Natural Language Processing (NLP) is a transformative field intersecting linguistics, artificial intelligence, and computer science.
Did you know that NLP is revolutionizing the way humans interact with technology? NLP enables computers to comprehend, interpret, and respond to human language. It's everywhere, from virtual assistants like Siri and Alexa to language translation apps.
According to Statista, the global NLP market will reach $23.68bn in 2023 and $31.9 billion by 2027. The NLP market is growing at a CAGR of 19.8% from 2022 to 2027. These statistics show how NLP is the future of the next generation.
But what exactly is NLP? Well, think of it as teaching computers to speak and understand our language just like we do. NLP is like a superpower that allows computers to comprehend sentiment, recognize entities, and generate human-like responses.
With NLP advancements, AI applications are becoming smarter, more intuitive, and better at understanding human language nuances.
So continue reading to find out more about Natural Language Processing. In this blog, you will find NLP's history, basics, applications, and future.
What is Natural Language Processing?
Natural Language Processing, or NLP, is a field of AI that enables computer programs to understand, interpret and generate human language.
In simple terms, NLP involves developing algorithms that can work with human language data. It can perform sentiment analysis, language translation, and speech recognition.
In 2023, NLP's landscape will be full of remarkable advancements. Cutting-edge models like GPT-3 have pushed the boundaries of language understanding. It enables tasks such as context-rich text generation and emotion analysis.
What is the Importance of NLP in Today's Technological Landscape?
NLP is a critical and growing field with applications in multiple industries. It includes healthcare, finance, marketing, and customer service. The ability of computer programs to understand and interpret human language is becoming increasingly important as more data gets generated daily.
NLP is also important for communication between individuals and organizations that don't share the same language. It aids in creating a globalized world with no communication barriers.
How is NLP Transforming Various Industries?
NLP finds applications across industries and transforms them. Let's see how NLP does it:
Healthcare
NLP makes it easier for healthcare providers to make sense of patient data in healthcare.
With the increasing amount of medical information, NLP is aiding in extracting relevant information from patient records and using that data to identify and analyze trends in patient outcomes.
According to MarketsandMarkets, the healthcare industry is the largest end-user of NLP. It accounts for 30% of the global NLP market in 2023.
To further boost the NLP in transforming your healthcare business, you can use chatbots by BotPenguin.
Customer Service
NLP is used extensively in developing chatbots that can provide customer service 24/7. With their ability to understand and respond to customer queries, chatbots are an inexpensive solution that saves customers time and effort.
BotPenguin studies show that using NLP and AI in customer service is growing rapidly. In 2023, over 60% of customer service interactions will get handled by chatbots or other AI-powered systems.
Marketing
NLP-based systems have their uses in personalization and customization. It is a critical factor in the success of marketing campaigns. They sift through data to determine customers' likes or dislikes and tailor product recommendations to their needs. It assists in scoring high customer satisfaction and loyalty.
Finance
The finance industry has started using NLP in fraud detection. It also analyzes news and social media sentiment to make better investment decisions.
NLP is also used in chatbots, automating conversations with customers. As per MarketsandMarkets studies, the financial services industry is the second largest end-user of NLP.
It accounted for 21% of the global market in 2022.
Take it further and integrate BotPenguin chatbots with rich language translation and sentiment analysis.
Basics of Natural Language Processing
In this section, we'll dive into the basics of NLP, exploring its components and the different techniques and algorithms that make it all possible.
Understanding the Components of NLP
NLP consists of three interconnected components that work together to process and understand human language. Let's take a closer look at these NLP components:
Tokenization
Tokenization breaks down a simple text message into smaller units, such as sentences or words. By breaking down the text into these chunks, computers can more easily understand and analyze the language.
Part-of-Speech Tagging
Part-of-Speech (POS) tagging involves assigning tags to words in a sentence. It includes nouns, verbs, adjectives, and more.
Part-of-Speech training helps chatbots understand the grammatical structure of a sentence. In turn, it can be helpful for tasks like text analysis and language translation.
Named Entity Recognition
Named Entity Recognition, or NER, identifies and classifies named entities into a text. It includes person names, locations, organizations, and more. NER is helpful in various ways, like information extraction and question-answering systems.
Exploring the Different Techniques and Algorithms Used in NLP
NLP utilizes various techniques and algorithms to process and interpret human language. Here are a few notable ones:
Sentiment Analysis
Sentiment analysis is a technique that aims to determine the sentiment or emotion expressed in a piece of text. By analyzing the idea, computers can gain insights into public opinion and customer feedback.
Sentiment analysis is helpful for social media monitoring and marketing analysis. It can identify positive, negative, or neutral sentiments in customer feedback.
NLP models use various techniques like rule-based systems and machine learning to perform sentiment analysis.
Machine Translation
Machine translation is all about translating text from one language to another automatically. With the help of NLP algorithms, computers can analyze the structure of the source language.
Then, it can generate equivalent sentences in the target language.
Machine translation is helpful for a wide range of applications, from language learning to global business.
Machine translation NLP models rely on various techniques, such as statistical methods and neural networks, to accurately translate text.
Text Summarization
Text summarization algorithms can automatically create concise summaries of longer texts.
It is beneficial while dealing with large amounts of data in the forms of text. It allows for quick extraction of key information.
Language Generation
Language generation involves generating human-like text. Chatbots, virtual assistants, and creative writing applications use Language generation techniques.
Computers can generate coherent and contextually relevant text, mimicking the style and tone of human language.
NLP Applications in Everyday Life
In this section, we will explore some exciting applications of NLP that you may encounter regularly.
-min.jpg)
NLP in Virtual Assistants and Chatbots
NLP is like the basis of the foundation for building virtual assistants and chatbots. It has direct applications in everyday life, like:
Virtual Assistants
Virtual assistants like Siri, Alexa, and Google Assistant have become integral parts of people’s lives. These AI-powered assistants rely on NLP to understand our voice commands and respond accordingly.
Whether you're asking them to play your favorite song or provide weather updates, NLP algorithms make it possible for virtual assistants to process and interpret human language.
Chatbots
Have you ever interacted with a chatbot? Chatbots understand customer queries and provide relevant information or assistance with the help of NLP. They can handle common inquiries, guide users through processes, and even mimic human-like conversations.
Thanks to NLP, chatbots, like BotPenguin, are available round the clock, providing instant support and saving us valuable time.
NLP in Language Translation
Language translation apps and services rely on NLP techniques. As it automatically translates text from one language to another.
NLP algorithms can generate accurate translations by analyzing sentence structure, grammar, and context.
It allows us to communicate with people who speak different languages, making the world more connected.
NLP in Sentiment Analysis for Social Media
Have you ever wondered how companies know what people say about their brand on social media? NLP algorithms can analyze and classify social media posts to determine sentiment and public opinion.
This information helps companies understand customer feedback, identify trends, and make better business decisions.
NLP in Spam Detection and Email Filtering
NLP algorithms play a crucial role in detecting and filtering spam emails. By analyzing the content and structure of emails, NLP can flag suspicious messages and prevent them from cluttering our inboxes.
It ensures that we only see the emails that matter and protects us from potential scams.
NLP in Voice Recognition and Voice Assistants
NLP empowers voice recognition systems to transcribe and understand spoken language accurately. Whether dictating a text message or giving voice commands to our devices, NLP algorithms convert spoken words into actionable information.
Voice assistants like Siri and Google Assistant rely on NLP to process and respond to human queries. NLP makes interaction with technology more intuitive.
Suggested Reading:
Transforming the Recruitment Process With NLP
Challenges in Natural Language Processing
Natural Language Processing allows applications to understand and process human language. However, it has its challenges. So, let's see the challenges:
Ambiguity in Language
One of the biggest challenges in NLP is word ambiguity. Many words in natural language have multiple meanings, and determining the correct interpretation can be tricky.
For instance, "bank" refers to a financial institution and the edge of a lank or river. NLP algorithms need additional contextual information to understand the intended meaning accurately.
Lack of Context
Understanding the context of a sentence or word plays a vital role in NLP. With context, it's easier for computers to interpret natural language accurately.
For example, "I saw her duck" can have two completely different meanings depending on whether "duck" refers to the bird or the action of avoiding. Incorporating contextual information is an ongoing challenge in NLP.
Special Language Forms and Variations
The natural language contains slang, jargon, and variations based on dialects and regions. It challenges NLP algorithms to understand and process these linguistic nuances without training.
For example, understanding informal language or deciphering text filled with abbreviations can be difficult for NLP systems.
Data Limitations
NLP algorithms require large amounts of high-quality data to get proper training. However, obtaining such data is a challenge.
Accurate and diverse training data is necessary to ensure NLP models can handle various language inputs.
Additionally, labeled data for specific domains or languages may be limited. It is challenging to build specialized NLP systems.
Language Evolution
Languages evolve, and new words, phrases, and grammatical constructions emerge. It poses a challenge for NLP algorithms, as they may need help understanding or processing language without proper training.
Keeping NLP models up to date with the latest language trends is an ongoing task for researchers and developers.
Sentiment Analysis with NLP
Have you ever wondered how chatbots can understand the emotions behind the text? Well, it's all thanks to Sentiment Analysis, a powerful Natural Language Processing (NLP) technique. It enables machines to determine a text's sentiment or emotional tone.
In this section, we'll see more about Sentiment Analysis.
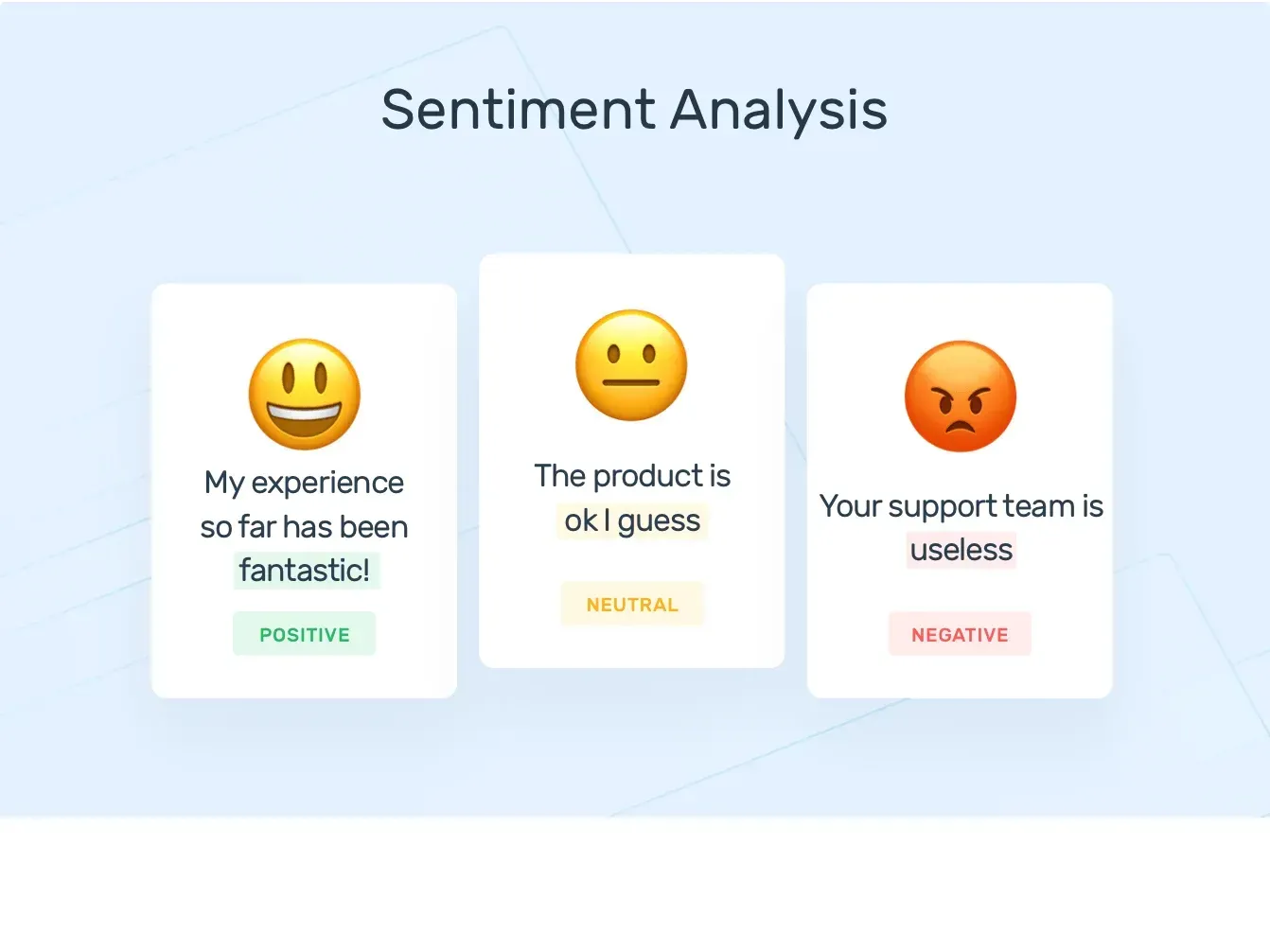
What is Sentiment Analysis?
Sentiment Analysis, or emotion-opinion mining, is the process of identifying and classifying the emotional tone in a piece of text. It helps us understand whether the text expresses positive, negative, or neutral sentiments. We can gain valuable insights into customer feedback, social media trends, and more by analyzing sentiment.
Techniques Used in Sentiment Analysis
These are the two techniques used in Sentiment analysis:
Rule-Based Systems
Some Sentiment Analysis models use predefined rules to recognize specific words or patterns associated with emotions. These systems classify text based on these rules but may need help with new or nuanced expressions.
Machine Learning
Other Sentiment Analysis models utilize machine learning algorithms to learn from labeled data. These models can recognize patterns and make predictions based on the ways they've learned.
With the correct training data, they can provide accurate sentiment analysis results.
Challenges of Sentiment Analysis
These are the two challenges of Sentiment analysis:
Contextual Understanding
One of the biggest concerns in Sentiment Analysis of NLP is understanding the context of a written text.
One word can have two different meanings in different contexts. And machines need to interpret the context to determine sentiment accurately.
Sarcasm and Irony
Detecting sarcasm or irony in the text is challenging for Sentiment Analysis models. These expressions often require a deeper understanding of language and context. It is trickier for machines to pick up on these subtle cues.
Applications of Sentiment Analysis
These are the two applications of Sentiment analysis:
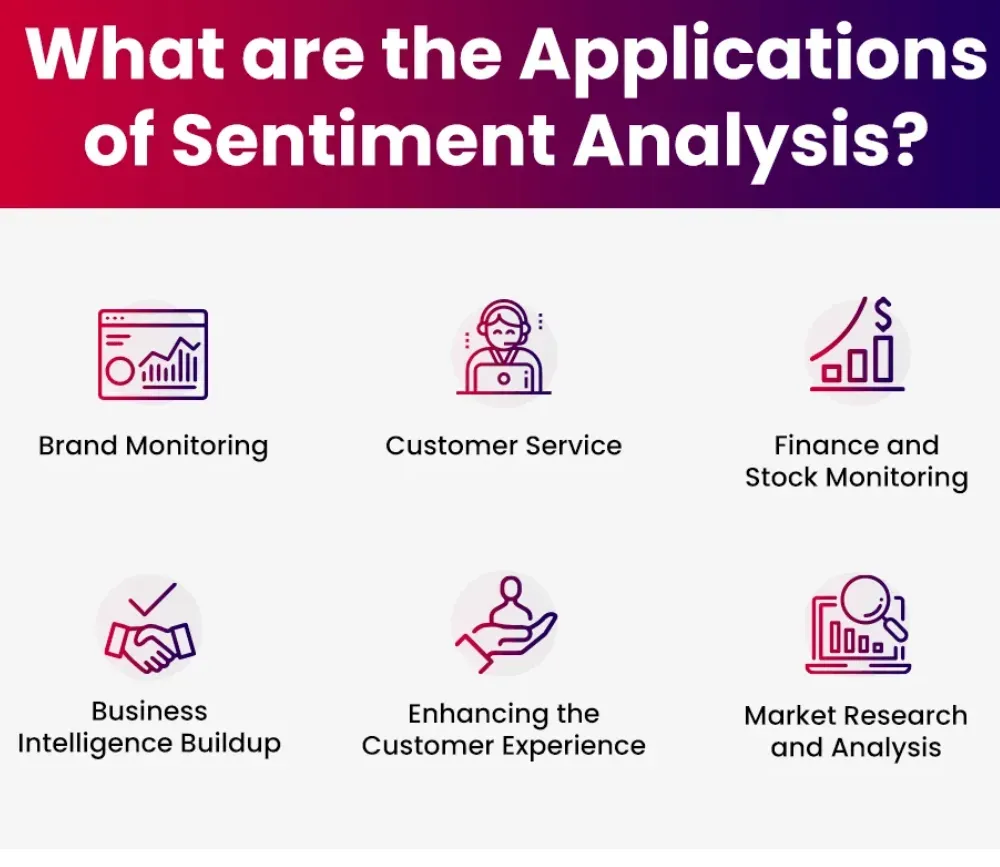
Customer Feedback Analysis
Companies use Sentiment Analysis to analyze customer feedback, such as reviews or social media comments. By understanding the sentiment behind customer opinions, businesses identify the areas for improvement. Thus, it aids in making more data-driven decisions.
Social Media Monitoring
Sentiment Analysis is also widely used for social media monitoring. It can be about a product, brand, or event in real-time and adjust their strategies accordingly.
Ethical Considerations in NLP
This section explores NLP's ethical challenges and how to ensure its fair and responsible uses.
Bias in NLP
NLP systems are only as unbiased as the data they use for training. Unfortunately, bias can be inherent in the data sources and their labeling, leading to biased models.
NLP must be developed with diverse perspectives to avoid perpetuating biases and stereotypes.
Privacy in NLP
NLP models can extract sensitive personal data from text, raising privacy concerns.
Developers must ensure that users' personal information is protected. And that NLP systems only collect data necessary for their intended purposes.
Transparency in NLP
NLP has far-reaching effects on individuals and society as a whole. Therefore, it's essential to be transparent about how NLP models work. It is crucial to show their trained data source and how the models make decisions.
Transparency ensures that the benefits and risks of NLP are clearly understood. And the NLP technology is used responsibly.
Accountability in NLP
NLP systems must be accountable for the decisions they make and the outcomes they produce. When deploying NLP models, monitoring their performance and promptly addressing any issues is essential.
End-users must have a clear path to appeal or report any outcomes they believe are unfair or discriminatory.
Suggested Reading:
Natural Language Processing Consulting: The Complete Guide
Meet BotPenguin: Your Way To Leverage NLP To Skyrocket Your Business
You just learned all about natural language processing and how powerful it is!.
Now, how exactly to put NLP into action to grow your business?
With BotPenguin's AI chatbots, you totally can!
Our bots use advanced NLP techniques so they can have super helpful, human-like conversations.
You can use our NLP chatbots to understand customers better, automate support 24/7, and make data-driven decisions. And we designed BotPenguin to be simple even for non-techies.
Numerous businesses are using our chatbots to boost results with NLP.
Ready to leverage NLP and take your business to new heights? Sign up today!
Frequently Asked Questions (FAQs)
How does NLP work?
NLP uses algorithms and computational linguistics to process and analyze vast amounts of textual data. It involves tasks like tokenization, part-of-speech tagging, syntactic parsing, and semantic analysis to comprehend and extract meaning from text.
What are the applications of NLP?
NLP has diverse applications, including sentiment analysis, machine translation, chatbots, voice assistants, text summarization, language modeling, and information retrieval. It has uses in healthcare, finance, customer service, and social media analytics.
How does NLP improve chatbots and virtual assistants?
NLP enhances chatbots and virtual assistants by enabling them to understand, intercept and respond to user queries more humanistically. It allows for natural language interactions, improving user experience and engagement.
Can NLP understand multiple languages?
Yes, NLP supports multiple languages through techniques like machine translation and language modeling. Advanced NLP models are trained on multilingual datasets to comprehend and generate text in various languages.
How does NLP contribute to language translation?
NLP facilitates language translation by using machine translation algorithms. It analyzes and translates text from one language to another, enabling seamless communication across linguistic barrier
How is NLP transforming industries?
NLP is revolutionizing industries by automating language-related tasks, improving customer service with chatbots, extracting insights from text data, and enabling personalized content recommendations. Its impact shows in healthcare, finance, e-commerce, and other sectors.