Introduction
Natural Language Understanding or NLU continues gaining tremendous commercial interest as part of broader conversational AI adoption. It is even projected to reach $42B in market size by 2030 according to Precedence Research (2022).
NLU specifically enables humanizing digital experiences by empowering machines to comprehend language, parse intent and generate contextual responses.
However business leaders continue grappling with many myths around NLU capabilities often fueled by hype and lacking real technical insight.
For instance over 54% presume NLU automatically implies realistic human mimicking when commercially deployed models still struggle with dynamic dialog state tracking, intent switching and sentiment analysis for open domains. Meanwhile 41% underestimate the multi-disciplinary effort spanning linguistics, human conversations analysis and robust validation needed to train such complex statistical systems reliably.
Without concrete understanding of inner workings supplemented by informed vendor selection, integration risks grow as 78% discover post-purchase while replacing ad-hoc regex scripts.
Hence clarifying NLU's strengths alongside limitations via unbiased knowledge sharing remains imperative. So continue reading to know more about the common myths about NLU.
Common Myths About NLU
Let's debunk some common myths surrounding NLU and gain a better understanding of its capabilities and limitations.
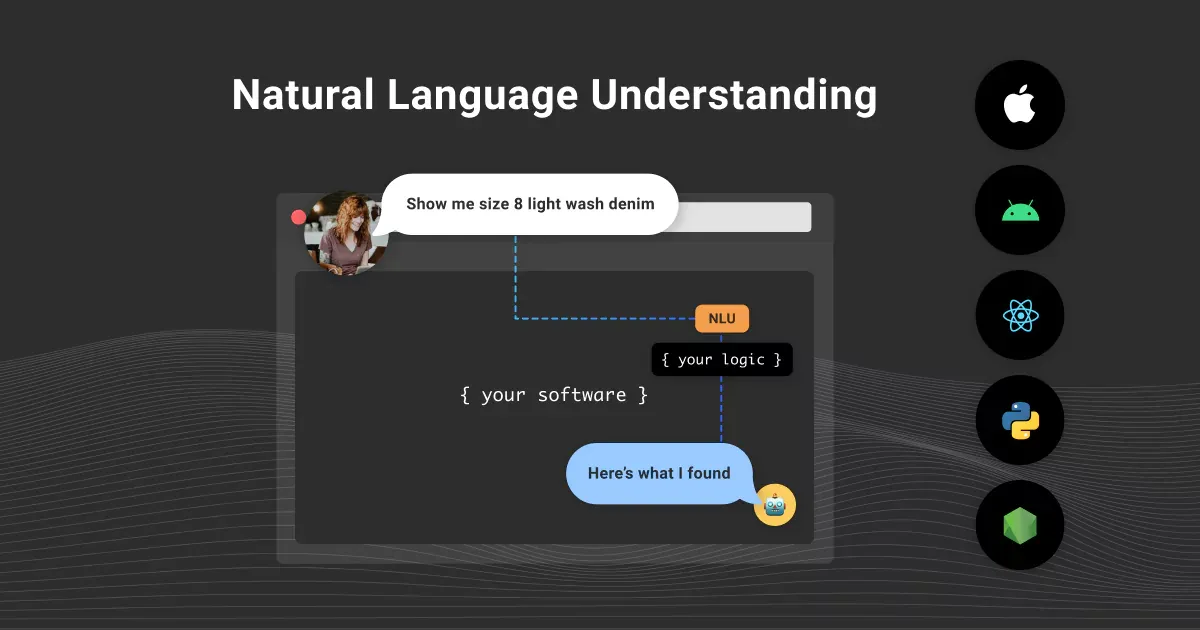
Myth #1: NLU is the same as Natural Language Processing (NLP)
While NLU is a part of NLP, they are not synonymous. NLP encompasses the entire process of working with human language, including tasks like text generation and sentiment analysis. NLU specifically focuses on understanding the meaning and intent behind language, allowing for more advanced and context-aware interactions.
Myth #2: NLU can understand all languages equally well
Despite significant advancements, NLU models still face challenges in comprehending all languages equally. Languages with a smaller amount of training data may have lower accuracy, and complexities in grammar and syntax can also pose difficulties. However, ongoing research and the development of multilingual models are continuously improving NLU's capabilities in diverse languages.
Myth #3: NLU algorithms are not customizable for specific businesses
Contrary to the misconception that NLU algorithms are rigid and non-adaptable, they can be highly customizable for specific business needs. NLU models can be trained on domain-specific datasets and fine-tuned to recognize industry-specific terminology and intents. This customization ensures that NLU systems are tailored to individual businesses, resulting in more accurate and contextually relevant understanding.
Myth #4: NLU can replace human customer service representatives
While NLU has made remarkable advancements in customer service automation, it is not intended to replace human representatives entirely. NLU-powered chatbots and virtual assistants can handle routine inquiries efficiently, freeing up human agents for more complex or emotionally sensitive interactions. The synergy of human expertise and NLU technology allows for a seamless and personalized customer experience.
Myth #5: NLU is only suitable for text-based interactions
NLU is not limited to text-based interactions alone. It has expanded to encompass voice recognition and speech-to-text conversion, enabling machines to understand spoken language as well. With advancements in Automatic Speech Recognition (ASR) and NLU, voice assistants like Siri and Alexa have become popular examples of utilizing NLU in voice-driven applications.
These myths reveal misperceptions about NLU's capabilities and highlight its true potential in understanding and processing human language. As research continues to progress, NLU will undoubtedly advance further, enhancing our ability to communicate and interact with machines naturally and effectively.
Dispelling Myths About NLU
Now, let's delve into the facts to dispel the myths surrounding NLU and gain a clearer understanding of its capabilities.
Fact #1: NLU and NLP are different but related concepts.
NLU focuses specifically on understanding the meaning and intent behind human language, while NLP encompasses a broader range of language processing tasks. NLU is just one piece of the puzzle in achieving comprehensive NLP systems. By differentiating between NLU and NLP, we can appreciate the specific advancements and challenges within each domain.
Fact #2: The quality of NLU can differ depending on the language being used.
NLU algorithms can excel in certain languages where there is an abundance of labeled training data. However, for languages with limited resources, the performance of NLU models may vary. Researchers and developers are actively working on improving NLU's accuracy and capabilities across diverse languages, as they understand the importance of linguistic diversity for global applications.
Fact #3: NLU algorithms can be trained specifically for individual businesses.
Contrary to the myth that NLU algorithms are one-size-fits-all, they can be trained and fine-tuned for specific businesses and industries. By providing domain-specific data and customization, NLU models can understand industry-specific terms and perfectly align with a business's needs. This customization ensures that NLU systems become powerful tools to enhance customer experiences and streamline operations within specific contexts.
Fact #4: NLU is designed to complement human customer service representatives, not replace them.
While NLU-powered chatbots and virtual assistants have transformed customer service interactions, their purpose is not to replace human agents. Rather, NLU systems are designed to complement human representatives by handling routine inquiries, providing quick and accurate responses, and freeing up human agents' time for more complex and critical tasks. The fusion of human expertise and NLU technology creates a powerful synergy in delivering exceptional customer service.
Fact #5: NLU can be used for a variety of interaction types, including voice-based interactions.
NLU's applications extend beyond text-based interactions and encompass voice-based interaction types as well. With advances in Automatic Speech Recognition (ASR) technology, NLU can interpret spoken language, enabling voice assistants like Siri and Alexa to understand user commands and queries. This functionality allows for a more natural and intuitive user experience, widening the possibilities for voice-driven applications.
By embracing these facts, we can break down the misconceptions surrounding NLU and appreciate its true potential. NLU is a remarkable field that combines technology and language understanding, paving the way for more intelligent and effective human-machine communication.
Advantages of NLU
Now, let's explore some of the key advantages of Natural Language Understanding (NLU) and how it can positively impact businesses and users alike.
Improved Customer Service
With NLU, businesses can offer faster and more accurate customer service. NLU-powered chatbots and virtual assistants can understand user intents, provide relevant information, and handle common inquiries efficiently. This reduces the need for customers to wait for human assistance and provides them with instant support, resulting in improved customer satisfaction and loyalty.
Enhanced User Experience
NLU enables a more natural and intuitive user experience. Instead of navigating complex menus or filling out forms, users can engage in spoken or written dialogue, just like they would with a human. NLU models can interpret user queries and intents, generating appropriate responses and taking relevant actions. This conversational approach enhances user engagement and makes interactions more enjoyable and personalized.
Cost Savings
Implementing NLU can lead to significant cost savings for businesses. By automating routine inquiries and tasks, NLU-powered systems reduce the need for large customer support teams. This allows businesses to reallocate resources to other areas while maintaining efficient customer service. Additionally, automation through NLU can handle multiple interactions simultaneously, leading to scalability and improved operational efficiency.
Challenges of Implementing NLU
While NLU offers numerous advantages, there are also challenges to consider when implementing these systems.
Data Privacy Concerns
NLU relies on processing and analyzing user language data, raising concerns about data privacy. Businesses must ensure that they handle user data in a secure and compliant manner, adhering to relevant privacy regulations. By implementing robust data protection measures and obtaining user consent, businesses can address these concerns and build trust with their customers.
Technical Challenges
Developing and deploying NLU systems can pose technical challenges. Building accurate NLU models requires access to annotated training data, which may be limited or costly for some languages or specialized domains. NLU models also need continuous updating to stay relevant and adapt to changing user behavior. Overcoming these challenges involves investing in data collection, model refinement, and consistent system monitoring to deliver high-quality NLU performance.
Ethical Concerns
Ethical considerations are essential when developing and using NLU systems. It is crucial to ensure that the technology does not perpetuate biases or discriminate against certain groups. NLU models must be trained on diverse datasets and subject to rigorous evaluation to minimize biases and promote fair and unbiased outcomes. Ongoing research and collaboration within the AI community can help address these ethical concerns and encourage responsible implementation of NLU.
Future Trends and Innovations in NLU
As Natural Language Understanding (NLU) continues to evolve, let's explore some of the exciting trends and innovations that lie ahead.
Improved Multilingual Capabilities
NLU is becoming increasingly multilingual, allowing systems to understand and interpret a wide range of languages. Ongoing research is focused on improving the performance of NLU models in languages with limited training resources. This progress will enable businesses to offer NLU-powered interactions in diverse languages, connecting with users globally and fostering inclusivity.
Advancements in Context Understanding
One of the key challenges for NLU systems is understanding the context of a conversation. Future innovations in NLU will emphasize context modeling, allowing systems to better grasp the nuances of user queries within a conversation. By enhancing context understanding, NLU models can provide more accurate and personalized responses, leading to even more engaging and efficient interactions.
Integration with other AI Technologies
NLU's integration with other AI technologies will unlock new possibilities. Combining NLU with Natural Language Generation (NLG) can create a seamless dialogue, where systems not only understand but also generate natural language responses. Embracing NLU in conjunction with other AI approaches, such as computer vision and machine learning, will lead to more comprehensive and capable AI systems. It can understand and interact with humans in a more human-like way.
NLU Applications in Industry
NLU is finding its way into various industries, transforming the way businesses and users engage. Let's explore some specific applications across different sectors.
Healthcare
In the healthcare industry, NLU holds enormous potential. Chatbots powered by NLU can assist patients in scheduling appointments, providing basic medical information, and answering common health-related questions. NLU also enables the analysis of patient sentiment and feedback, helping healthcare providers enhance the overall patient experience. Incorporating NLU in healthcare systems contributes to more efficient and accessible healthcare services, improving patient outcomes.
Customer Service
NLU has already made a significant impact on customer service. Chatbots and virtual assistants equipped with NLU can handle customer inquiries, provide personalized recommendations, and assist with common troubleshooting. This automation streamlines customer support processes, reduces wait times, and liberates human agents to focus on complex cases. NLU-powered customer service provides businesses with a scalable and efficient way to deliver exceptional support while maintaining cost-effectiveness.
E-commerce
NLU is revolutionizing the e-commerce landscape. By understanding and interpreting user queries and preferences, NLU-powered systems can deliver highly personalized product recommendations and tailored shopping experiences. Additionally, chatbots equipped with NLU can guide users through the purchase process, answer product-related questions, and provide real-time assistance, replicating the personalized support of an in-store shopping experience.
Banking and Finance
NLU plays a vital role in the banking and finance industry. Virtual assistants powered by NLU can help customers with account inquiries, provide personalized financial insights, and assist with transactions. NLU also enables sentiment analysis, allowing financial institutions to gauge customer satisfaction and proactively address issues. Leveraging NLU in banking and finance enhances customer experiences, simplifies processes, and improves operational efficiency.
Conclusion
As the introduction covered, widespread myths and hype around NLU capabilities often fuel premature adoption and sub-optimal results from conversational AI investments as per survey data (Federico Bianchi, 2023). By clarifying strengths alongside limitations around multi-turn dialog management, sentiment detection accuracy and model transparency, organizations can calibrate expectations and make informed vendor selections.
Specifically, Businesses must evaluate NLU solution readiness across key pillars like breadth of semantic understanding, inference latency thresholds, explanation provision for predictions and scalability infrastructure before integration rather than assuming generalized competency. As per Gartner, 87% of language AI projects demanding real-time engagement fail from inadequate assessment (2023).
Platforms such as BotPenguin counter poor evaluations by offering configurable NLU pipelines tailored to enterprise needs. BotPenguin's MorphNLUTM engine allows combining modular neural functions like named entity recognition, intent classification and dialog state tracking for customized language capabilities targeting defined use cases. Such flexibility coupled with performance benchmarking and transparency around model provenance facilitates suitability-based deployment minimizing disillusionment.
With conversational AI projected to reach $42B in market size by 2030, establishing NLU competence barriers via responsible education will uplift delivery success as more organizations seek transforming digital experiences.
Frequently Asked Questions (FAQs)
What is NLU and how does it work?
NLU (Natural Language Understanding) is a branch of AI that focuses on machine understanding and interpretation of human language. It utilizes algorithms and models to process text and derive meaning from it.
Can NLU understand and interpret different languages?
Yes, NLU systems are designed to comprehend multiple languages. They use language models and techniques to analyze and interpret the meaning of text in various languages.
Are NLU models capable of understanding context and nuance in language?
Yes, NLU models are trained to understand the context and nuances of language. They use techniques like semantic analysis, sentiment analysis, and named entity recognition to interpret text accurately.
Is NLU able to handle complex and ambiguous language?
NLU models are built to handle complex and ambiguous language by utilizing techniques like disambiguation and context analysis. They aim to accurately understand and interpret the intended meaning behind such language.
Does NLU have limitations in understanding sarcasm, irony, or humor?
While NLU models have improved in their understanding of sarcasm, irony, and humor, their performance may vary. These models rely on contextual cues and training data, so their interpretation of such language may not always be perfect.
Can NLU accurately handle user queries and provide relevant responses?
NLU algorithms strive to accurately understand user queries and provide relevant responses. However, the performance of NLU systems can depend on factors like training data quality and the complexity of the query being posed.