PwC estimated a 7% price increase in healthcare by 2024. Why? Healthcare workers are facing burnout, resulting in a labor shortage, disagreements between payers and providers, and inflation.
The sector is investigating breakthrough technologies, such as generative AI in healthcare, to ensure effective patient care without incurring unnecessary operating expenditures.
According to Accenture, AI can improve 40% of healthcare practitioners' working hours, and a recent Forbes piece claims that this technology can save the US medical sector at least $200 billion each year.
In healthcare, generative AI uses machine learning algorithms to analyze unstructured data, such as patient health records, medical photographs, audio recordings of consultations, and so on, and generate new content similar to what it has been trained on.
This guide examines the possible applications and benefits of generative artificial intelligence in healthcare and the problems of implementation and ethical implications.
Understanding Generative AI
Generative Artificial Intelligence, in simple terms, refers to the ability of AI algorithms to create new and original data that imitates human-like patterns.
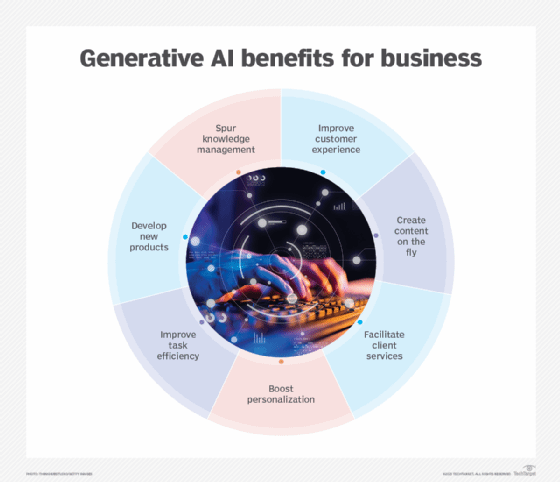
Unlike traditional AI, which relies on pre-existing data, generative AI can generate new information based on what it has learned from analyzing vast amounts of data.
Importance of Data in Healthcare
Data forms the cornerstone of healthcare decision-making. It drives scientific discoveries, treatment protocols, and personalized patient care.
However, healthcare data is often limited, incomplete, and fragmented.
This is where generative AI provides a magical solution by generating new data that fills the gaps.
Benefits of Generative AI in Healthcare
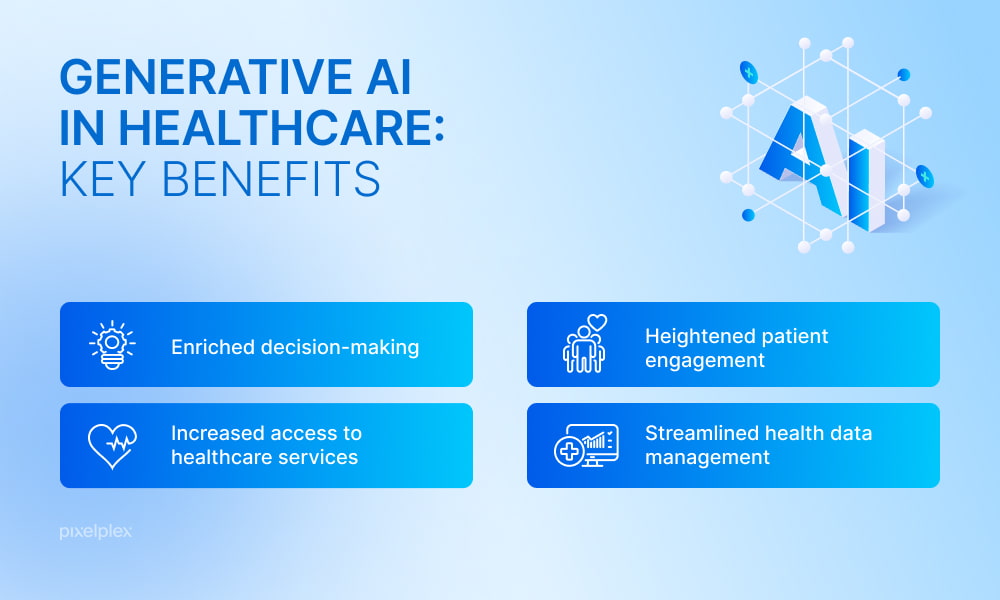
This section will explore the benefits of generative AI in healthcare and discuss its implementation challenges and ethical considerations.
Enhancing Diagnostic Accuracy and Precision
One of the most significant advantages of generative AI in healthcare is its ability to enhance diagnostic accuracy and precision.
By training algorithms on massive amounts of medical data, generative AI models can learn to identify intricate patterns and anomalies, aiding healthcare professionals in making more accurate diagnoses.
These models can interpret medical images, such as X-rays, MRIs, and CT scans, with a level of detail and accuracy that surpasses human capabilities.
This speeds up the diagnostic process and reduces the possibility of misdiagnosis, ultimately leading to better patient outcomes.
Enabling Personalized Treatment Plans
Generative AI also has the potential to revolutionize the way personalized treatment plans are created.
By analyzing a patient's medical history, genetic information, and other relevant data, generative AI algorithms can generate personalized treatment recommendations.
These recommendations consider individual factors such as genetic predispositions and medication sensitivities, providing a more tailored and effective approach to treatment.
By utilizing generative AI, healthcare professionals can optimize treatment plans, potentially minimizing adverse effects and maximizing positive outcomes for patients.
Improving Patient Monitoring and Disease Prediction
Generative AI can be crucial in improving patient monitoring and disease prediction.
By analyzing large volumes of patient data, including electronic health records, vital signs, and lifestyle information, generative AI models can identify subtle patterns that may indicate the onset or progression of a disease.
This early detection and prediction can facilitate timely interventions, potentially preventing adverse health events and improving patient outcomes.
Furthermore, generative AI algorithms can continuously monitor patients, providing real-time feedback and alerts to healthcare professionals, ensuring a proactive approach to patient care.
Facilitating Drug Discovery and Development
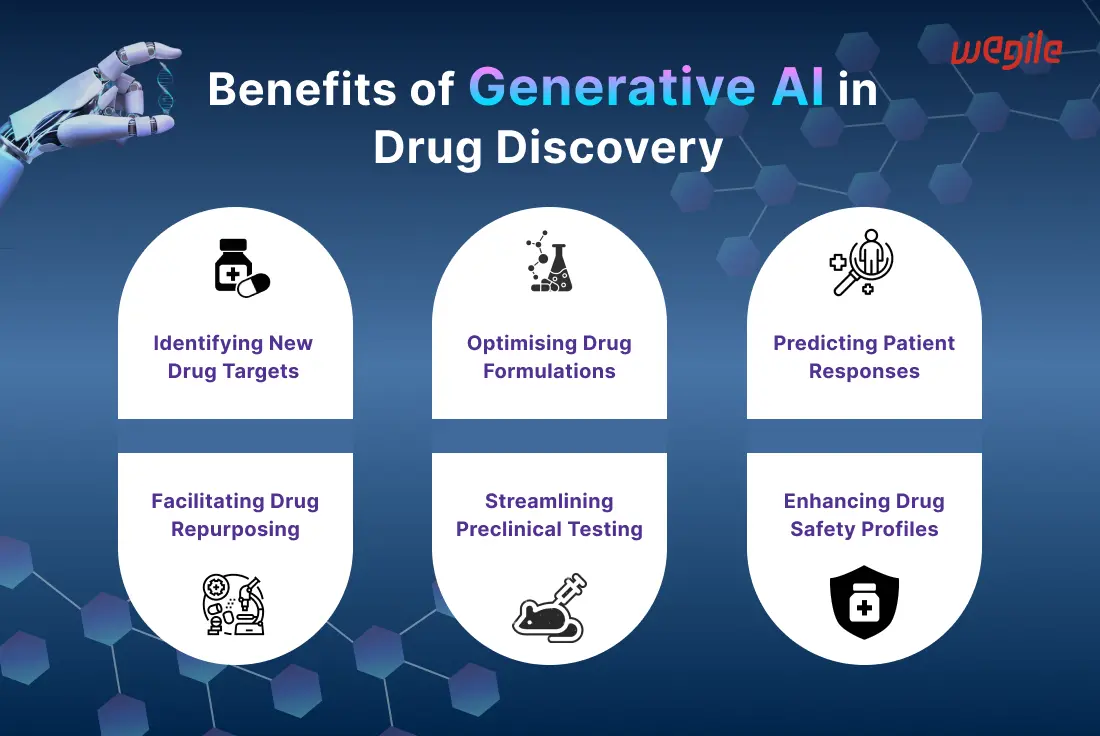
The drug discovery and development field is another area generative AI can profoundly impact.
Developing new drugs is an intricate and time-consuming process that involves synthesizing and testing numerous chemical compounds.
By leveraging generative AI, researchers can streamline this process by generating virtual compounds with desirable properties optimizing the selection of compounds for synthesis and testing.
This significantly reduces the time and resources required for drug discovery, potentially accelerating the development of new treatments and expanding the range of available therapies.
Challenges & Ethical Considerations

While generative AI holds immense potential, its implementation in healthcare also presents various challenges and ethical considerations.
Data Privacy and Security Concerns
Utilizing generative AI in healthcare requires access to vast patient data. This raises significant privacy and security concerns.
Safeguarding patient information is paramount to maintaining patient trust and ensuring privacy regulations compliance.
Healthcare organizations must invest in robust data security measures, such as encryption and strict access controls, to protect sensitive patient data from unauthorized access or breaches.
Bias and Fairness Issues in Data Generation
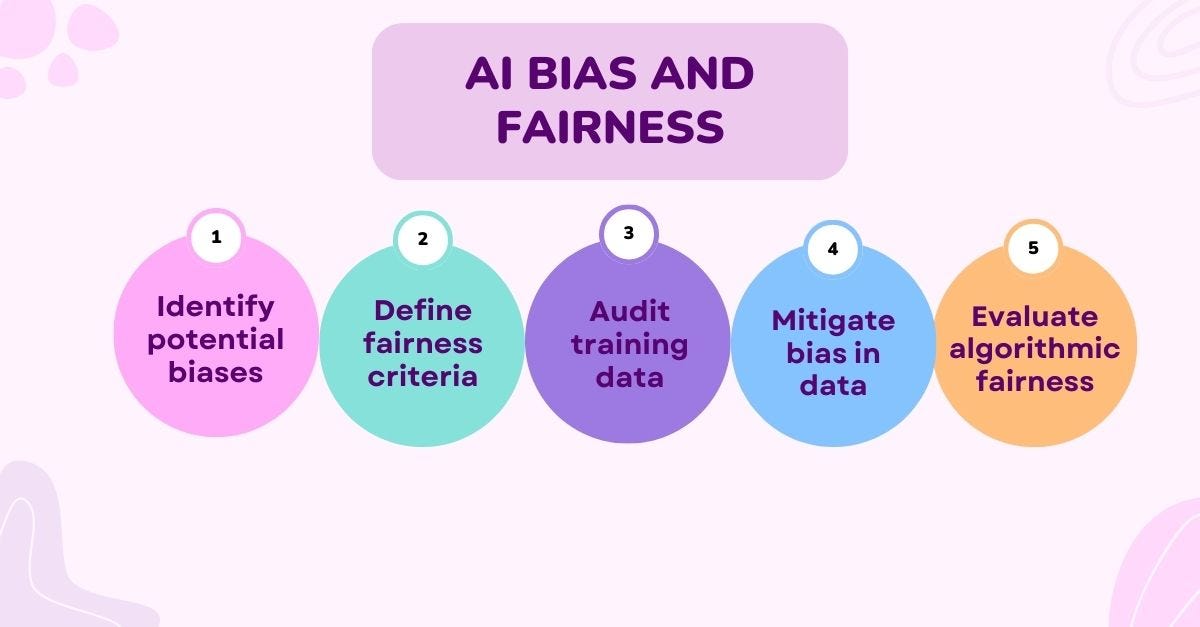
Another challenge when using generative AI in healthcare is the potential for bias and fairness issues in data generation.
If the training data used to develop generative AI models is biased, the generated content will reflect those biases.
This can result in inaccurate or unfair diagnostic assessments or treatment recommendations, impacting certain patient populations disproportionately.
To mitigate this, it is crucial to ensure diverse and representative training data and implement rigorous testing and validation processes to identify and rectify any biases in the models.
Suggested Reading:
Generative AI in Healthcare vs Traditional Methods
Ensuring Transparency and Interpretability of AI Algorithms
Transparency and interpretability of AI algorithms are essential in the healthcare domain.
Healthcare professionals and patients need to understand the reasoning and decision-making processes behind the generated content.
Black-box AI models, which lack transparency, can undermine trust in the technology and make it challenging to identify and rectify any errors or biases.
Therefore, developing explainable AI models that can provide human-readable explanations for their outputs is crucial.
This promotes accountability, improves collaboration between AI systems and human healthcare professionals, and improves patient understanding and confidence in the generated content.
Uses of Generative AI in Healthcare
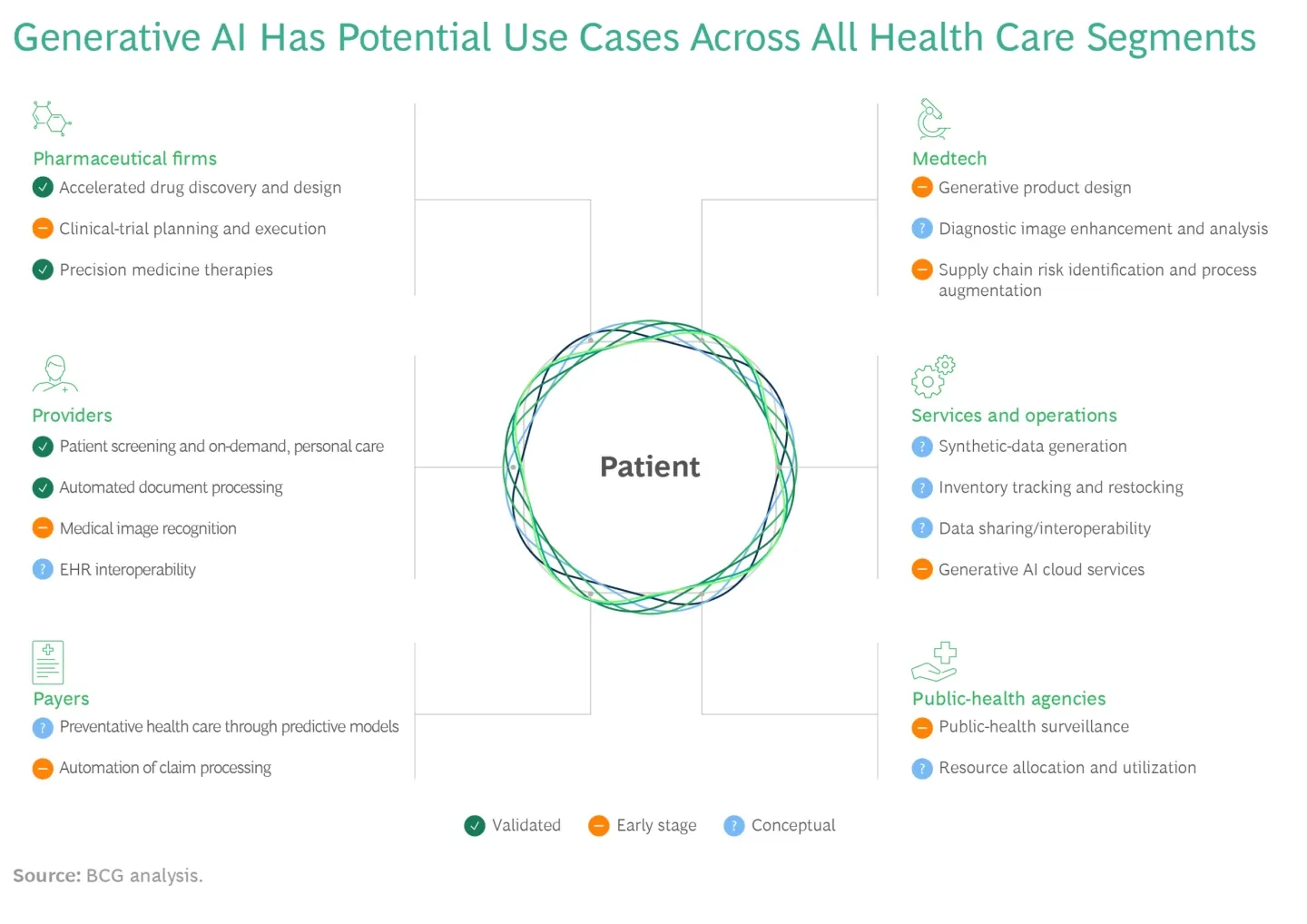
Generative AI, a subset of artificial intelligence, has found numerous applications in healthcare, offering innovative solutions to improve patient care, diagnosis, and research.
Here are some key Generative AI Use cases in the healthcare industry:
- Medical Image Generation: Generative Adversarial Networks (GANs) can create synthetic medical images, which can be used to train machine learning models and simulate rare medical conditions. This helps in education, diagnosis, and treatment planning.
- Drug Discovery: Generative models can assist in the discovery of new drugs and compounds by generating molecular structures that have potential therapeutic value. This can significantly speed up the drug development process.
- Personalized Treatment Plans: Generative AI can analyze a patient's medical history and genetic information to generate personalized treatment plans. These plans can consider the patient's unique needs, increasing treatment efficacy.
- Medical Data Augmentation: Generative models can generate synthetic patient data, including medical records and images. This can augment real data for research and training healthcare AI algorithms while maintaining patient privacy.
- Natural Language Processing (NLP): NLP models like GPT (Generative Pre-trained Transformer) can be used for medical record summarization, translation of medical documents, and even generating patient education materials.
- Disease Prediction: Generative AI can create predictive models that generate risk scores for various diseases based on patient data. These scores can help in early disease detection and prevention.
- Radiology Report Generation: AI-powered generative models can automatically generate radiology reports from medical images, saving time for radiologists and improving report consistency.
- Behavioral Health: Chatbots powered by generative AI can provide mental health support, generate responses to patients' emotional queries, and offer virtual counseling.
- Drug Formulation: Generative AI can assist in developing new drug formulations and combinations optimizing drug delivery methods for specific conditions.
- Medical Research: AI can generate hypotheses, simulate experiments, and analyze data, aiding researchers in exploring various aspects of healthcare and medical science.
- Patient Simulation: Generative models can create realistic patient simulations for medical training and education, allowing healthcare professionals to practice procedures and decision-making in a risk-free environment.
- Healthcare Chatbots: AI-driven chatbots can use generative AI to respond instantly to patient queries, schedule appointments, and even assist with post-treatment care instructions.
Chatbots play an important role in automating healthcare’s administrative tasks. And, appointment scheduling is just a fraction of the whole automation process that healthcare chatbots can provide.
Suggested Reading:
7 Innovative Ways Generative AI Apps Boost Business Productivity
Implementing Generative AI in Healthcare
Implementing generative AI in healthcare requires careful planning, compliance with regulatory requirements, and a focus on data privacy and security.
Here are the steps and considerations for effectively implementing generative AI in a healthcare setting:
Data Collection and Preprocessing
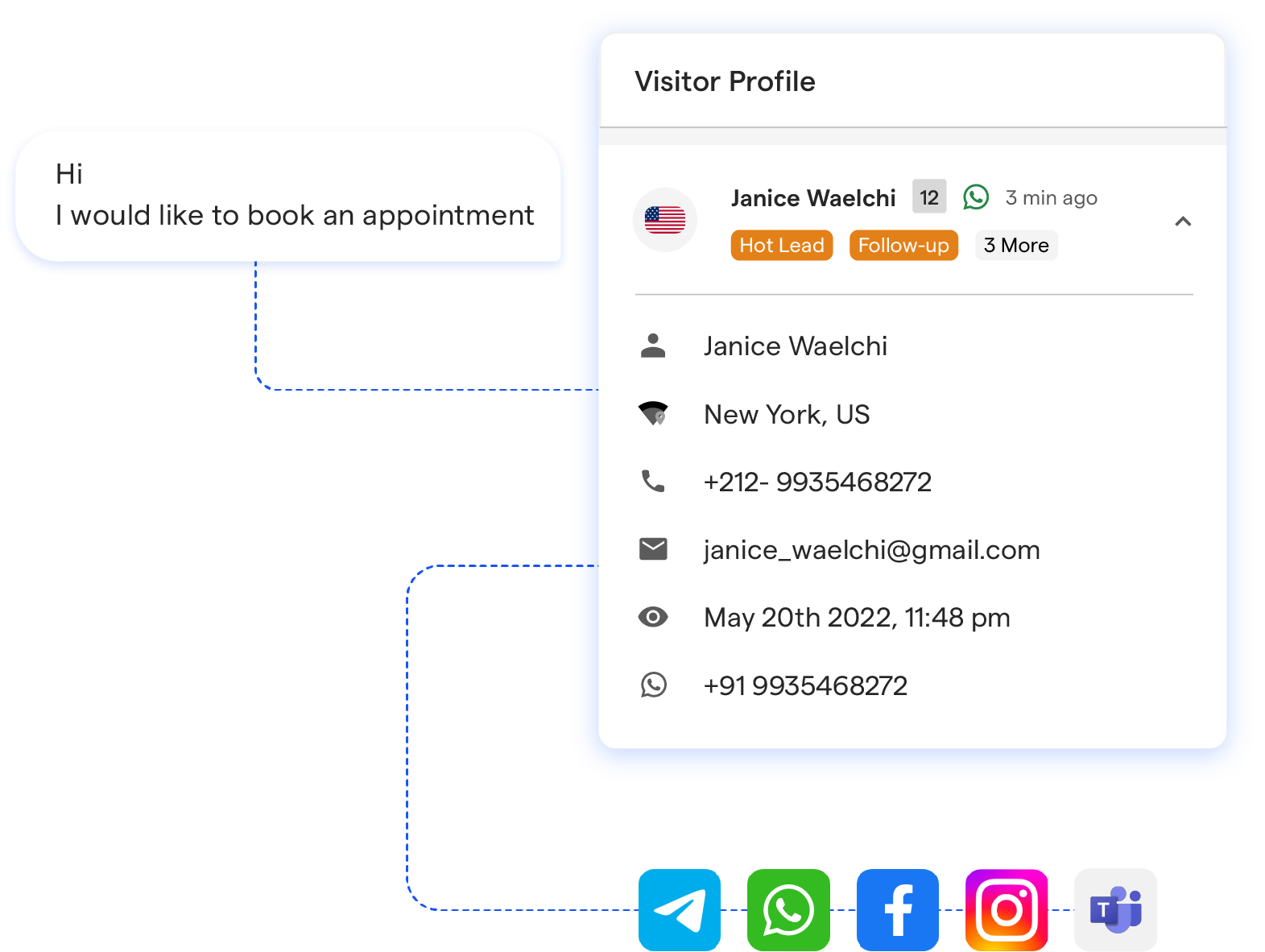
Collecting and organizing large volumes of high-quality data is essential for training generative AI models. This data includes medical images, patient records, genetic information, and other relevant healthcare data.
In addition, data preprocessing techniques must be employed to clean and normalize the data for effective analysis.
Choosing Appropriate Generative AI Algorithms
Choosing the right generative AI algorithms depends on the specific healthcare application.
Different algorithms, such as generative adversarial networks (GANs) or variational autoencoders (VAEs), offer distinct capabilities and suit different use cases.
Healthcare professionals and data scientists must select the most appropriate algorithm to achieve the desired outcomes.
Training and Fine-Tuning the Models
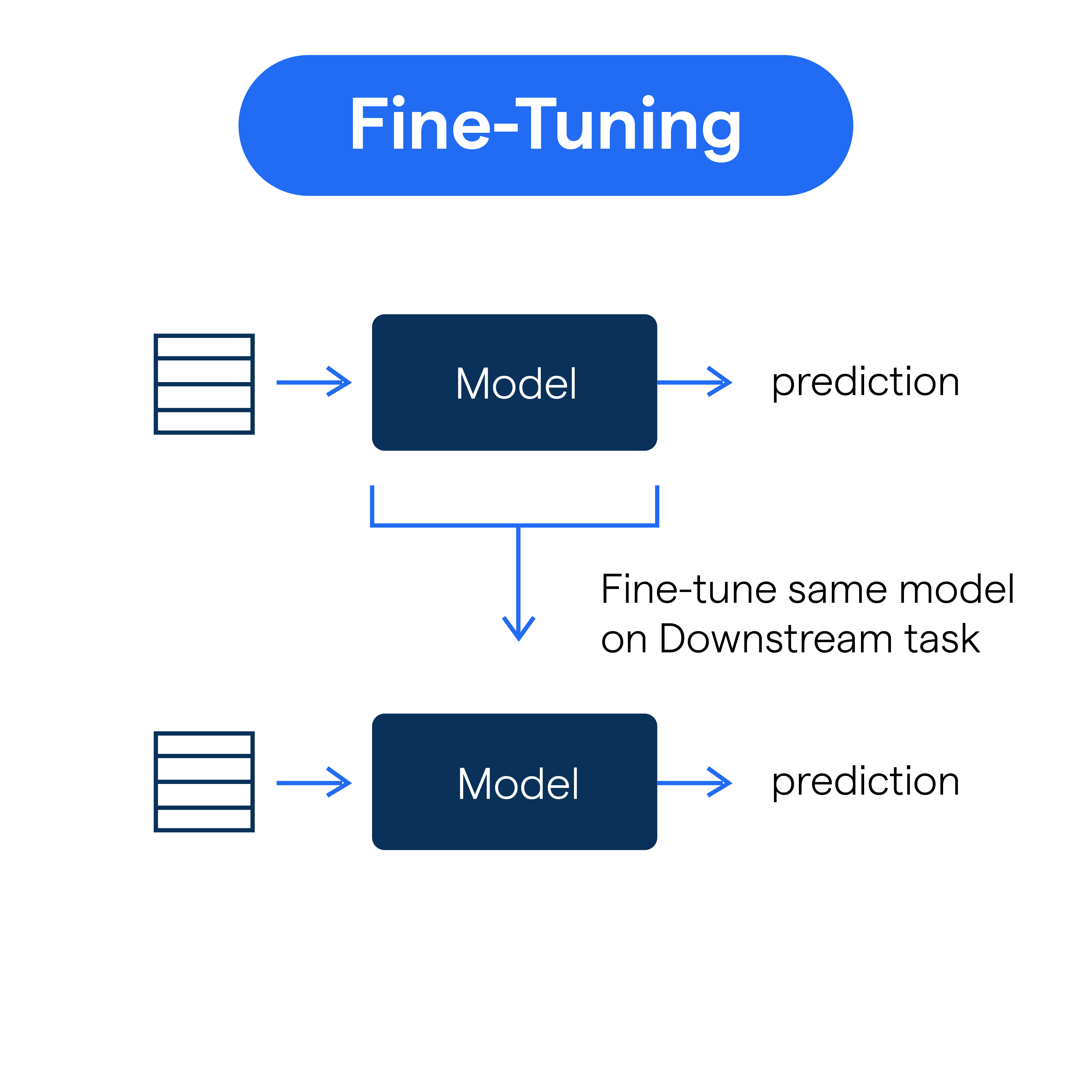
Generative AI models require extensive training on large datasets to learn patterns and generate accurate outputs.
The models' parameters are adjusted to improve performance during the training process.
Fine-tuning and optimization techniques ensure the models can deliver reliable and accurate results.
Validating and Evaluating the Performance of Generative AI Models
Validating generative AI models is crucial to ensure their reliability and efficacy in real-world healthcare settings.
By comparing the model's outputs with ground truth data and involving domain experts, healthcare professionals can evaluate the models' performance and identify any potential limitations or biases.
Conclusion
Healthcare using Generative AI can bring about transformative changes that greatly benefit patients and healthcare professionals.
We can increase disease diagnosis accuracy, speed drug discovery, deliver personalized treatment, and transform patient monitoring using generative models.
However, implementing generative AI requires careful consideration of data collection and preprocessing, choosing appropriate algorithms, training and fine-tuning models, and validating their performance.
By following these steps and embracing the potential of generative AI, we can unlock exciting possibilities for revolutionizing healthcare and achieving better patient outcomes.
So, let's embrace the power of generative AI and pave the way for a healthier future!
Frequently Asked Questions (FAQs)
How can generative AI be used for medical image analysis?
Generative AI assists in interpreting medical images, aiding in diagnosis, anomaly detection, and treatment planning, thus enabling more accurate and efficient analysis of complex medical data.
What are the benefits of using generative AI in drug discovery?
Generative AI expedites the process of drug development by generating molecular structures, predicting molecular properties, and optimizing compounds, facilitating faster and more effective drug discovery.
In what ways can generative AI assist in personalized treatment planning?
Generative AI aids in analyzing patient data to recommend personalized treatment plans, considering individual health factors, medical history, and potential treatment outcomes, thereby improving the precision and effectiveness of patient care.
How can generative AI be used in healthcare?
Generative AI finds application in medical image analysis, drug discovery, and patient data generation, facilitating accurate diagnosis, efficient research, and personalized treatment planning.
How does generative AI make headway in healthcare?
Generative AI advancements contribute to faster drug development, improved disease prediction, and customized patient care, revolutionizing healthcare practices and enhancing patient outcomes.