured data requires advanced processing methods to extract useful insights. Techniques like natural language processing (NLP), machine learning (ML), and artificial intelligence (AI) are commonly used to analyze and make sense of unstructured data.
Data Sources
Structured data often comes from highly organized sources like transaction logs, order details, sensor measurements, or survey responses. This type of data is generated through formal and well-defined processes, resulting in a consistent and predictable format.
Unstructured data is generated from various sources such as emails, social media posts, video recordings, images, and web pages. The nature and format of unstructured data can vary greatly based on the context and the source generating it.
Prevalence
Historically, structured data represented the bulk of the data generated and processed. However, with the increasing digitization of society and the rapid expansion of the internet, unstructured data is growing exponentially. Today, unstructured data accounts for the majority of the data being generated, storing immense potential for analysis and insights.
Applications of Unstructured Data
In this section, we'll delve into the various applications of Unstructured Data across fields and industries, highlighting its immense potential and value.
Enhancing Customer Understanding
Unstructured data like customer reviews and social media posts offer invaluable insights into customer expectations, preferences, and sentiments. Businesses use this data to tailor their products and services, creating personalized customer experiences.
Enhancing Machine Learning Models
Unstructured data plays a crucial role in training and refining machine learning algorithms. Text, images, and audio data help these models gain a deeper understanding of complex patterns and phenomena, resulting in improved predictions and decision-making.
Fuelling Medical Research
In the healthcare sector, unstructured data from medical records, patient histories, and research papers can be leveraged to enhance diagnostic accuracy, personalize treatment plans and drive medical innovations.
Expediting Legal Processes
Law firms use unstructured data to analyze legal documents, contracts, and case studies. This analysis aids in understanding legal precedents, expediting case reviews, and formulating effective strategies.
Bolstering Cybersecurity Measures
In cybersecurity, unstructured data from log files, network traffic, threat intelligence feeds, and more help identify patterns indicative of security breaches, anomalous behavior, or emerging threats, helping organizations to improve their security posture and response.
Exploring Tools for Unstructured Data Management
This section will help you understand unstructured data management and discover tools that help analyze, process, and visualize these complex datasets.
Text Analytics and NLP Tools
These tools enhance our capacity to process textual data, ranging from business reports to social media posts. They include Python libraries such as NLTK, spaCy, and Gensim, or dedicated platforms like IBM Watson or Google Cloud Natural Language.
Speech Recognition and Processing Libraries
Audio data management tools are oriented towards processing voice or speech data effectively. For instance, Python's Speech Recognition and PyDub libraries or APIs like Google Cloud Speech-to-Text and Mozilla's DeepSpeech.
Video Analytics Libraries
Video analytics tools enable us to process vast video datasets and extract meaningful insights. Examples include the OpenCV library or cloud-based platforms like Amazon Rekognition, Google Video Intelligence, or Microsoft Azure Video Indexer.
Image Recognition and Processing Frameworks
These tools cater to unstructured images or graphics data by recognizing and processing visual content. Notable examples include TensorFlow, Keras, and PyTorch, or cloud-based APIs such as Google Cloud Vision, Amazon Rekognition, or Microsoft Azure Custom Vision.
Data Visualization and Reporting Tools
Tools that facilitate the visualization of unstructured data, enabling us to discover trends and insights otherwise hard to discern. Some favorites include Tableau, Power BI, Plotly, Looker, and Qlik.
Harnessing these powerful tools, you'll have the capability to effectively manage and unlock the hidden value within your unstructured data.
Challenges of Unstructured Data
As enticing as unstructured data may be, it comes with its fair share of challenges. Let's explore a few of them:
- Volume and Velocity - The sheer amount and speed of unstructured data being generated can be overwhelming to traditional IT infrastructure. Requires scalable big data systems.
- Variety - Unstructured data comes in many formats like video, images, audio, documents, logs, etc. Processing diverse data types is difficult with traditional databases.
- Complexity - Unstructured data lacks organization and context. Deriving insights involves complex analytical techniques like NLP and machine learning.
- Quality - Irrelevant, redundant, biased or erroneous unstructured data can lead to poor analysis outcomes. Maintaining quality at scale is challenging.
- Security - Securing decentralized data like documents, messaging data, IoT data is difficult compared to structured databases. More vulnerable to breaches.
- Compliance - Adhering to regulations around data privacy, retention and sovereignty gets complex with fluid unstructured data spread across silos.
- Storage and Management - The dynamic nature of unstructured data makes it difficult to store and manage efficiently long-term compared to structured data.
- Integration - Unstructured data analysis depends heavily on integrating disparate data sources. Introduces technological and organizational challenges.
- Skill Gap - Data scientists capable of extracting insights from unstructured data are in short supply. Legacy skillsets lag modern requirements.
- Justifying ROI - Tangible ROI from unstructured data analytics can be hard to demonstrate compared to traditional structured data analysis.
Suggested Reading:
Frequently Asked Questions (FAQs)
What is unstructured data?
Unstructured data refers to any data that doesn't fit into a traditional structured database. It includes things like emails, social media posts, and documents.
How is unstructured data different from structured data?
Unlike structured data, unstructured data doesn't follow a predefined format. It lacks a specific organization and can be difficult to analyze using traditional methods.
What are some examples of unstructured data?
Examples of unstructured data include text documents, images, audio and video files, social media posts, emails, spreadsheets, and presentations.
How is unstructured data managed?
Unstructured data can be managed through data mining techniques, natural language processing, and machine learning algorithms. These methods help to extract meaningful insights from the data.
Why is unstructured data important?
Unstructured data contains valuable information that can be used for business intelligence, customer insights, and decision making. It provides a more comprehensive view of user behavior and preferences.