mm,Introduction
In an era where technology continues to redefine human interaction, Emotion AI stands as a testament to the evolving capabilities of artificial intelligence.
At the heart of this revolution lies Sentiment Analysis, a groundbreaking application that enables AI to decode the intricacies of human emotions embedded within vast digital landscapes.
From deciphering the sentiment behind casual tweets to extracting insights from formal texts, Sentiment Analysis sifts through over 500 million daily expressions. It offers a panoramic view of public opinion and paves the way for informed decision-making.
Natural Language Processing is the backbone of Emotion AI, and confronts the challenges inherent in interpreting the nuances of human emotions through digital mediums.
Yet, amidst these complexities, lies a world brimming with potential—a future where Emotion AI. So continue reading to know more about it.
What is Sentiment Analysis?
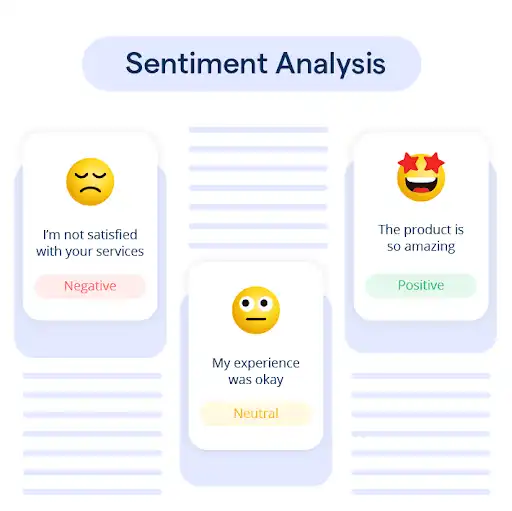
The exciting topic of sentiment analysis, or opinion mining, trains artificial intelligence (AI) systems to comprehend and decipher human emotions represented through textual data.
It entails examining text to ascertain the mood or emotional undertone.
Emotion AI can analyze enormous volumes of data and extract insightful information, regardless of whether the attitude is favorable, harmful, or neutral.
The Importance of Sentiment Analysis
Sentiment analysis plays a vital role in various industries. Companies can leverage it to monitor brand reputation, analyze customer feedback, conduct market research, and predict trends.
By understanding the sentiment of social media posts, product reviews, or customer surveys, businesses can make data-driven decisions, improve customer satisfaction, and stay ahead of the competition.
How Does Sentiment Analysis Work?
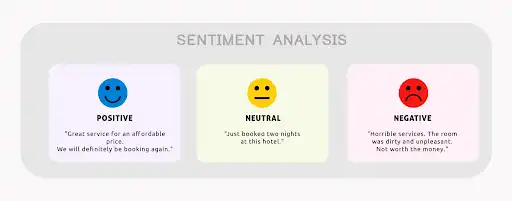
Sentiment analysis works on the following things:
Preprocessing and Data Collection
The text data must be preprocessed. This involves removing noise, such as punctuation and special characters, and handling capitalization and stemming.
Additionally, data collection is crucial, as a diverse and representative dataset is needed for training the AI models.
Text Classification Techniques
Text classification is at the core of sentiment analysis. Various techniques are employed to classify text into different sentiment categories.
Rule-Based Approaches: Rule-based approaches involve creating predefined rules and patterns to identify sentiment.
These rules can include keyword matching or regular expressions. While they provide a simple and interpretable solution, other approaches may need to be more accurate and adaptable.
Machine Learning Algorithms: Machine learning algorithms are commonly used in sentiment analysis.
Supervised learning techniques, such as Naive Bayes, Support Vector Machines (SVM), and Recurrent Neural Networks (RNN), are trained on labeled data to classify text into sentiment categories. These algorithms can learn from patterns and make predictions on unseen data.
Deep Learning Models: Deep learning models, particularly Convolutional Neural Networks (CNN) and Long Short-Term Memory (LSTM) networks, have gained popularity in sentiment analysis.
These models can capture complex relationships in text data and achieve high accuracy in sentiment classification.
Sentiment Analysis and Natural Language Processing (NLP)
A branch of artificial intelligence called "natural language processing" (NLP) aims to make it possible for computers to comprehend and process human language.
For sentiment analysis to improve sentiment categorization accuracy, NLP approaches are essential.
Tokenization and Lemmatization
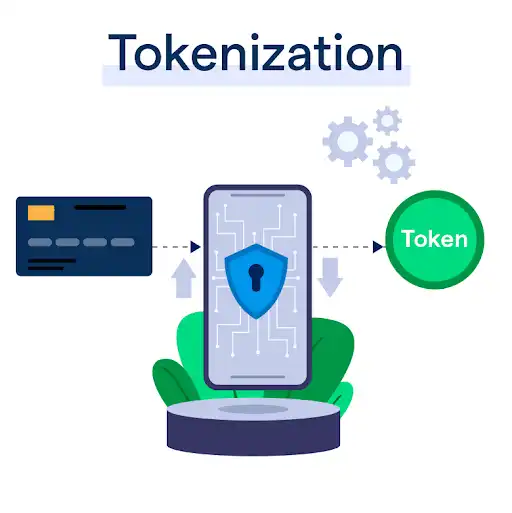
Tokenization involves separating text into smaller units, such as words or phrases, to analyze them individually.
Lemmatization helps to reduce words to their base or root form, allowing the AI models to recognize variations of the same sentiment.
Part-of-Speech (POS)
Tagging POS tagging is used to identify the grammatical structure of sentences.
It assigns labels to words, such as nouns, verbs, or adjectives, which aids in understanding the context and sentiment of the text.
Named Entity Recognition (NER)
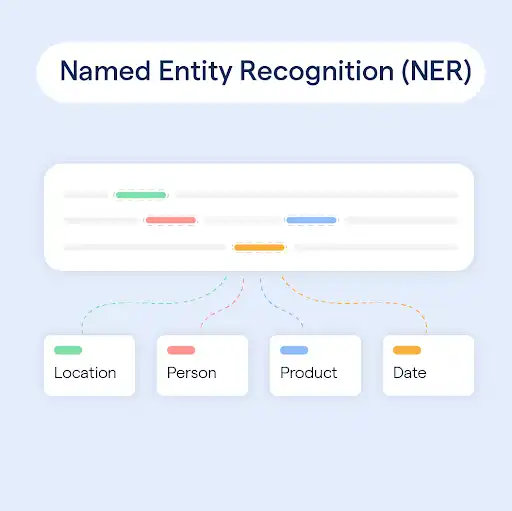
Named Entity Recognition (NER) involves identifying and classifying named entities in text, such as names, locations, or organizations.
This helps in sentiment analysis by capturing the sentiment associated with specific entities mentioned in the text.
Challenges in Sentiment Analysis
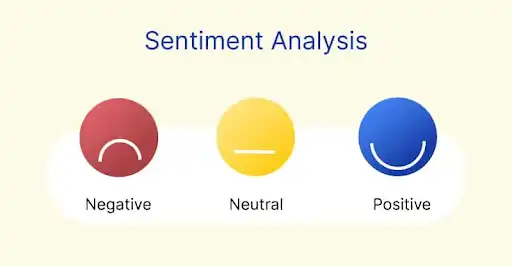
Here are a few of the challenges faced with sentiment analysis.
Ambiguity and Contextual
Understanding Language is inherently complex and can be ambiguous. The exact words can have different meanings based on the context in which they are used.
Understanding the context and disambiguating sentiments is a significant challenge in sentiment analysis.
Handling Sarcasm and Irony
Sarcasm and irony are forms of figurative language that convey sentiments opposite to the literal meaning of the words.
These linguistic nuances can confuse sentiment analysis models, as they need to comprehend the underlying tone and intention of the text.
Multilingual Sentiment Analysis
Sentiment analysis becomes even more challenging in multilingual environments.
Different languages have distinct grammatical structures, idiomatic expressions, and cultural nuances, making developing language-specific sentiment analysis models and datasets essential.
Conclusion
In this blog, we embarked on a captivating journey into Emotion AI and Sentiment Analysis. We explored the definition and importance of sentiment analysis, understanding how AI can analyze and interpret human emotions expressed through textual data.
We dived into the inner workings of sentiment analysis, including the preprocessing and data collection stages, as well as the text classification techniques such as rule-based approaches, machine learning algorithms like Naive Bayes and Support Vector Machines (SVM), and deep learning models like Recurrent Neural Networks (RNN).
Experience the transformative power of BotPenguin's chatbot services. With its intuitive AI, your business gains a competitive edge through personalized interactions and seamless automation.
Elevate customer satisfaction, streamline tasks, and achieve unparalleled success. Join the chatbot revolution with BotPenguin now!
Suggested Reading:
How ChatGPT Consultants can aid in AI Sentiment Analysis?