Introduction
Text-to-speech (TTS) technology has advanced a lot from the beginning, and the methods used in earlier times are now considered outdated.
The invisible hand behind this transformation is Generative AI. To give an idea, the generative AI market is estimated to reach $1.3 trillion by 2032 as per Statista.
Generative AI employs deep learning techniques to generate new content, mimicking human-like behaviors in creating text, images, and even voices. In the TTS, Generative AI offers innovative solutions, allowing for the creation of natural-sounding speech from written text.
The uses of Generative AI for TTS extend beyond chatbots and storytelling.
Its ability to generate expressive and contextually appropriate speech opens avenues for creative expression and communication.
Generative AI plays a pivotal role in advancing TTS technology. So continue reading to know more.
The Evolution of Text-to-Speech Technology
In this section, you’ll find the evolution of Text-to-Speech technology.
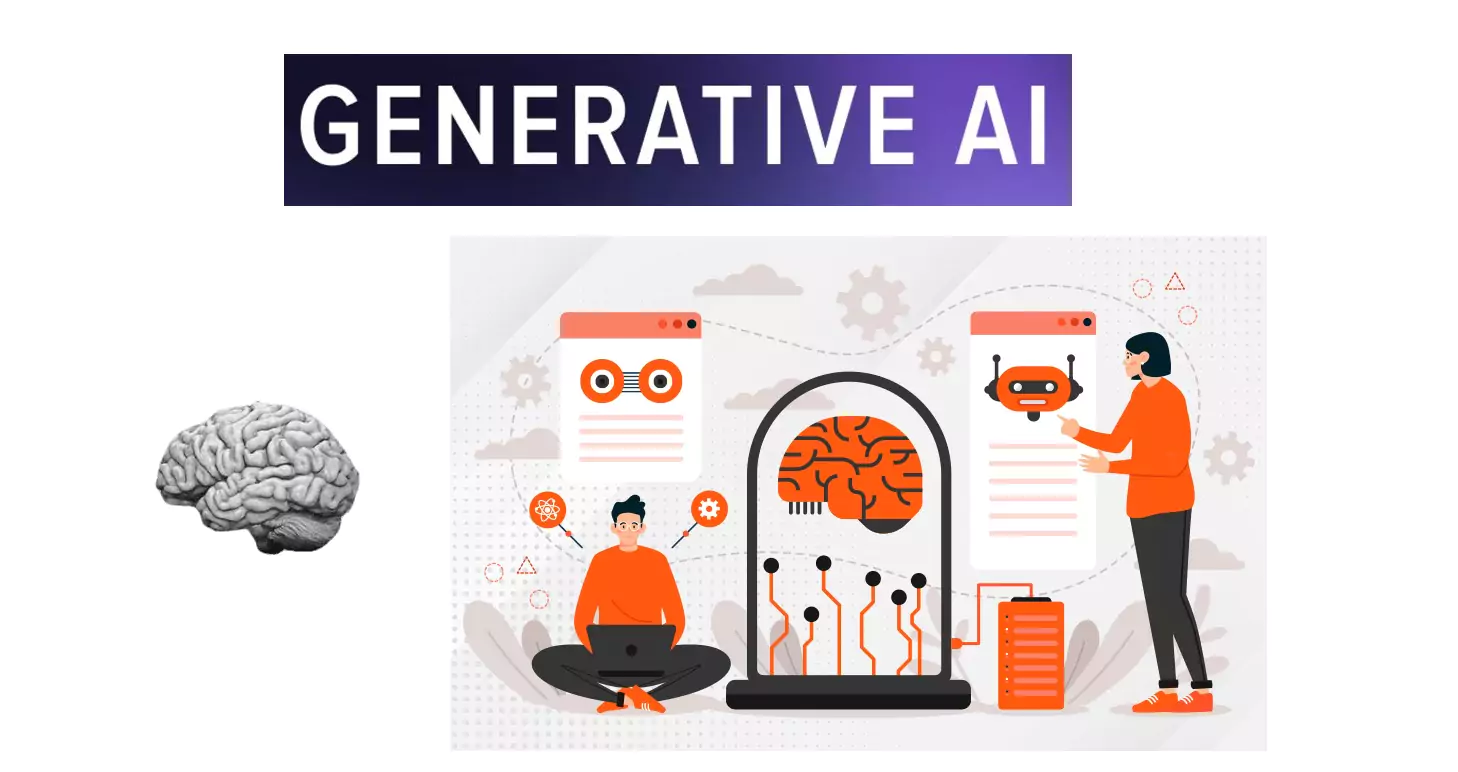
Early Approaches to Text-to-Speech Conversion
Concatenation, which uses a pre-recorded collection of speech sounds to form new words and phrases, was the first method of using TTS technology.
Splicing speech units together was how this method operated, but the drawback was that it frequently produced speech that sounded artificial.
Formant synthesis, which included producing sounds based on an established
frequency range, was later employed thoroughly.
Limitations of Traditional Methods
These early speech synthesis methods worked, but they had some drawbacks that prevented them from being used often.
The main drawback was that the computer-created voice sometimes sounded mechanical and robotic, making it difficult to understand.
Introduction to Generative AI in Text-to-Speech
Deep learning and machine learning techniques are used in generative AI to produce algorithms that can learn and imitate human behavior.
Through computer simulation, generative AI has made it possible to create realistic and lifelike sounds in text-to-speech synthesis.
High-quality voice synthesis that is limited by speech units or pre-recorded speech databases can be produced by using generative AI.
Understanding Generative AI
Let's learn more about Generative Adversarial Networks (GANs) and the importance of deep learning in improving Text-to-Speech (TTS) performance.
Generative Adversarial Networks (GANs)
Generative Adversarial Networks (GANs) are a subset of generative AI modeling that have shown great success in speech production.
Understanding GANs is essential to understanding generative AI in TTS.
The two networks that make up a GAN are the generator, which produces the output, and the discriminator, which evaluates the output's quality and gives the generator feedback so it can get better
Role of Deep Learning in Generative AI
A key aspect of generative AI in TTS is deep learning. Speech signals include hidden patterns and meanings that can be extracted and represented using deep neural networks.
Speech synthesis has improved considerably due to the use of deep learning in TTS, enabling voices to sound more realistic.
Training Generative AI Models for Text-to-Speech Generation
Researchers often use a dataset of recorded voices combined with matching text transcriptions to apply generative AI models for text-to-speech.
Through training, the model learns to link text input with related voice output and uses this knowledge to produce speech from new texts.
Usually, these models can produce highly customized and realistic speech that adjusts to each speaker's unique speech patterns.
How Generative AI Works in Text-to-Speech Generation?
There has been interesting progress in the quality of text-to-speech (TTS) technology, with generative artificial intelligence.
The discussion given below will give a general overview of the generative AI process in TTS.
Overview of the Process
There are usually a few steps involved in the TTS generative AI process. First, textual input is converted into phonetic transcriptions using a text-to-phoneme conversion method. After that, a phonetic transcription to acoustic feature translation is trained into an acoustic model. The generative AI model uses these Acoustic features to create waveforms, which are then transformed back into voice output.
Text-to-Phoneme Conversion
The TTS process starts with text-to-phoneme conversion. The idea behind this step is to turn textual input into phonetic transcriptions, which show how the text ought to be spoken. Several popular systems are available for converting text to sounds, such as Sequitur G2P and the CMU Pronouncing Dictionary.
Acoustic Model Training
An acoustic model is trained to convert phonetic transcriptions to acoustic characteristics after the transcriptions are received.
Acoustic modeling is the name of this procedure, which is important to the TTS process. TTS uses several audio model types, including deep neural networks (DNNs) and Hidden Markov Models (HMMs).
Much development has been made in DNN-based acoustic models recently, leading to improved quality synthetic speech.
Generative AI Model for Waveform Generation
The generative AI model uses the acoustic information produced by the acoustic model to create waveforms.
Deep Neural Networks (DNNs) and Generative Adversarial Networks (GANs) are two methods for generating waveforms. Deep neural networks use patterns they have learned during training to examine the auditory data and predict the waveforms.
In contrast, generative adversarial networks include two networks: a discriminator network that assesses the output waveform's quality and a generator network that creates the output waveform.
Input and Output in Text-to-Speech Conversion Using Generative AI
Textual input is used as the input data for TTS using generative AI. After processing this input, the model produces an output waveform.
TTS output formats come in two forms: parameterized and raw.
The continuous sampled signal is the raw output; waveform envelope, duration, and pitch are examples of features in the parameterized output.
While parameterized output generates speech that sounds more realistic, raw output offers greater flexibility and can be used to easily modify the output waveform.
Case Studies and Examples of Successful Text-to-Speech Systems Powered by Generative AI
The use of generative AI in TTS has developed a lot in recent years. One great example of a generative AI-powered TTS system that works well is Google WaveNet.
With the use of deep neural networks, this technique can produce high-quality synthetic speech by directly generating waveforms from textual input.
Neural TTS from Amazon is another effective TTS system that produces high-quality voice output using generative models.
Advantages of Generative AI in Text-to-Speech Generation
In this section, you'll find the advantages of Generative AI in Text-to-Speech generation.
High-Quality Voice Synthesis
Synthetic speech created using TTS can sound almost identical to human speech thanks to generative AI technologies.
These days, TTS systems can generate high-quality synthetic speech appropriate for a wide variety of languages and accents because of deep learning algorithms.
Customizability and Control Over Voice Characteristics
Users can customize various elements of synthesized voice, such as pitch and speed, using generative AI models for text-to-speech.
With the use of this control, one can create distinctive synthetic voices that can be edited and personalized as needed without having to record them again.
Improved Naturalness and Expressiveness in Speech
Speech output produced by deep learning-based TTS models sounds more genuine because they can identify small contextual cues in human speech.
More expressive synthetic speech is produced by these models, which also mimic the pitch and emotion seen in human speech.
Multilingual and Diverse Voice Generation
Many languages and accents that are difficult to generate using conventional rule-based modeling methods can be supported by generative AI. It can create voices that are appropriate for speakers with several vocal features, including gender, age, and local accents.
Challenges and Limitations of Generative AI for Text-to-Speech
In this section, check out the challenges and limitations of Generative AI for text-to-speech.
Data Scarcity and Quality
Limited and poor quality of data is one of the issues generative AI in text-to-speech (TTS) faces. For generative AI models to train effectively, a lot of high-quality data is needed.
gathering this kind of information can be costly and time-consuming. The TTS system's performance and generalization abilities can be impacted by a lack of representative and expanded datasets.
Overfitting and generalization
One other drawback of generative AI in TTS is overfitting. When a model becomes too specific to the training set and struggles to generalize to new data, it is said to be overfitted.
This can result in artificial speech that sounds unnatural and lacks the necessary expressiveness and diversity. In developing TTS, it is important to strike a balance between capturing the subtleties of the training data and generalizing to unseen input.
Ethical concerns and potential misuse
The use of generative AI in TTS involves ethical questions about potential abuse and privacy. As AI models develop, they will eventually be able to precisely imitate human voices. This raises questions about how synthetic voices can be used offensively to spread false information or imitate real people. For generative AI to be developed and used in TTS, it is necessary to ensure ethical usage and overcome these issues.
Future Directions and Applications of Generative AI in Text-to-Speech
It's that time of an article to know about the future directions and applications of Generative AI in text-to-speech. So check out the following:
Advancements and Ongoing Research in Generative AI for Text-to-Speech
The field of generative AI for TTS research and development is constantly changing. Current research efforts focus on improving the underlying algorithms and models to increase the synthetic speech's naturalness and quality. Prosody prediction, multi-speaker modeling, and transfer learning are a few strategies that try to push the limits of TTS technology and go over its current limitations.
Potential applications include virtual assistants, audiobook production, and accessibility services
There is a ton of potential for generative AI in TTS applications. One well-known use is in virtual assistants, where human interaction requires voices that seem natural.
Virtual assistants can offer a more engaging and human-like experience using generative AI, which will increase user happiness.
The production of audiobooks is another use. The need for human voice actors in audiobook creation can be decreased thanks to the use of generative AI.
This scalability makes audiobooks more accessible to readers all across the world and increases their variety.
TTS generative AI has the power to transform accessibility services. Synthetic voices that resemble human speech can help people with speech problems communicate more fluently and efficiently.
With the use of technology, communication challenges can be removed and participation for people with special needs can be improved.
Conclusion
In conclusion, the use of Generative AI for text-to-speech generation offers numerous benefits and opportunities across various industries and applications.
By harnessing the power of advanced machine learning algorithms, Generative AI can produce high-quality and natural-sounding speech that closely mimics human speech patterns.
One of the key advantages of using Generative AI for text-to-speech generation is its ability to adapt and learn from large datasets.
This allows for continuous improvement in speech synthesis quality. Additionally, Generative AI chatbots powered by text-to-speech capabilities can enhance user experiences by providing more engaging and personalized interactions.
The features of Generative AI, such as its ability to generate speech in multiple languages and accents, make it a versatile tool for a wide range of applications.
Furthermore, its efficiency and scalability make it suitable for both small-scale projects and large-scale deployments.
After overcoming challenges like limited data, generative AI in TTS succeeds and unlocks an endless number of applications.
It provides you with generative AI-powered audiobooks that reduce the need for voice actors, increasing access to great reading for all.
Overall, Generative AI holds tremendous potential for transforming the way we interact with technology and consume information.
As the technology continues to evolve and improve, we can expect to see even more innovative applications and use cases emerge in the future.
Embracing Generative AI for text-to-speech generation can lead to enhanced communication, improved accessibility, and greater efficiency in various domains.
Frequently Asked Questions (FAQs)
How is AI used in text to speech?
AI is used in text-to-speech through natural language processing and deep learning models.
These models process text input, interpret linguistic nuances, and generate corresponding audio output, creating a lifelike speech synthesis experience.
Can generative AI create audio?
Yes, generative AI can create audio by employing advanced algorithms that generate human-like speech patterns based on the input text, enabling the creation of natural and expressive audio output that closely resembles human speech.
Can AI generate speech from text?
Certainly, AI can generate speech from text by leveraging generative models, which interpret textual data, understand context, and generate corresponding audio output. These models employ sophisticated techniques to mimic human speech patterns, intonations, and emotions from the provided text.
Is speech recognition generative AI?
Speech recognition, while related to AI, is not categorized as generative AI. It involves identifying and interpreting spoken language, converting it into text.
Generative AI, on the other hand, focuses on creating new content, such as generating natural-sounding speech from text inputs, without relying on existing data alone.