Introduction
In today's data-driven world, machine learning (ML) has become an indispensable tool for businesses. As it is important for seeking to gain valuable insights, automate processes, and drive innovation.
However, developing and deploying ML models effectively can be a complex and resource-intensive endeavor. It requires specialized expertise and infrastructure.
This is where ML model engineering services come into play. Further offering organizations the ability to leverage cutting-edge machine learning capabilities without the need for extensive in-house resources.
The global machine learning market is expected to grow from $21.17 billion in 2022 to $209.91 billion by 2029, at a CAGR of 38.8% (Source: Fortune Business Insights, 2023). This rapid growth is fueled by the increasing demand for ML solutions across industries, ranging from healthcare and finance to retail and manufacturing.
ML model engineering services provide end-to-end support, from data preparation and model training to optimization, deployment, and monitoring. By outsourcing these specialized tasks to experienced professionals, businesses can focus on their core competencies while ensuring the effective integration of ML into their operations.
This article will provide valuable insights into the advantages of partnering with ML model engineering service providers. So let’s get started with the first use case of having the ML model engineering services.
Fraud Detection
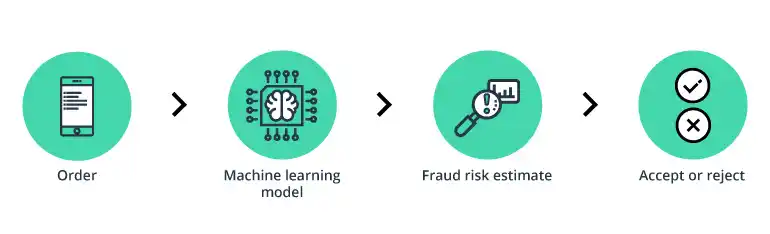
Fraud is a growing concern for businesses across various industries. Companies are constantly at risk of losing substantial amounts of money due to fraudulent activities.
Fortunately, Machine Learning (ML) model engineering services are making a significant impact in the fight against fraud.
ML models can analyze large volumes of data and identify patterns that indicate fraudulent behavior.
By leveraging historical data, these models can learn to recognize patterns associated with fraudulent transactions, enabling businesses to detect and prevent fraud in real-time.
ML can help organizations identify fraudulent activities in multiple scenarios. For example, ML models can be trained to detect anomalies in credit card transactions.
By analyzing factors such as transaction amount, time, and location, ML algorithms can quickly identify suspicious patterns and prompt immediate action. This not only saves businesses from financial losses but also protects their customers from unauthorized charges.
Fraud detection using ML models is not limited to the financial sector. ML can also be applied to identify insurance fraud, such as fake claims or fraudulent medical procedures.
By analyzing historical data and identifying suspicious patterns, ML models can assist insurance companies in reducing fraudulent claims and saving millions of dollars.
The use of ML for fraud detection is an ongoing process. ML models need to be constantly updated and trained with fresh data to stay ahead of new fraud techniques and adapt to changing patterns.
This dynamic approach ensures that organizations can stay one step ahead of fraudsters and protect themselves from potential threats.
Customer Churn Prediction
Keeping existing customers is as important as acquiring new ones. In today's competitive market, businesses need to understand customer behavior to retain loyal customers and prevent churn.
ML model engineering services provide effective solutions for customer churn prediction.
By analyzing various customer-related data such as past purchases, customer complaints, and browsing patterns, ML models can identify factors that contribute to customer churn.
By identifying customers who are at risk of churning, businesses can implement targeted retention strategies.
These strategies may include personalized offers, loyalty programs, or proactive customer support.
By predicting customer churn, businesses can take preventive measures to retain valuable customers, reducing the negative impact on their revenue and reputation.
ML models can also help businesses identify potential reasons behind customer churn. For example, by analyzing customer feedback, ML algorithms can detect common pain points and areas where businesses need improvement.
This valuable insight can be used to enhance products or services, providing a more satisfying customer experience and reducing churn in the long run.
Additionally, ML models can help businesses segment customers based on their likelihood of churn.
By identifying common characteristics among customers who have churned in the past, businesses can proactively target similar customers and prevent churn before it happens.
Overall, ML model engineering services enable businesses to gain a deeper understanding of customer behavior and take proactive measures to reduce churn.
By leveraging ML algorithms, businesses can retain valuable customers and ensure long-term success.
Product Recommendation
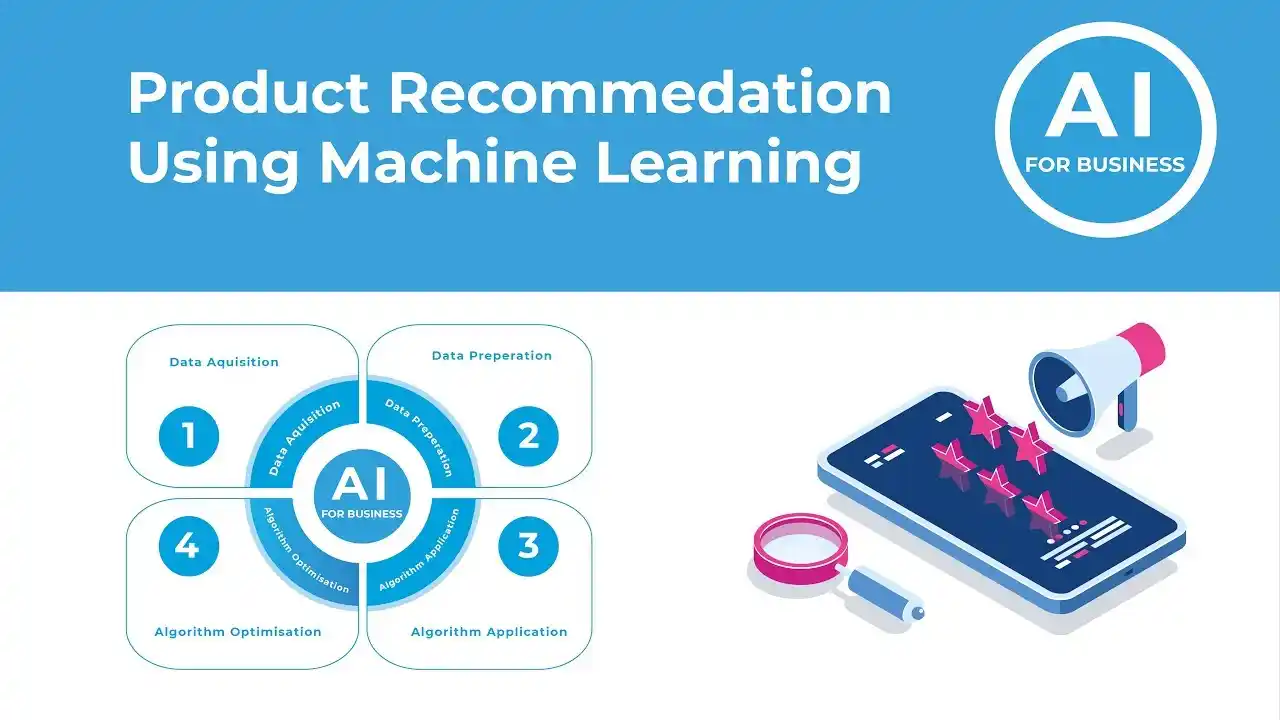
With the increasing amount of data generated by customers, businesses are looking for ways to personalize their offerings and enhance customer experiences.
ML model engineering services pave the way for effective product recommendations that cater to individual customer preferences.
ML models analyze customer data such as past purchases, browsing history, and demographic information to generate personalized recommendations.
By identifying patterns and similarities among customers, ML algorithms can suggest products or services that customers are likely to be interested in.
Product recommendation models can be particularly effective in e-commerce businesses, where customers have a wide range of options.
By providing personalized recommendations, businesses can increase customer engagement, boost sales, and enhance customer satisfaction.
ML models can also help businesses improve cross-selling and upselling strategies. By analyzing customer behavior and identifying purchasing patterns, ML algorithms can suggest complementary products or premium versions of existing products.
This not only increases revenue but also enriches the overall shopping experience for customers.
Medical Diagnosis
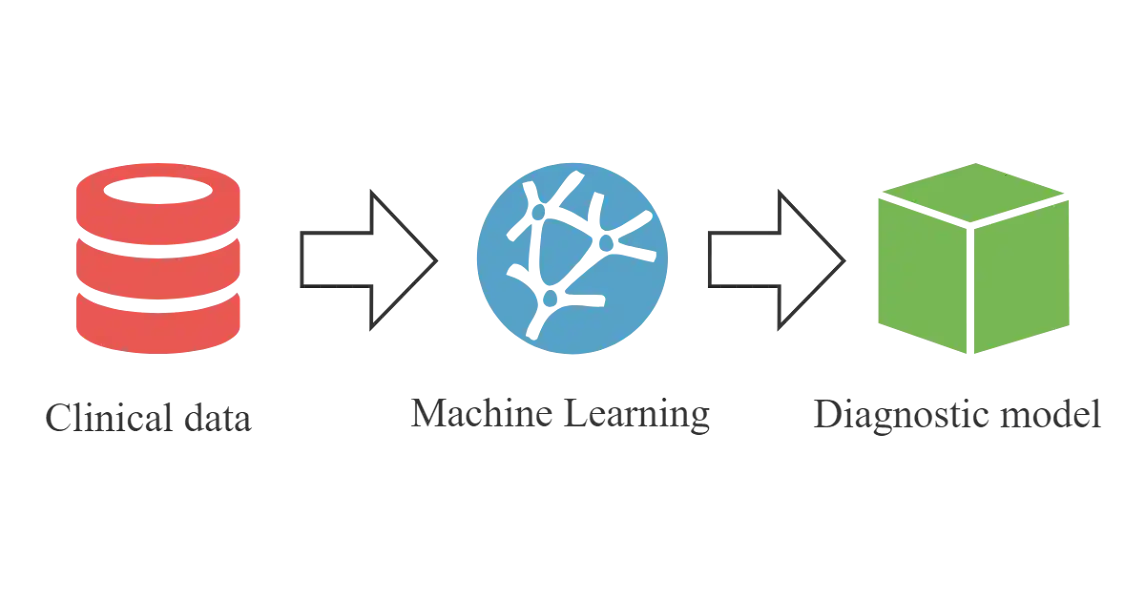
ML model engineering services are revolutionizing the field of medical diagnosis, enabling healthcare professionals to make more accurate and timely diagnosis.
By leveraging ML algorithms, medical data, and advanced analytics, ML models can assist doctors in identifying diseases, predicting outcomes, and recommending treatment options.
ML models can analyze a vast amount of patient data, including medical records, lab results, and imaging studies.
By learning from past cases, ML algorithms can detect patterns and correlations that human doctors may miss. This can lead to earlier detection of diseases, more precise diagnosis, and improved patient outcomes.
For example, ML models can assist in the early detection of cancer by analyzing patient data and identifying potential risk factors.
By recognizing subtle patterns and deviations in medical images, ML algorithms can help radiologists and oncologists make more accurate diagnoses.
Thus enabling patients to receive timely treatment and improving their chances of recovery.
Now, if you want to begin with machine learning development to enhance your customer service but have no clue about how to do it, then check out the NO-CODE chatbot platform, named BotPenguin.
With all the heavy work of chatbot development already done for you, BotPenguin allows users to integrate some of the prominent language models like GPT 4, Google PaLM, and Anthropic Claude to create chatbots that are powered by machine learning for platforms like:
- WhatsApp Chatbot
- Facebook Chatbot
- Wordpress Chatbot
- Telegram Chatbot
- Website Chatbot
- Squarespace Chatbot
- Woocommerce Chatbot
Drug Discovery
The process of discovering new drugs is a complex and time-consuming task that requires extensive research, testing, and analysis.
ML model engineering services are revolutionizing the field of drug discovery by speeding up the process and improving the success rate of identifying potential drug candidates.
ML models can analyze vast amounts of biological and chemical data to identify patterns and relationships between molecules and their effects on the human body.
By learning from existing drug data and clinical trial results, ML algorithms can predict the effectiveness of potential drug candidates and assist researchers in identifying promising molecules for further study.
ML models can also assist in optimizing drug formulations and dosage recommendations. By analyzing patient data and drug response patterns, ML algorithms can provide personalized dosage guidelines, maximizing the effectiveness of treatments and minimizing side effects.
Additionally, ML models can help researchers identify potential drug targets and uncover hidden relationships between diseases and biological mechanisms.
By analyzing complex biological data, ML algorithms can identify novel pathways and targets for drug intervention, enabling the development of innovative and targeted therapies.
By leveraging ML in drug discovery, researchers can reduce the time and cost associated with traditional trial-and-error approaches.
ML models can prioritize potential drug candidates, guiding researchers towards molecules with a higher likelihood of success, and streamlining the drug development process.
Suggested Reading:
Machine Learning Development in Action: Real World Use-Cases
Anomaly Detection
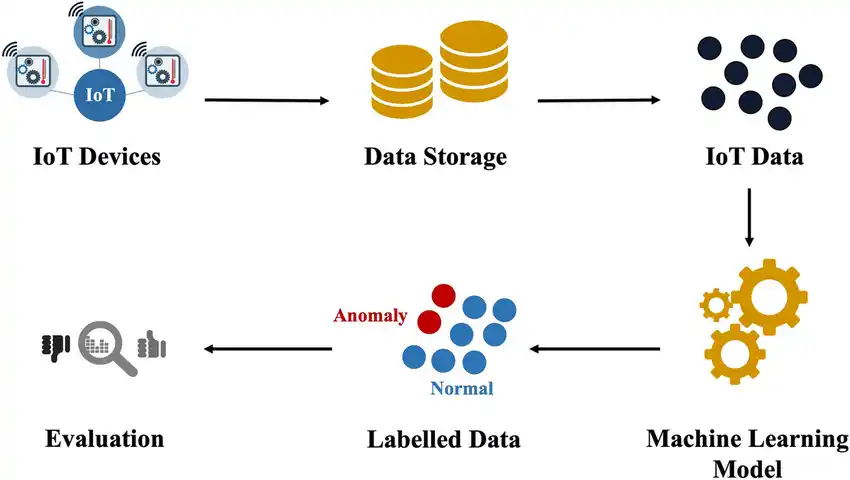
Detecting anomalies or unusual patterns in data plays a crucial role in various industries, including finance, cybersecurity, and manufacturing.
ML model engineering services offer robust solutions for anomaly detection, enabling businesses to identify abnormalities and take prompt action.
ML models can analyze large volumes of data and learn normal patterns, enabling them to detect deviations from expected behavior.
By training the models with historical data, ML algorithms can identify anomalies in real-time, alerting businesses to potential issues or threats.
In finance, for example, ML models can detect fraudulent transactions by analyzing factors such as transaction amounts, time, and location.
By identifying unusual patterns, ML algorithms can flag potentially fraudulent activities and prompt immediate action, saving businesses from financial losses and protecting their customers.
In cybersecurity, ML models can detect abnormal network activity or suspicious behavior that may indicate a cyber attack.
By analyzing network traffic, user behavior, and system logs, ML algorithms can identify anomalies and raise alarms, allowing organizations to respond quickly and protect their systems from potential breaches.
Predictive Maintenance
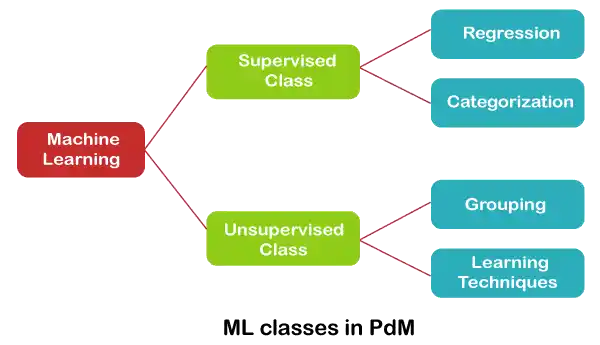
Predictive maintenance is a proactive approach that uses ML model engineering services to detect potential equipment failures before they occur.
By analyzing real-time data from sensors, ML models can predict when and how equipment might fail. Thus enabling businesses to schedule maintenance and prevent costly downtime.
ML models can analyze data such as temperature, vibration, and performance metrics from equipment and identify patterns that indicate deterioration or impending failure.
By continuously monitoring equipment and comparing real-time data to historical patterns, ML algorithms can predict maintenance needs and trigger alerts when anomalies are detected.
This predictive approach allows businesses to move away from traditional reactive maintenance, where equipment failures and unplanned downtime disrupt operations and result in costly repairs.
By implementing predictive maintenance strategies, businesses can reduce maintenance costs, optimize resource allocation, and ensure the reliability of their equipment.
Natural Language Processing (NLP)
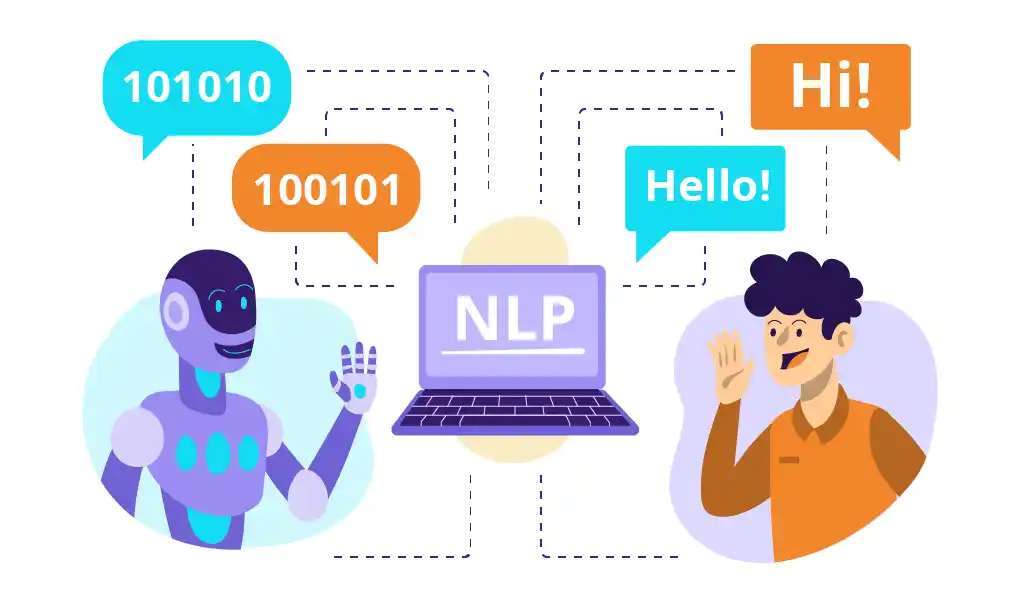
Natural Language Processing (NLP) is a subfield of ML that focuses on the interaction between computers and human language.
NLP uses ML model engineering services to process, understand, and derive meaning from natural language data such as text and speech.
NLP models can perform a wide range of language-related tasks, including sentiment analysis, entity recognition, text summarization, and language translation.
By applying ML algorithms to large amounts of textual data, NLP models can extract insights, automate tasks, and enable more effective communication between humans and machines.
One of the key applications of NLP is sentiment analysis, which involves determining the attitude or opinion expressed in a piece of text.
NLP models can analyze customer reviews, social media posts, and other text sources to identify the sentiment, helping businesses gauge public opinion and make informed decisions.
Another application of NLP is language translation. ML models can be trained on large multilingual datasets to automatically translate text from one language to another.
This has significant implications for global businesses. Thus enabling them to communicate with customers and partners across different languages.
Suggested Reading:
How to Solve Business Problems with Machine Learning Development
Computer Vision
Computer Vision is a branch of ML that focuses on enabling computers to interpret and understand visual data, such as images and videos.
By applying ML model engineering services, computer vision can be used to analyze and extract valuable information from visual content.
Computer Vision models can perform a wide range of tasks, including image recognition, object detection, and image segmentation.
By training ML models on large datasets containing labeled images, the models can learn to identify and classify objects or features within images.
Robotic Process Automation (RPA)
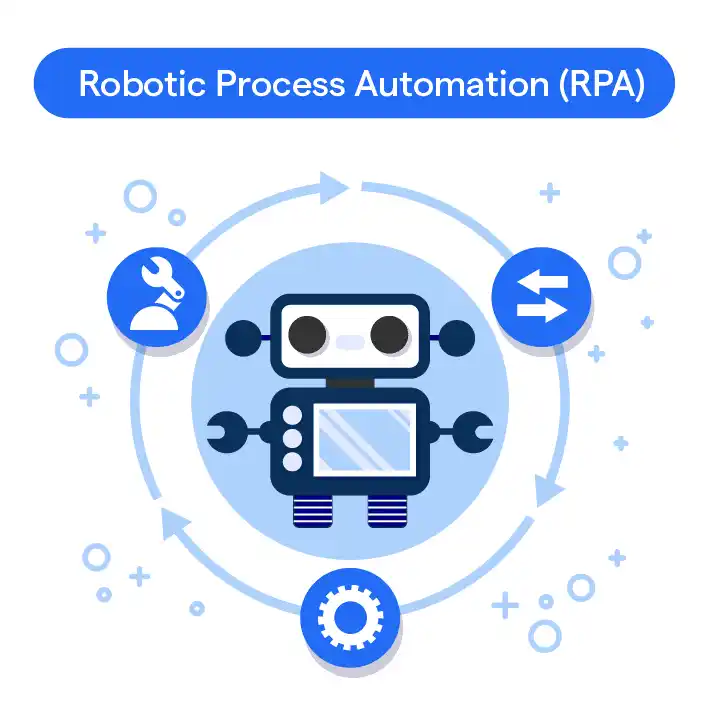
Robotic Process Automation (RPA) is a technology that uses ML model engineering services to automate repetitive and rule-based tasks previously performed by humans.
RPA models can analyze and mimic human actions, enabling businesses to streamline processes, reduce errors, and increase efficiency.
RPA models can automate a wide range of tasks across different industries, such as data entry, invoice processing, and customer support.
By learning from human actions and interactions, ML algorithms can replicate these tasks and perform them automatically, eliminating the need for human intervention.
One of the key benefits of RPA is its ability to reduce errors and increase accuracy. Unlike humans, RPA models do not get tired, distracted, or make mistakes due to human error. This results in improved data quality and increased operational efficiency.
RPA models can also work 24/7, without the need for breaks or rest, ensuring uninterrupted productivity. By automating repetitive tasks, businesses can free up human resources to focus on more complex and value-added activities.
Thus resulting in improved productivity and employee satisfaction.
Conclusion
In conclusion, ML model engineering services have emerged as a powerful tool for businesses to leverage the capabilities of machine learning in various domains.
From predictive maintenance to natural language processing, computer vision, and robotic process automation, ML models are revolutionizing the way businesses operate.
These use cases provide tangible benefits such as reducing downtime, improving communication, extracting insights from visual data, and automating repetitive tasks.
By harnessing the power of ML model engineering services, businesses can stay competitive, enhance operational efficiency, and make data-driven decisions.
The possibilities for ML applications are endless, and it is clear that ML model engineering services are indispensable in today's technology-driven world.
Frequently Asked Questions (FAQs)
What are ML model engineering services?
ML model engineering services help businesses design, develop, deploy, and manage machine learning models.
What are the benefits of using ML model engineering services?
These services offer expertise, efficiency, and resources to overcome challenges in the ML model development lifecycle.
What are some common use cases for ML model engineering services?
Businesses use these services for tasks like fraud detection, product recommendation, and customer churn prediction.
Can ML model engineering services help improve business processes?
Yes, by automating tasks, optimizing operations, and enabling data-driven decision-making.
What industries can benefit from ML model engineering services?
A wide range of industries can benefit, including finance, healthcare, retail, and manufacturing.