What is Lemmatization?
Lemmatization is a linguistic process that involves reducing words to their base or dictionary form, which is known as a lemma.
This process helps simplify textual analysis by grouping together variants of a word.
Significance of Lemmatization
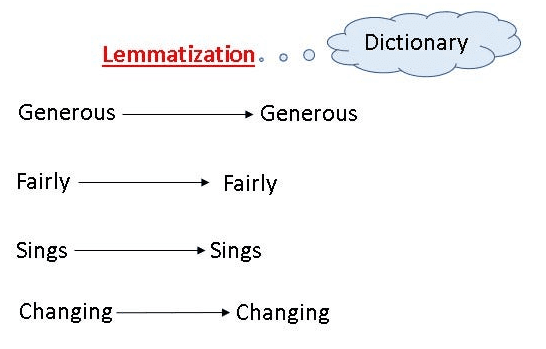
Lemmatization is particularly important in natural language processing (NLP), where it aids in semantic analysis, information retrieval, and text mining. It allows models to understand and process different forms of a word as a single entity.
Lemmatization goes beyond simple word reduction and considers the context of a word in a sentence. It analyzes the part of speech and meaning to accurately convert words to their base form.
A lemmatizer is a software tool or application that conducts lemmatization. It uses a set of rules or a vocabulary of known lemmas to match and transform word forms.
Key Components of Lemmatization
- Lemma: A lemma is the base form or dictionary form of a word. It acts as the standard form under which all variants of a word are grouped.
- Part-of-Speech Tagging: Lemmatization often involves part-of-speech (POS) tagging, which categorizes words based on their function in a sentence (noun, verb, adjective, etc.). This helps ensure accurate lemmatization.
- Morphological Analysis: Lemmatization takes morphological analysis into account, studying the structure of words to identify their roots and affixes.
- Vocabulary: The lemmatizer uses a pre-defined vocabulary of known lemmas as a reference point to transform words to their base form.
Types of Lemmatization
- Rule-Based Lemmatization: Rule-based lemmatization applies a set of predetermined grammatical rules to reduce words. This approach can be fast but may struggle with irregular words.
- Lookup Lemmatization: This type of lemmatization uses a dictionary of known lemmas. Each word is looked up in the dictionary. While it's slower than rule-based, it can handle irregular words better.
- Learning-Based Lemmatization: Here, machine learning models are taught to recognize and lemmatize words. This technique can be versatile and accurate but requires a large amount of annotated training data.
- Hybrid Lemmatization: Hybrid systems combine rule-based, lookup, and learning-based techniques to achieve effective and efficient lemmatization.
Lemmatization versus Stemming
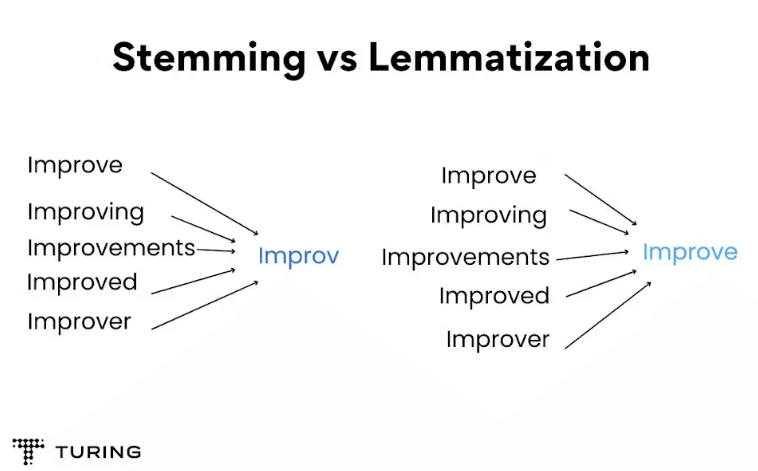
Understanding Stemming
Stemming is a related process to lemmatization, where words are trimmed to their root form. However, stemming doesn't always result in real words and doesn't consider the context.
When to Use Lemmatization Versus Stemming
Use lemmatization when the understanding of the context and accurate reductions are more important. Use stemming when speed is more crucial and incorrect or non-dictionary words are acceptable.
Advantages of Lemmatization
Lemmatization understands the context and the part of speech, resulting in real, meaningful words. It can effectively handle words with different meanings based on the context.
Limitations of Lemmatization
Lemmatization can be slower and computationally more extensive than stemming. It may also require language-specific resources, and the results might be less normalized.
Benefits and Challenges of Lemmatization
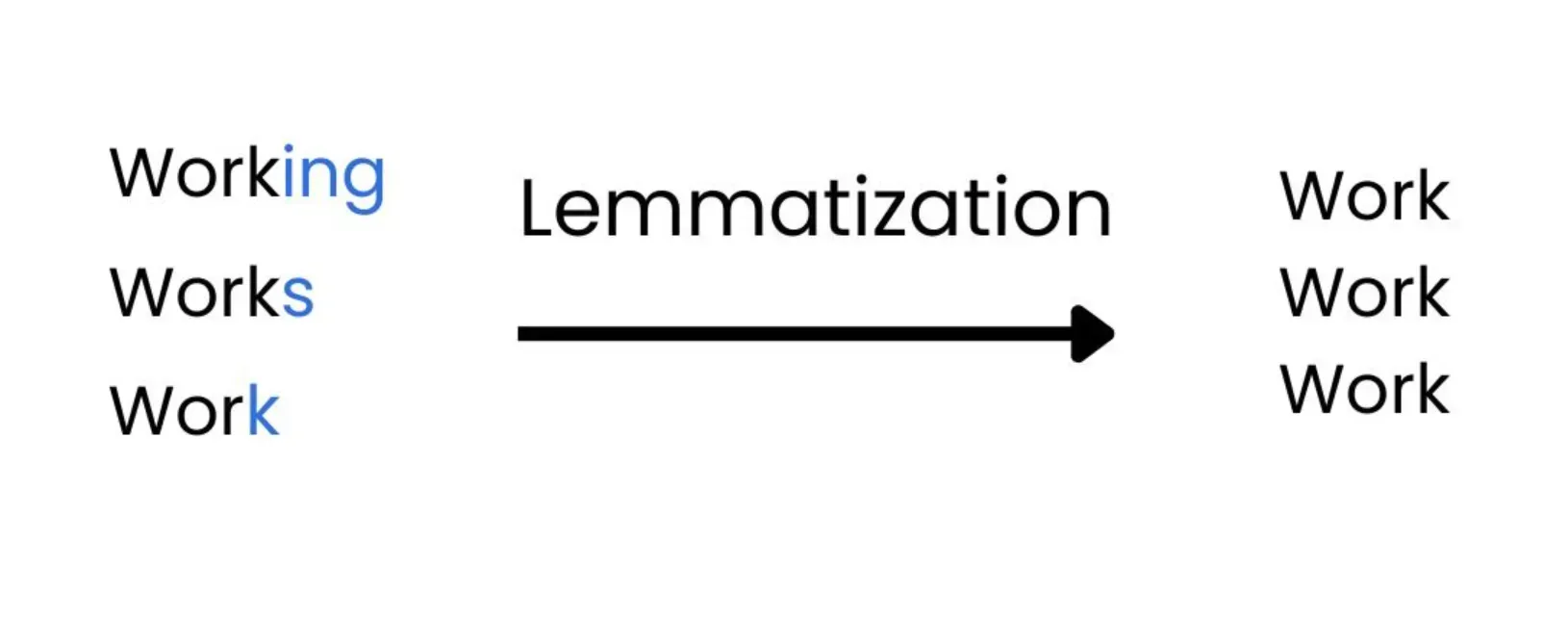
Improved Text Analysis
By reducing words to their lemmas, data becomes more manageable, straightforward, and comparable in text analysis.
Enhanced Information Retrieval
In information retrieval systems, lemmatization can improve search accuracy by treating different forms of a word as a single entity.
Better Natural Language Processing
NLP applications such as machine translation, sentiment analysis, and text summarization can benefit from lemmatization which aids in understanding language semantics.
Consideration of Language and Context
While a benefit, the need for considering the part of speech and context can also be a challenge in lemmatization. Handling homonyms (words with different meanings based on context) is a common difficulty.
Lemmatization in Other Applications
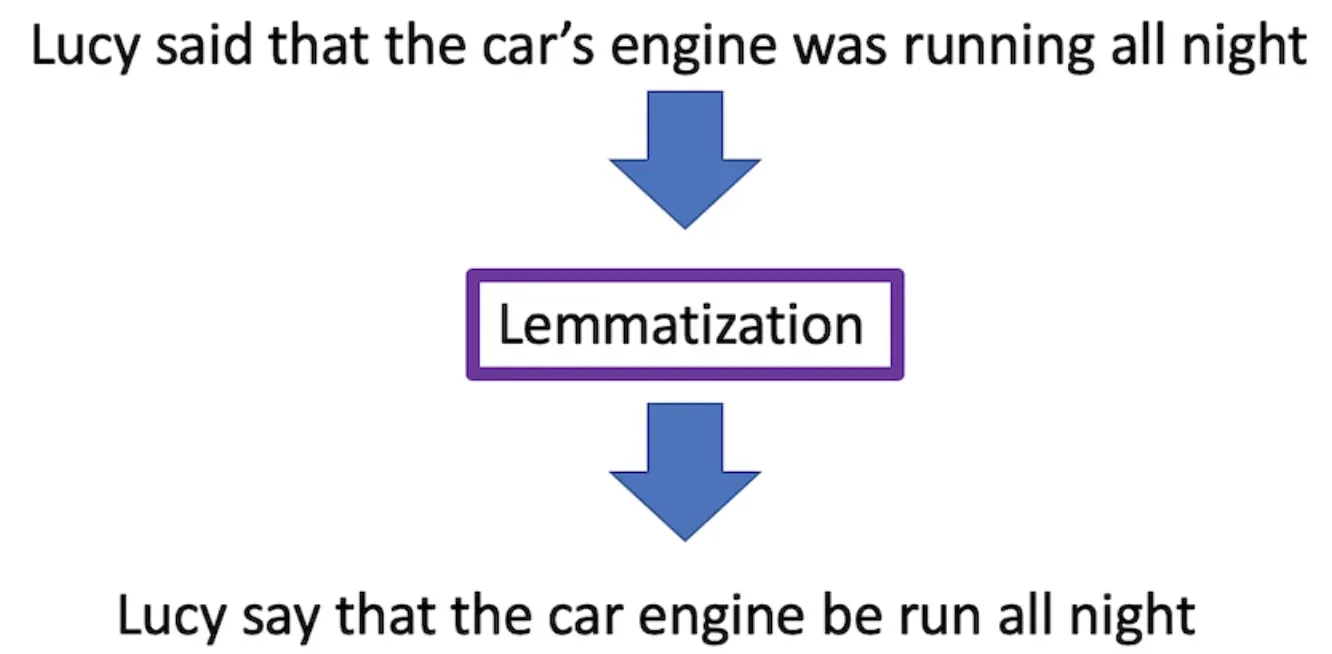
- Search Engines: Search engines use lemmatization to improve search results by considering all forms of a word during a search.
- Sentiment Analysis: Analysing sentiment in text requires an understanding of the context and semantics. Lemmatization aids by simplifying text and improving accuracy.
- Machine Translation: In machine translation, lemmatization helps handle variations in words, improving the accuracy of translations.
- Document Clustering: In document clustering, lemmatization simplifies text, making it easier to identify patterns and themes in test collections.
Frequently Asked Questions (FAQ)
What is lemmatization and why is it important?
Lemmatization is a process in linguistics of reducing words to their base form or 'lemma'.
It is significant in natural language processing, semantic analysis, information retrieval, and text mining as it helps to understand and process disparate forms of a word as the same entity.
How does lemmatization work?
Lemmatization works by analyzing the word's context in a sentence, considering its part of speech and meaning to accurately convert it into its base or dictionary form.
What is the difference between lemmatization and stemming?
Stemming is a more primitive form of word reduction that often results in non-dictionary words, whereas lemmatization is more sophisticated, taking into account the context and part of speech of a word to return meaningful and dictionary-based words.
When should I use lemmatization instead of stemming?
Lemmatization should be used when the understanding of word context and output of accurate, meaningful words matter more in an application.
On the other hand, stemming is useful when speed is more crucial and you can tolerate the output of non-dictionary words.
What are the benefits and challenges of lemmatization?
Lemmatization simplifies text analysis, aids information retrieval, and improves natural language processing.
However, it can be slower and more computationally demanding than stemming. It also requires handling of part of speech and context, and can struggle with handling homonyms.