What is Few-Shot Learning?
You remember how, as a child, your mom showed you a couple of cats, and you learned to recognize all cats? That's exactly how few-shot learning works—it's a concept in machine learning where models learn from a very limited amount of data—just like a quick learner.
Terminology: Shots
In the world of few-shot learning, 'shots' depict the amount of data or instances we're working with. Consider shots as hints a student gets before a surprise quiz; fewer the hints, more challenging the test.
Categorizations: One-Shot & Zero-Shot Learning
Depending on the quantity of data used, few-shot learning can be further categorized into two types—One-Shot and Zero-Shot learning.
These are like learning to sketch from observing just one sketch or learning to sketch without a reference, respectively.
Significance of Few-Shot Learning
The beauty of Few-Shot Learning lies in its simplicity and practicality. It's like cherry-picking information to simplify learning—it doesn't require a bulk of data to be effective and is superbly efficient.
Who uses Few-Shot Learning?
Few-Shot Learning isn't just for nerdy tech wizards. Let's see who finds this concept particularly useful.
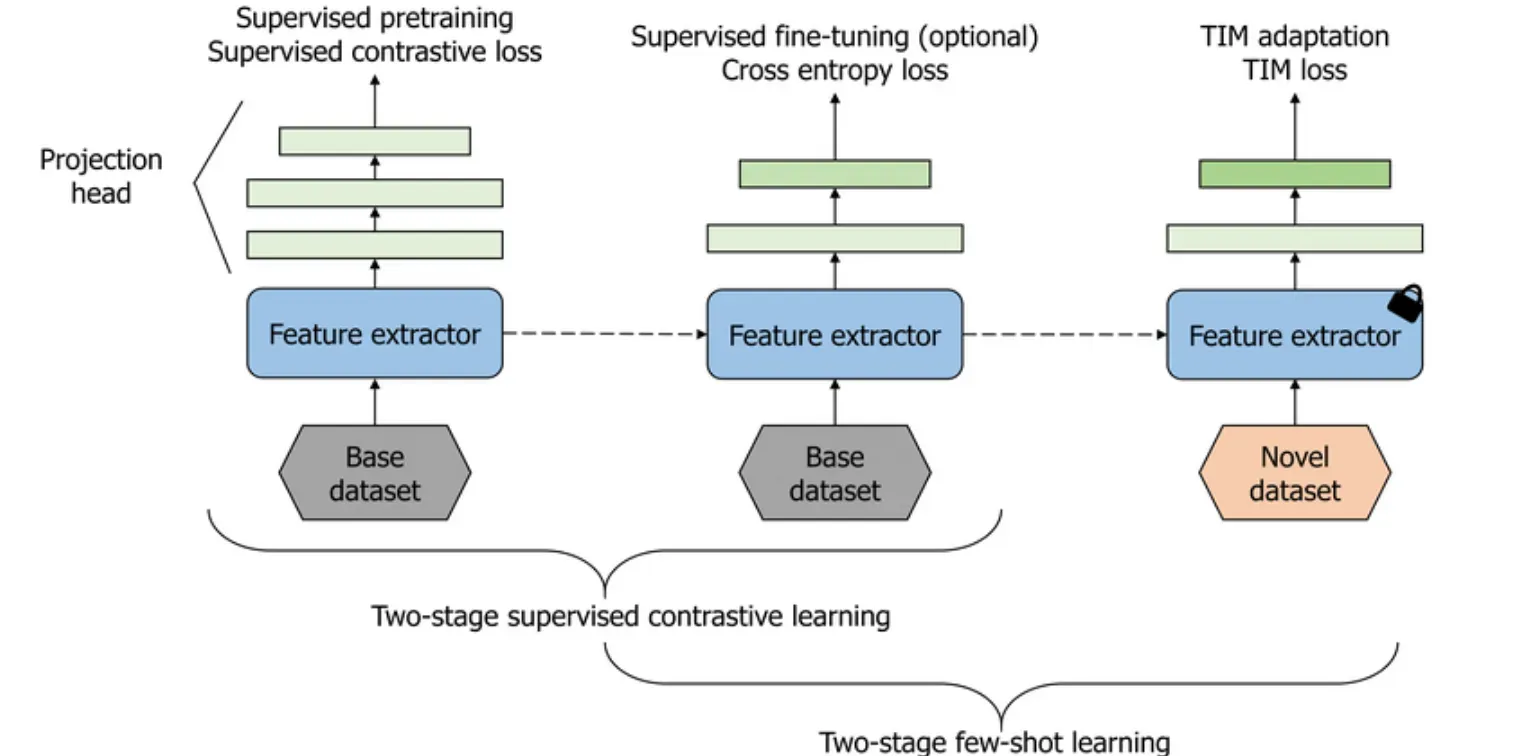
AI Researchers
AI researchers make extensive use of Few-Shot Learning to build efficient models with limited data at hand. Think of them as master chefs experimenting with a few ingredients to whip up a gourmet meal.
Businesses
Not every business has access to an exhaustive amount of data. For them, few-shot learning is a blessing, enabling them to make effective decisions and get AI-driven insights with limited information, much like a shrewd businessperson making moves based on the market pulse.
Healthcare Professionals
Imagine a doctor figuring out a rare disease from a handful of symptoms—that's where Few-Shot Learning comes in handy in healthcare. With limited patient data, professionals can identify patterns and diagnose illnesses.
Computer Vision Experts
When you're teaching a computer to recognize images or patterns, Few-Shot Learning is your best friend, helping achieve remarkable results with just a few examples.
Why is Few-Shot Learning Important?
Now we've got an idea of 'what' and 'who', let's examine 'why' Few-Shot learning is such a revolution in machine learning.
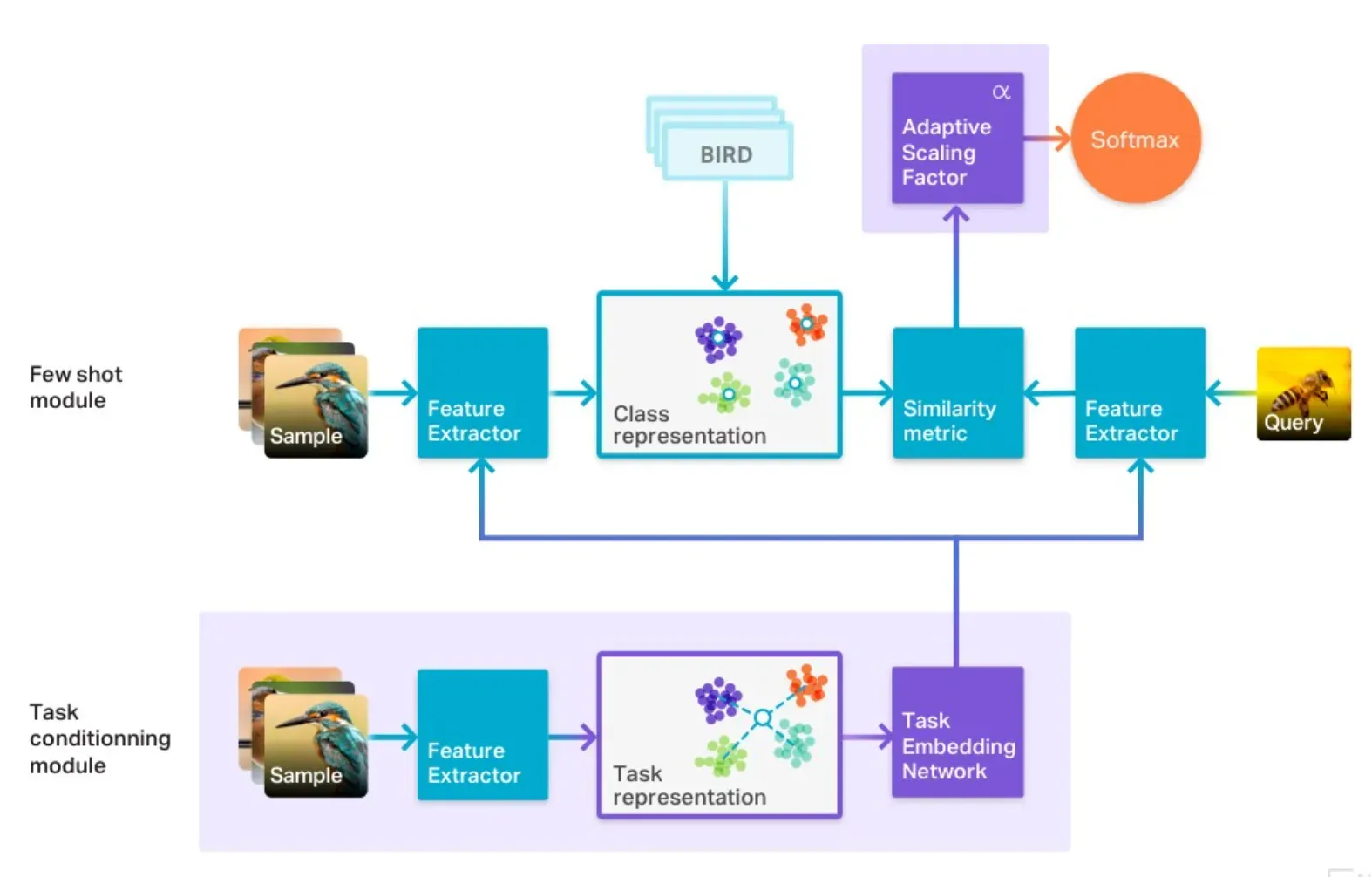
Addresses Data Deficiency
Few-Shot Learning overcomes the hurdle of data scarcity, much like finding a clear path in unfamiliar terrain. It's a boon for those who do not have copious amounts of data at their disposal.
Saves Time & Resources
It significantly reduces the computational capacity and time consumed in learning—from several weeks of training to a few hours or even minutes, like an express elevator to the destination.
Enables Quick Learning
Few-Shot Learning shines in encouraging quick intelligence from minimum data—kind of like getting a grasp of the game rules quickly to enjoy it better.
Enhances Generalization
Few-Shot Learning enhances the model's ability to generalize from limited data—definitely a leap forward towards achieving AI that can learn like a human.
When is Few-Shot Learning Used?
Let's take a look at situations where Few-Shot learning can be leveraged to gain maximum results.
Limited Data Scenarios
When there's a limited bunch of data, for instance, detecting rare diseases from a small set of patient data, Few-Shot Learning is the 'go-to' method, like using a flashlight in the dark.
Quick Adaptations
In scenarios where models need to learn and adapt quickly to new tasks with just a few examples, this concept is a lifesaver. It's like quickly improvising and winning a shooting game with only a few bullets left.
Real-time decision-making
In applications where quick and efficient decisions are needed based on limited data, few-shot learning gets the place of honor, like a fighter making quick moves based on the opponent's limited actions.
Low-resource Environments
In environments where computational resources are limited, few-shot learning comes in handy to deliver results without straining resources, much like making a delectable dish with limited ingredients.
Where is Few-Shot Learning Applied?
Now that we know when to use it, let's delve into practical applications where Few-Shot Learning shines like a superstar.
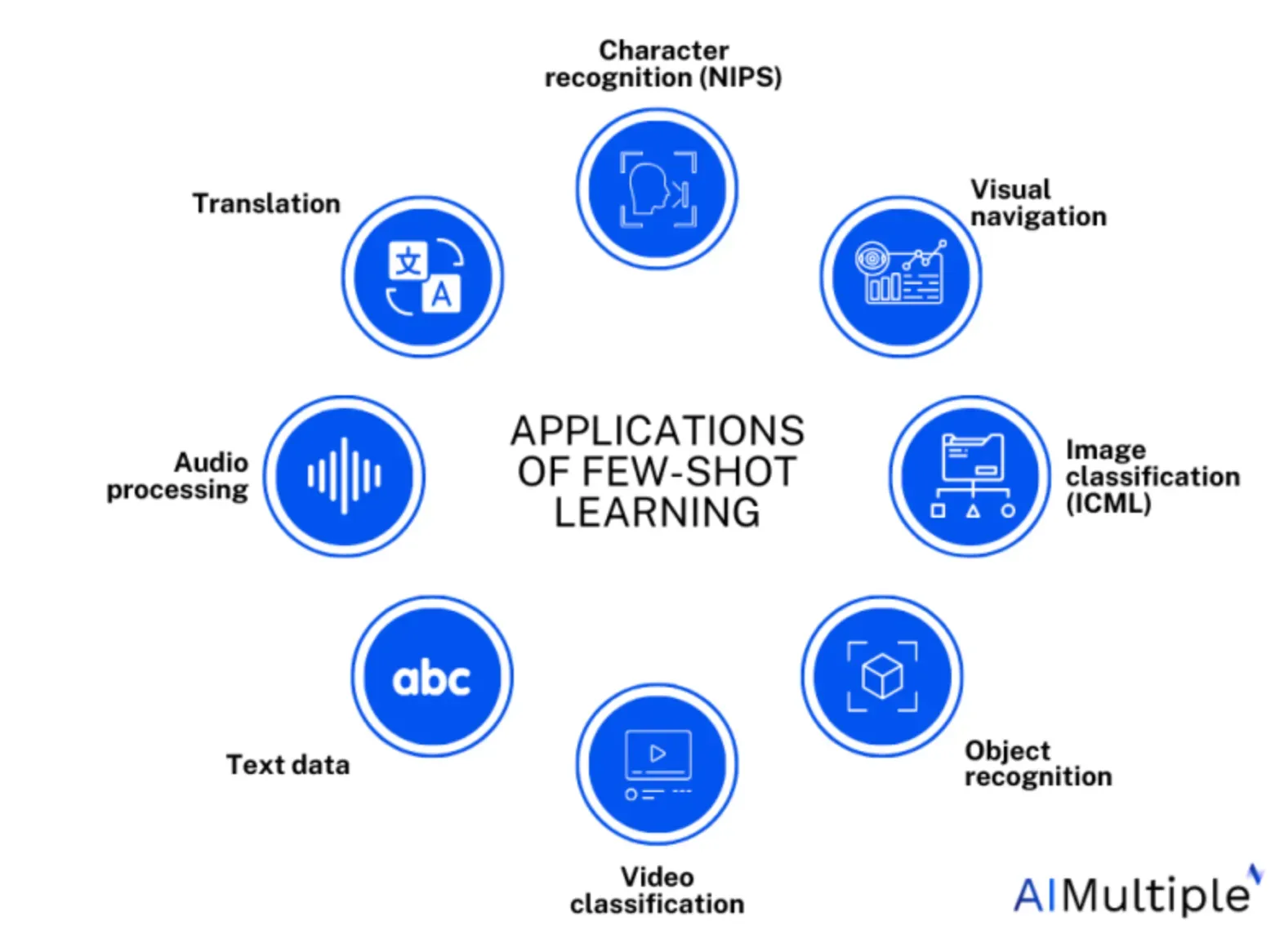
Computer Vision
In Computer Vision, Few-Shot Learning helps in image or object recognition just from a few examples—like recognizing your favorite bird in a crowded aviary.
Natural Language Processing (NLP)
Leveraging Few-Shot Learning, NLP models learn to comprehend and generate human-like responses from scarce language data—it's like learning a new language quickly from a phrasebook.
Healthcare
Few-Shot Learning assists in diagnosing rare diseases from a tiny number of patient records or predicting patient outcomes based on minimum patient history, much like Sherlock Holmes solving a complex case from a few clues.
Anomaly Detection
In systems that require anomaly detection, like intrusion detection or credit card fraud detection, Few-Shot Learning helps the system detect abnormal behavior from a limited set of examples—it's akin to spotting a miscreant among a bunch of people.
How does Few-Shot Learning work?
Finally, let's unveil the 'how' of the Few-Shot Learning magic.
Meta-Learning
At the heart of Few-Shot Learning lies Meta-Learning. Here, the model learns to learn from minimum data—like you learn how to learn a new musical instrument quickly.
Optimization
After initial learning, Few-Shot Learning invigorates the model optimization process so that learning happens more effectively—like tweaking the tuning of your newly learned musical instrument.
Evaluating Performance
The performance of a few-shot learning model is routinely evaluated based on how well it performs on new, unseen tasks using precision, recall, and other metrics, like a pianist receiving feedback to perfect a complicated piece.
Deployment
Finally, once the model is well-optimized and has shown good performance, it's deployed to take on real-world tasks, like a well-trained guard ready for his watch.
Frequently Asked Questions (FAQs)
Can Few-Shot Learning Be Applied to Any Machine Learning Problem?
Few-Shot Learning can be supremely helpful in problems where data scarcity is an issue. However, it might not be the ideal candidate for tasks requiring extensive data for precision.
How Does Few-Shot Learning Differ from Traditional Machine Learning?
While traditional machine learning thrives on large data sets, few-shot learning, on the other hand, learns effectively from a modest amount of data.
Why Can't We Always Use Few-Shot Learning?
Few-Shot Learning can excel at tasks with scarce data but might not yield the best results for tasks highly dependent on the degree of granularity that comes with more extensive data.
Can Few-Shot Learning Be Used in Unsupervised Learning?
In theory, it could be, but in practice, Few-Shot Learning is typically used in the realm of supervised learning.
Is Few-Shot Learning the Future of AI?
Few-Shot Learning is undoubtedly a significant step towards achieving AI that learns more like a human but to call it the 'future' might be a simplification as AI is a broad and ever-evolving field.