Natural language processing (NLP) is revolutionizing how we interact with technology. Two powerhouse models stand out - Google's Flan-T5 and OpenAI's GPT-3.
These language giants can understand and generate human-like text with remarkable prowess.
But which one reigns supreme?
In this blog post, we explore Flan-T5's capabilities, strengths, and limitations. We explore how Flan-T5 excels at complex language understanding across multiple tasks, such as translation and summarization. We witness GPT-3's unparalleled text generation skills, which blur the line between human and machine.
From real-world applications to ethical considerations, we leave no stone unturned. Discover how these models are transforming industries like content creation, conversational AI, and machine translation.
But the journey doesn't end there. We'll address these models' challenges, from potential biases to the need for immense training data. Get insights into the future of NLP and how Flan-T5 and GPT-3 are shaping it.
Get ready to embark on an enlightening exploration of the cutting-edge in natural language processing. The battle between Flan-T5 and GPT-3 awaits!
Understanding Flan-T5
Flan-T5 is a natural language processing model developed by Google that aims to comprehend and generate human-like text.
Its fundamental concept revolves around deep learning techniques applied to large-scale language training.
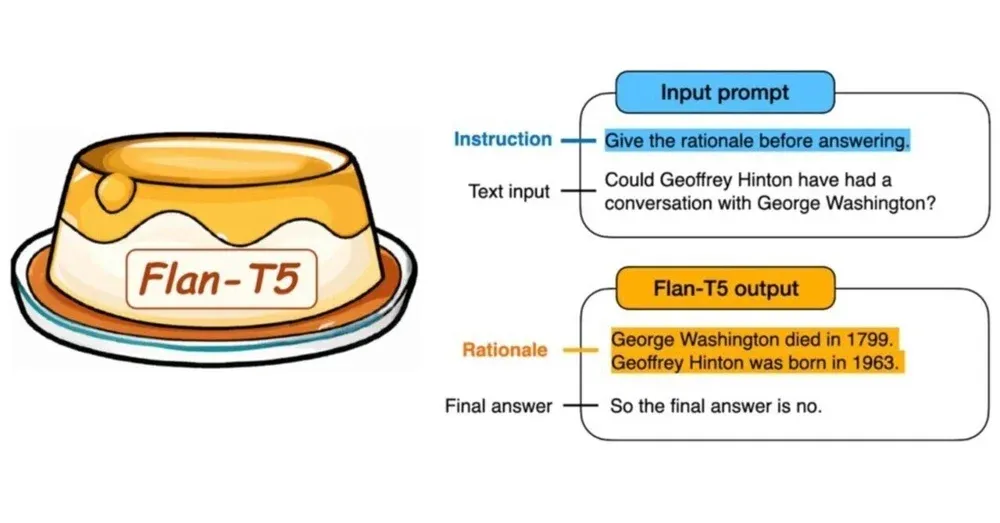
Key Features of Flan-T5
- Powerful Language Understanding: Flan-T5 demonstrates exceptional understanding of complex linguistic structures and contextual information, making it a reliable choice for a wide range of natural language processing (NLP) tasks.
- Versatile Multitasking: This model is designed to handle multiple NLP tasks simultaneously, including translation, question-answering, summarization, and sentiment analysis. Developers can leverage this multitasking capability to build more efficient and versatile NLP applications.
- Transfer Learning Capability: Flan-T5 incorporates transfer learning, allowing it to acquire knowledge from pre-trained models. This capability enables the model to adapt to specific domains or tasks with minimal fine-tuning, thus saving time and resources.
- Efficient Computation: Flan-T5 optimizes computation resources, resulting in faster processing times and scalability. This efficiency makes it suitable for both small-scale and large-scale projects.
Reasons for Popularity
Flan-T5 has gained substantial popularity among developers and researchers for several reasons.
Firstly, the model has access to vast training data, enabling it to capture diverse linguistic patterns and nuances effectively. This helps developers create applications that better understand and interpret human language.
Secondly, Flan-T5's powerful language understanding capabilities contribute to its popularity. The model's ability to comprehend and generate text allows for the creation of highly sophisticated and accurate NLP applications.
Lastly, Google's reputation for delivering high-quality and dependable technologies elevates Flan-T5's appeal. Developers trust Google's expertise and rely on Flan-T5 for their NLP projects, further driving its popularity in the community.
Next, we will explore about GPT-3.
Exploring GPT-3
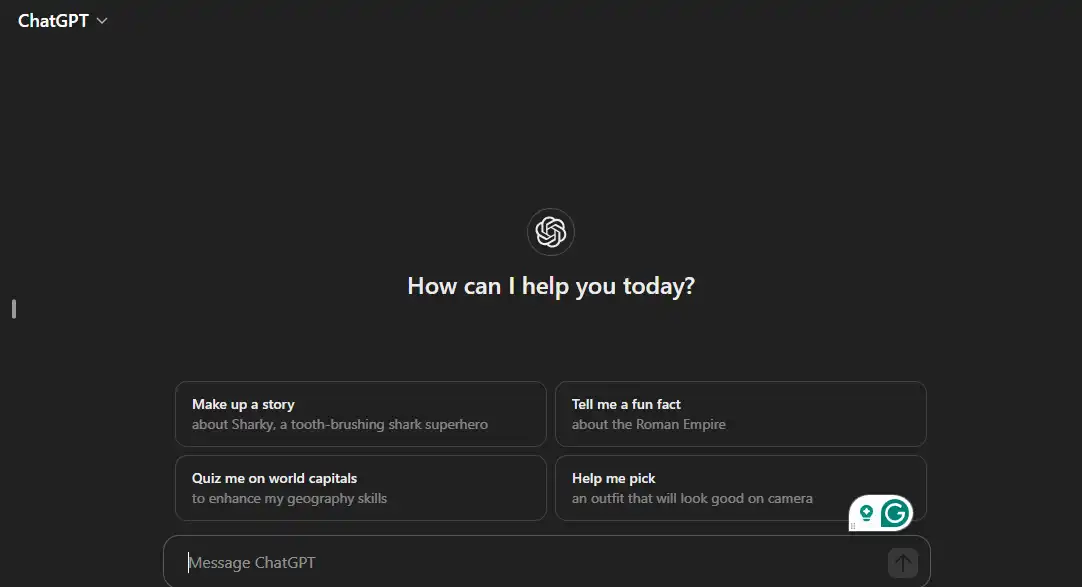
GPT-3, developed by OpenAI, represents "Generative Pre-trained Transformer 3," and it revolutionizes the field of natural language processing with its remarkable capabilities.
Overview of GPT-3 and its Capabilities
GPT-3 is an advanced language model known for its impressive generation of human-like text.
Trained on diverse datasets, it leverages the power of deep learning to comprehend and produce relevant responses.
The model's capabilities extend beyond simple pattern-matching, allowing it to generate contextually coherent and creative text.
Advantages and Unique Aspects of GPT-3
- Language Generation Par Excellence: GPT-3's primary strength lies in its ability to generate high-quality, human-like text.
Its language generation capabilities are ideal for content drafting, creative writing, and chatbot interactions. GPT-3 is often praised for producing coherent and contextually appropriate responses.
- Multi-Domain Adaptability: GPT-3 can adapt to different domains, enabling users to train it on specific datasets related to their industry or area of interest.
This flexibility enhances the model's performance and allows it to cater to specialized requirements.
- Unsupervised Learning: GPT-3 is trained unsupervised by learning from vast amounts of internet text data. This training approach enables the model to comprehend and generate text in various contexts, making it highly versatile.
- Few-Shot Learning: GPT-3 exhibits the capability of few-shot learning, performing well with minimal training on specific tasks. This reduces the need for extensive task-specific training and allows for more efficient and rapid development.
The impressive language generation capabilities, multi-domain adaptability, unsupervised learning, and few-shot learning make GPT-3 highly sought-after among developers and researchers in the NLP community.
Reasons for the Popularity of GPT-3
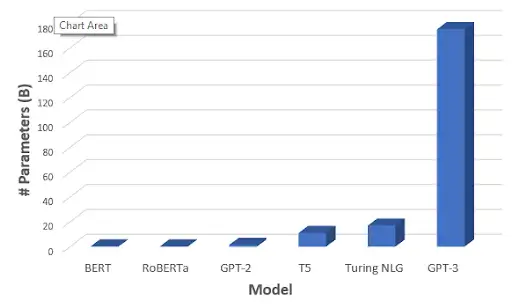
GPT-3's popularity is primarily driven by its ability to generate human-like text that is virtually indistinguishable from human-generated text.
This feature alone has opened up many possibilities for developing applications in content creation, chatbots, language translation, and more.
GPT-3's versatility and adaptability across domains further contribute to its acclaim among developers and researchers.
OpenAI's dedication to democratizing access to GPT-3 by offering API access has led to widespread adoption.
This accessibility allows developers to integrate GPT-3's capabilities into their projects easily.
Overall, GPT-3's advanced language generation, adaptability, and availability have propelled its popularity, making it a game-changer in the field of NLP.
Next, we will compare Flan-T5 and OpenAI GPT-3.
Flan-T5 Vs. OpenAI GPT-3: Comparison of Features
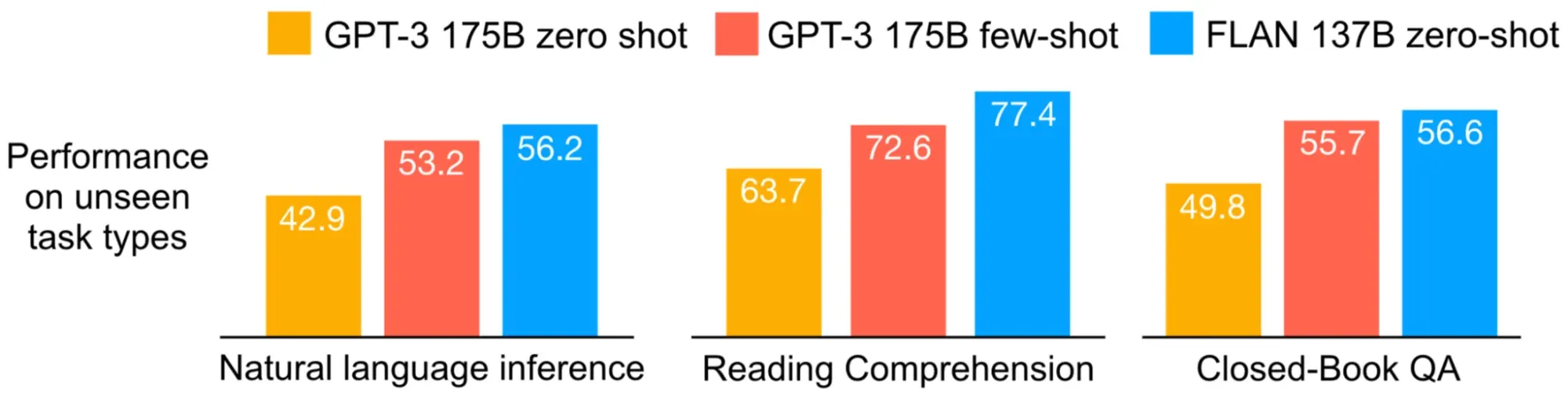
Flan-T5 and GPT-3 are advanced natural language processing models with robust language understanding and generation capabilities. While both models are noteworthy for NLP tasks, they differ significantly.
Flan-T5's powerful language understanding capability and multitasking make it ideal for translation, summarization, question-answering, and other NLP applications.
Its ability to adapt to specific domains with minimal fine-tuning and optimize computation resources results in faster processing times and scalability.
However, while still impressive, Flan-T5's language generation capability does not match GPT-3's ability.
On the other hand, GPT-3's generation capabilities are among the best currently available. Its unsupervised learning approach and multi-domain adaptability make it ideal for content generation, chatbot interactions, and creative writing applications. Its few-shot learning capability has shown promising results.
In summary, Flan-T5 is ideal for NLP tasks that require robust language understanding, multitasking, and efficiency, whereas GPT-3 is ideal for NLP tasks that require exceptional language generation capabilities.
Next, we will see the applications and use cases of Flan-T5 and OpenAI GPT-3.
Flan-T5 Vs. OpenAI GPT-3: Applications and Use Cases
Flan-T5 and GPT-3 have numerous real-world applications across industries.
Flan-T5 Use Cases
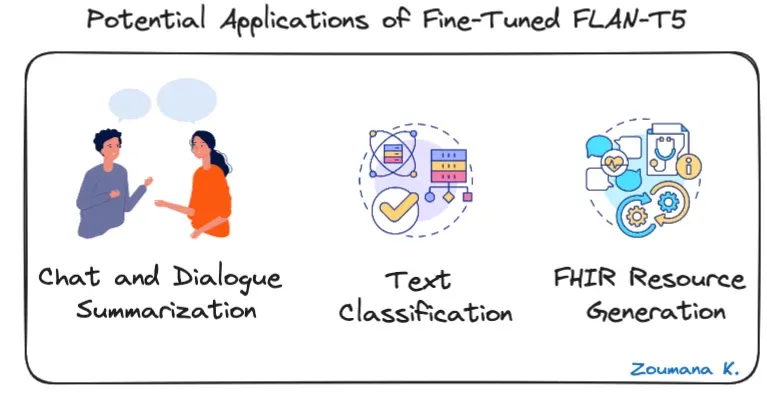
- Translation: Flan-T5's multitasking capability and robust understanding make it ideal for translation applications. Flan-T5 has already shown promising results in machine translation testing.
- Summarization and Question-Answering: Flan-T5's multitasking capability makes it ideal for generating highly efficient summaries and answering complex questions.
- Sentiment Analysis and Chatbots: Flan-T5's efficient computation makes it ideal for processing large volumes of data. This efficiency and its ability to understand contextual information make it suitable for sentiment analysis and chatbot applications.
GPT-3 Use Cases
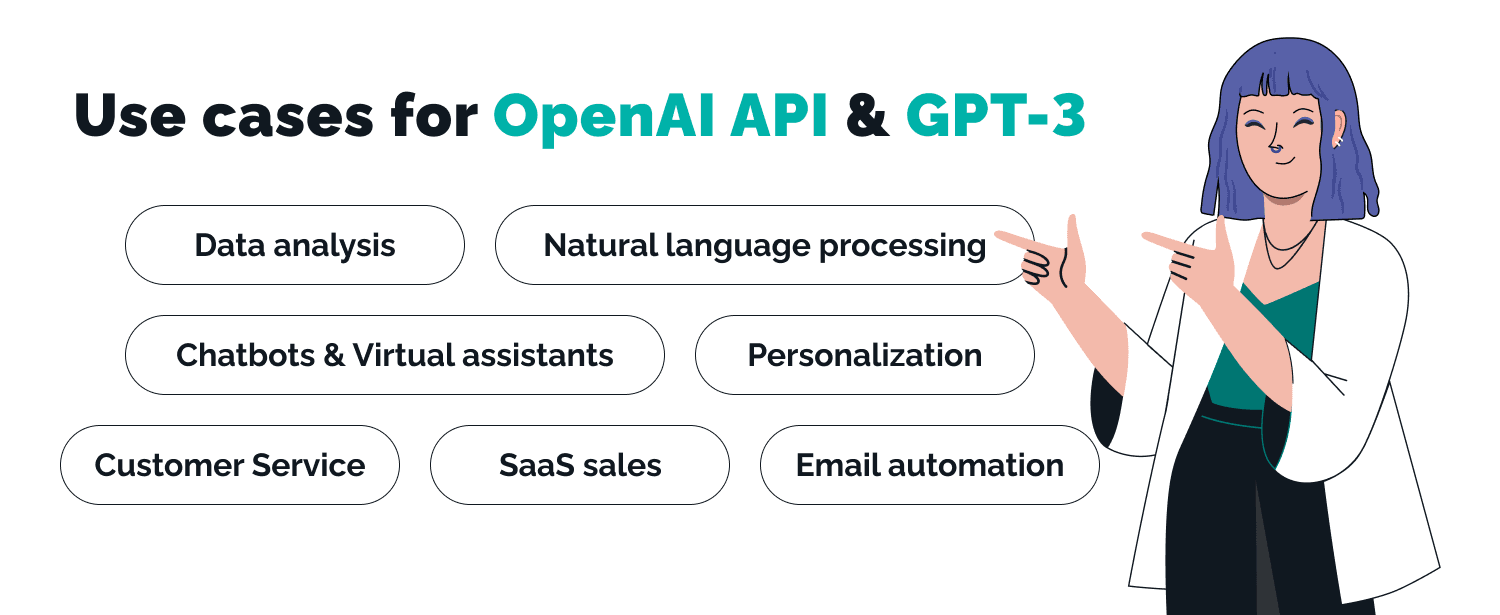
- Content Generation and Creative Writing: GPT-3's powerful language generation capability makes it ideal for content generation and creative writing applications.
Its ability to generate contextually appropriate responses has also made it suitable for content creation tools.
- Chatbots and Conversational Agents: GPT-3's language generation capability is ideal for chatbot and conversational agent applications.
GPT-3's few-shot learning capability has already shown impressive results in chatbot testing.
- Language Translation: GPT-3's excellent language generation capability makes it a promising tool in language translation applications.
While still under testing, GPT-3 has shown promising results in machine translation.
Suggested Reading:
OPT-175B vs GPT-3: Which LLM is Better?
Limitations and Challenges of FLAN-T5 and GPT-3
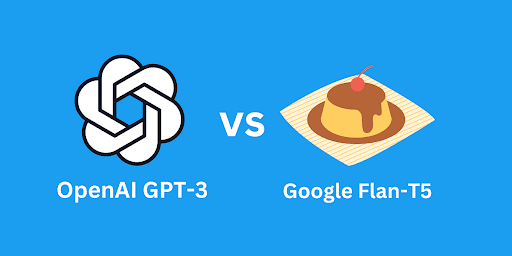
While Flan-T5 and GPT-3 exhibit impressive capabilities, it is essential to acknowledge their limitations and address the challenges associated with their use in natural language processing (NLP) tasks.
Language Generation Bias and Ethical Concerns
One of the critical challenges faced by both models is the potential for bias in language generation.
As these models are trained on vast amounts of data from the Internet, they may inadvertently learn biases present in the training data.
This can result in the generation of biased or discriminatory text. Ethical concerns arise when these models propagate or amplify biases, potentially leading to unintended consequences or reinforcing existing inequalities.
Insufficient Understanding of Context
Despite their language generation capabilities, both Flan-T5 and GPT -3 have limitations in fully understanding complex contextual information.
While they can generate coherent and contextually appropriate responses, they may struggle with nuanced interpretation and lack a deep understanding of underlying concepts.
This limitation can pose challenges in certain NLP tasks that require a higher level of contextual comprehension.
Suggested Reading:
How to Create AI-driven Chatbot Solutions with FLAN-T5
Dependence on Large-Scale Training Data
An inherent challenge with powerful language models like Flan-T5 and GPT-3 is their dependence on large-scale training data.
These models usually require access to vast amounts of diverse and high-quality data to achieve optimal performance.
However, gathering such datasets can be time-consuming and resource-intensive, especially for organizations or researchers without extensive resources.
Ethical Concerns and Potential Risks
The use of powerful NLP models like Flan-T5 and GPT-3 also raises ethical concerns and potential risks.
Generating text that is indistinguishable from human-generated text may lead to misinformation or malicious use. These models could be leveraged to create deepfake content or spread propaganda.
Additionally, issues related to data privacy and consent arise when working with user-generated data to train these models.
Conclusion
The ongoing competition between Flan-T5 and GPT-3 is truly a sight to behold, as each model demonstrates its remarkable capabilities.
Flan-T5 stands out with its impressive language understanding and multitasking skills, while GPT-3 leaves us in awe with its unrivaled text-generation abilities.
As these giants keep evolving, their influence on our world expands. They're transforming various sectors, from enhancing next-generation chatbots and AI assistants to revolutionizing content creation and machine translation.
The potential applications are boundless, making it an exhilarating time for advancements in natural language processing.
However, we must tread carefully and address the challenges that lie ahead. Issues such as biases, ethical usage, and data privacy need to be at the forefront of our minds as we continue to harness the full capabilities of these language models.
The future of natural language processing is here, and it's a thrilling frontier to explore. So, let's embrace the power of Flan-T5 and GPT-3, but do so with a thoughtful and responsible approach. After all, the journey has only just begun, and the best is yet to come.
Suggested Reading:
Building a ChatGPT Clone With BotPenguin: Step-by-Step Guide
Frequently Asked Questions (FAQs)
What are the key differences between Google's Flan-T5 and OpenAI's GPT-3?
Flan-T5 and GPT-3 differ in their architectures, training data, and applications, impacting their performance and use cases.
Which AI model is more suitable for natural language processing tasks?
GPT-3 excels in natural language processing tasks, showcasing superior language understanding and generation capabilities.
How do Flan-T5 and GPT-3 compare in terms of computational requirements?
Flan-T5 may have different computational requirements than GPT-3, influencing considerations for implementation and scalability.
What industries benefit most from Flan-T5, and which ones favor GPT-3?
Flan-T5 and GPT-3 may find varying levels of applicability across industries; understanding their strengths helps determine the best fit.
Can Flan-T5 or GPT-3 be fine-tuned for specific business needs?
Both models offer varying degrees of adaptability, allowing users to fine-tune them for specific business applications.
What kind of support and documentation do Flan-T5 and GPT-3 provide for developers?
Understanding the available support, documentation, and developer resources is crucial for seamless integration and troubleshooting.