Introduction
Machine learning is failing us. That might sound surprising, but without proper engineering, most ML models don't reach their full potential. Many businesses struggle to implement ML effectively. This is where ML Model Engineering Services come in. They ensure that ML models are well-designed, robust, and scalable.
In the current data-driven world, businesses are increasingly turning to artificial intelligence (AI) to gain a competitive edge. But behind every smart AI solution lies the magic of machine learning (ML) models, which are the true workhorses driving innovation.
So, what exactly goes into creating these models, and how do businesses ensure they’re not just functional but also powerful and reliable? And the answer is ML Model Engineering Services—a fascinating blend of art, science, and engineering that turns raw data into actionable insights.
In this article, you'll find information on ML Model Engineering Services, explore how they’re transforming industries, and dive into the best practices for implementing them effectively.
What Are ML Model Engineering Services?
ML Model Engineering Services are specialized services focused on developing, deploying, and maintaining ML models. They ensure that ML Model Engineering Services are robust, scalable, and effective in real-world applications.
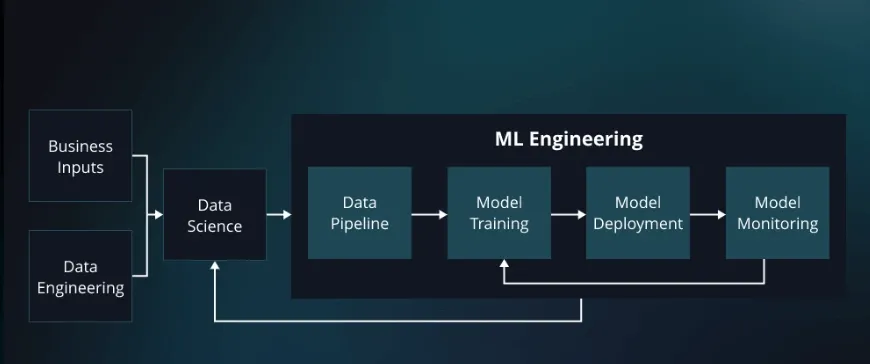
ML Model Engineering Services refer to a specialized set of processes and practices aimed at developing, deploying, and maintaining machine learning models in a production environment.
These services encompass the entire lifecycle of an ML Model Engineering Services, from the initial data collection and preprocessing stages to model selection, training, evaluation, and deployment.
The primary goal of ML Model Engineering Services is to ensure that machine learning models are not only accurate and efficient but also scalable, reliable, and aligned with business objectives. This involves selecting the right algorithms, optimizing performance models, and implementing them in a way that they can be integrated seamlessly into existing systems.
The Role of ML Model Engineering Services
ML Model Engineering Services are essential for transforming raw data into valuable insights. These services play a pivotal role in various aspects of business operations.
How These Services Support Businesses
ML Model Engineering Services supports businesses in their operations in many ways:
- Enhancing Decision-Making: ML Model Engineering Services & Development Company helps businesses make data-driven decisions. In retail, predictive models can forecast sales trends, allowing businesses to optimize inventory and improve customer satisfaction.
- Improving Efficiency: Automating routine tasks with ML model engineering services saves time and reduces errors. In logistics, ML model engineering services can optimize delivery routes, reducing fuel costs and improving delivery times.
- Driving Innovation: These services enable the development of innovative solutions. In healthcare, ML model engineering services can analyze medical images to detect diseases early, leading to better patient outcomes.
- Cost Reduction: By automating processes and improving efficiency, businesses can significantly reduce operational costs. In finance, fraud detection models can save millions by identifying fraudulent transactions quickly.
- Personalization: ML model engineering services allow businesses to personalize customer experiences. In e-commerce, recommendation engines suggest products based on customer preferences, increasing sales and customer loyalty.
Suggested Reading:The Role of ML Model Engineering in Building AI Solutions
Key Components of ML Model Engineering Services
To fully leverage the power of ML, it's important to understand the key components of ML Model Engineering Services. Each component plays a crucial role in the success of ML projects.
Data Collection and Preparation
Data is the foundation of any ML model engineering services. Proper data collection and preparation are essential.
- Data Collection: Gathering relevant data from various sources is the first step. In the automotive industry, data from sensors, cameras, and user interactions is collected to develop self-driving car models.
- Data Cleaning: Ensuring the data is clean and free of noise is crucial. In finance, cleaning transaction data helps in building accurate models for risk assessment.
- Data Preprocessing: This involves transforming raw data into a format suitable for model training. In healthcare, preprocessing patient records ensures the data is consistent and ready for analysis.
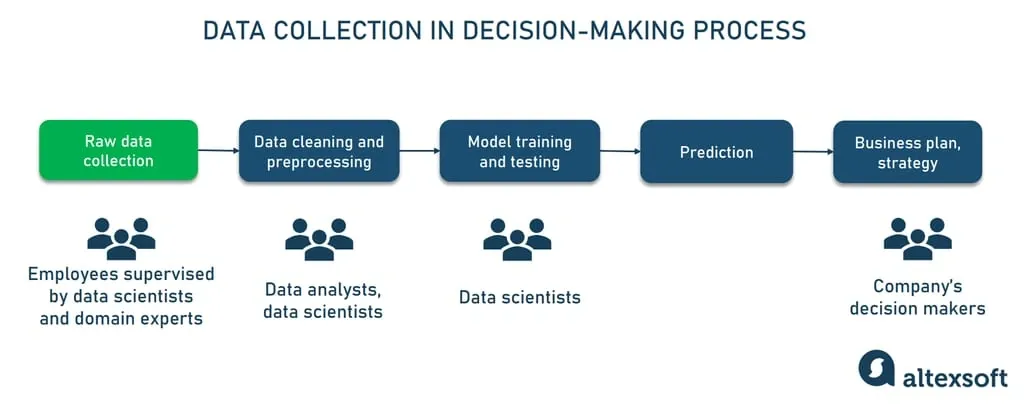
Model Selection and Training
Choosing the right model and training it properly is critical to the success of any ML project.
- Model Selection: Selecting the appropriate model depends on the specific problem. In marketing, a classification model might be chosen to predict customer churn.
- Model Training: Training the model with historical data to learn patterns. In weather forecasting, models are trained with historical weather data to predict future conditions.
- Hyperparameter Tuning: Adjusting model parameters to improve performance. In sports analytics, tuning parameters can enhance the accuracy of player performance predictions.
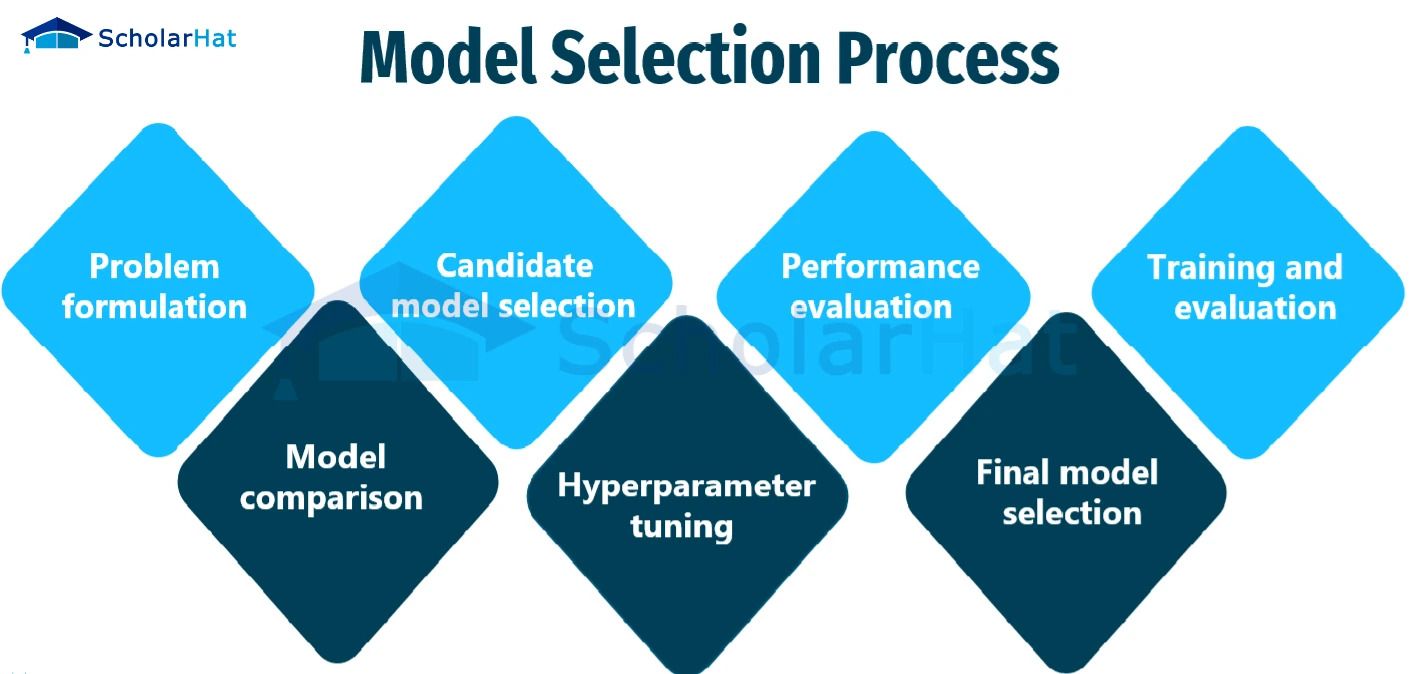
Model Evaluation and Validation
Evaluating and validating models ensures they perform well and are reliable.
- Model Evaluation: Assessing the model's performance using metrics such as accuracy and precision. In medical diagnostics, evaluating the model ensures it can accurately detect diseases.
- Cross-Validation: Using different subsets of data to validate the model. In financial forecasting, cross-validation helps ensure the model can generalize to new data.
- Performance Metrics: Common metrics include accuracy, recall, precision, and F1 score. In image recognition, these metrics help assess the model's ability to correctly identify objects.
Suggested Reading:7 Tips for Fine TuningYour Machine Learning Model
Model Deployment
Deploying models is the process of making them operational in real-world applications.
- Integration: Integrating the model into existing systems and workflows. In retail, integrating a recommendation engine into an e-commerce platform enhances the shopping experience.
- Scalability: Ensuring the model can handle large volumes of data and requests. In social media, deploying scalable models helps in analyzing vast amounts of user data for content recommendations.
- Real-Time Processing: Making models capable of processing data in real time. In cybersecurity, real-time models can detect and respond to threats instantly.
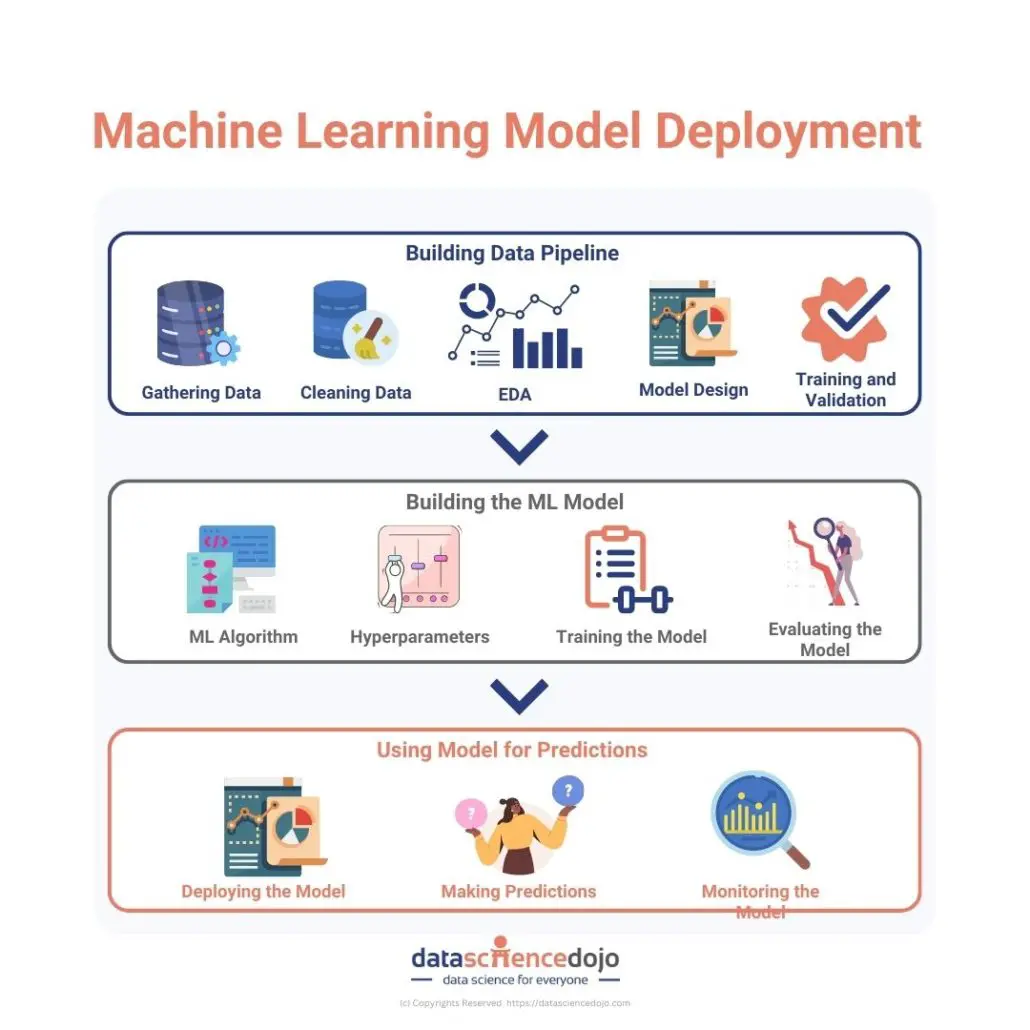
Monitoring and Maintenance
Continuous monitoring and maintenance are essential to keep models effective over time.
- Performance Monitoring: Regularly checking the model's performance to detect any degradation. In online advertising, monitoring ensures ad targeting models remain effective.
- Model Retraining: Updating the model with new data to maintain its accuracy. In supply chain management, retraining models with recent data ensures accurate demand forecasting.
- Error Handling: Addressing any errors or anomalies that arise during model operation. In healthcare, promptly addressing errors in diagnostic models is critical to patient safety.
ML Model Engineering Services are vital for any business looking to harness the power of machine learning. By understanding and implementing these components, businesses can develop robust, scalable, and effective ML models.
Partnering with an ML Model Development Company can provide the expertise needed to navigate the complexities of ML model development and deployment, driving efficiency and innovation.
Benefits of ML Model Engineering Services
ML model engineering services offer numerous benefits that can revolutionize business operations. By improving efficiency, reducing costs, and enhancing decision-making capabilities, these services enable companies to stay competitive and innovative.
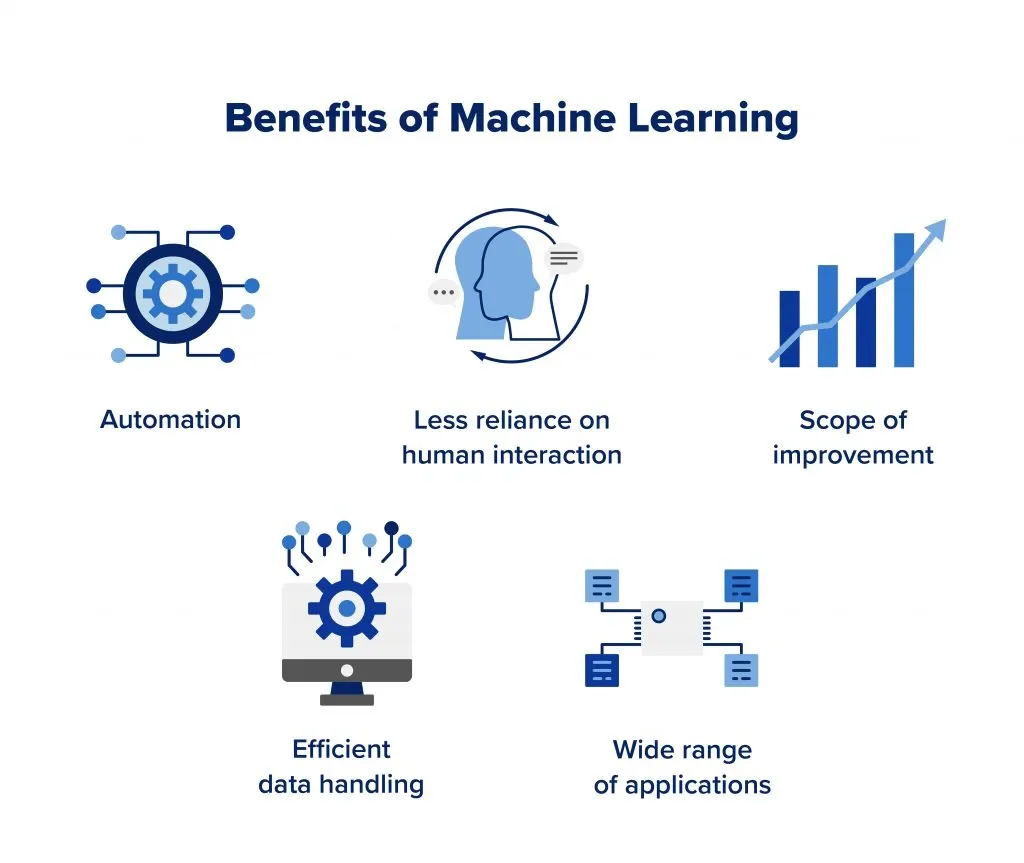
Improved Efficiency and Accuracy
Efficiency and accuracy are critical in today’s fast-paced business environment. ML model engineering services enhance these aspects through automation and precise data analysis.
- Automation of Routine Tasks: Automating repetitive tasks frees up valuable time for employees to focus on more complex activities.
In customer service, chatbots powered by ML can handle routine queries, allowing human agents to address more complex issues.
- Accurate Predictions and Analysis: Models trained with historical data can provide accurate predictions, helping businesses anticipate future trends. In supply chain management, ML model engineering services predict demand, ensuring inventory levels are optimized, and reducing overstock and stockouts.
- Streamlined Processes: By integrating ML models into business processes, companies can streamline operations. In finance, automated fraud detection systems analyze transactions in real-time, reducing the need for manual review and increasing detection accuracy.
Suggested Reading:The Benefits of Machine Learning Development
Cost Savings and Scalability
ML Model Engineering Services help businesses save costs and scale their operations efficiently. These services ensure that ML models are robust and scalable, capable of handling large volumes of data and transactions.
- Reduced Operational Costs: Automation of tasks and optimization of processes lead to significant cost savings. In manufacturing, predictive maintenance models reduce downtime by forecasting equipment failures before they occur, minimizing repair costs and lost production time.
- Scalability of Solutions: Scalable ML models can grow with the business, handling increasing data volumes without compromising performance. E-commerce platforms use scalable recommendation systems to manage growing customer bases and product inventories, providing personalized recommendations to millions of users.
- Efficient Resource Allocation: ML model engineering services optimize resource allocation, ensuring that resources are used where they are most needed. In logistics, route optimization models determine the most efficient delivery routes, reducing fuel consumption and delivery times.
Enhanced Decision-Making Capabilities
Accurate and timely decision-making is vital for business success. ML model engineering services provide the tools and insights needed to make informed decisions quickly.
- Data-Driven Insights: ML models analyze vast amounts of data, providing insights that inform decision-making. Customer segmentation models analyze buying behaviours in marketing, helping businesses target their marketing efforts more effectively.
- Predictive Analytics: These models predict future trends, enabling proactive decision-making. In healthcare, predictive analytics models forecast patient admission rates, allowing hospitals to manage staffing and resources better.
- Real-Time Decision Support: ML models offer real-time analysis, supporting immediate decision-making. In finance, real-time trading models analyze market data, allowing traders to make quick, informed decisions to maximize returns.
ML model engineering services play a crucial role in enhancing business operations. By partnering with an ML Model development company, businesses can leverage these benefits to stay ahead of the competition.
These companies provide the expertise needed to develop and deploy robust ML model engineering services, ensuring that businesses can efficiently and accurately analyze data, save costs, and make informed decisions.
Challenges in ML Model Engineering
ML model engineering services face various challenges that can hinder their success. Addressing these challenges is essential for creating robust and reliable ML model engineering services
Data Quality Issues
Data quality is a critical factor in the success of ML model engineering services. Poor-quality data can lead to inaccurate predictions and unreliable models.
- Inconsistent Data: Inconsistent data can create significant problems. In healthcare, inconsistent patient records can lead to incorrect diagnoses and treatment plans, jeopardizing patient safety.
- Missing Data: Missing data can skew model results. In financial services, missing transaction data can lead to inaccurate fraud detection, allowing fraudulent activities to go unnoticed.
- Noisy Data: Noise in data can obscure meaningful patterns. In manufacturing, sensor data with a lot of noise can lead to faulty predictive maintenance models, causing unexpected equipment failures.
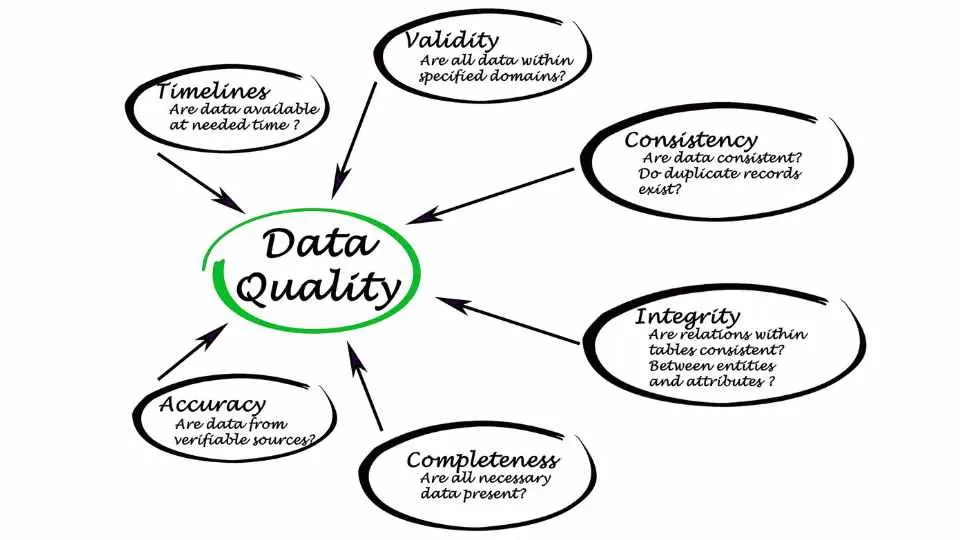
Model Interpretability
Understanding how ML models make decisions is crucial for trust and accountability. However, many models operate as "black boxes," making their decisions difficult to interpret.
- Complex Algorithms: Advanced models like deep learning are often complex and opaque. In legal contexts, using such models for case outcome predictions can be problematic if the rationale behind decisions cannot be explained.
- Lack of Transparency: Without transparency, it is hard to trust model outcomes. In hiring processes, using opaque models for candidate selection can lead to biases and discrimination, causing legal and ethical issues.
- Regulatory Compliance: Some industries require explanations for decisions made by automated systems. In finance, regulations demand that credit scoring models be interpretable to ensure fair lending practices.
Keeping Up with Rapid Technological Changes
The field of ML model engineering services is evolving rapidly, and keeping up with these changes is challenging for many organizations.
- Constant Innovation: New algorithms and techniques are constantly emerging. In cybersecurity, new threat detection algorithms can quickly become outdated, leaving systems vulnerable to new types of attacks.
- Skill Gaps: Rapid technological advances require continuous learning. In many industries, finding and retaining skilled ML model engineering services engineers who are up-to-date with the latest advancements can be difficult.
- Integration with Existing Systems: Integrating new ML technologies with legacy systems can be complex. In logistics, implementing new route optimization algorithms might require significant changes to existing software and hardware infrastructure.
ML model engineering services must navigate these challenges to deliver effective solutions. Partnering with an ML Model development company can provide the expertise needed to address data quality issues, improve model interpretability, and keep up with technological changes.
By focusing on data quality, businesses can ensure their ML model engineering services are built on a solid foundation. Partnering with ML Model Engineering Services & Development Company ensures access to high-quality data and robust preprocessing techniques.
Best Practices for Effective Implementation of ML Model Engineering Services
Implementing ML model engineering services effectively requires a combination of strategic planning, robust methodologies, and continuous monitoring. Here are some best practices to ensure success:
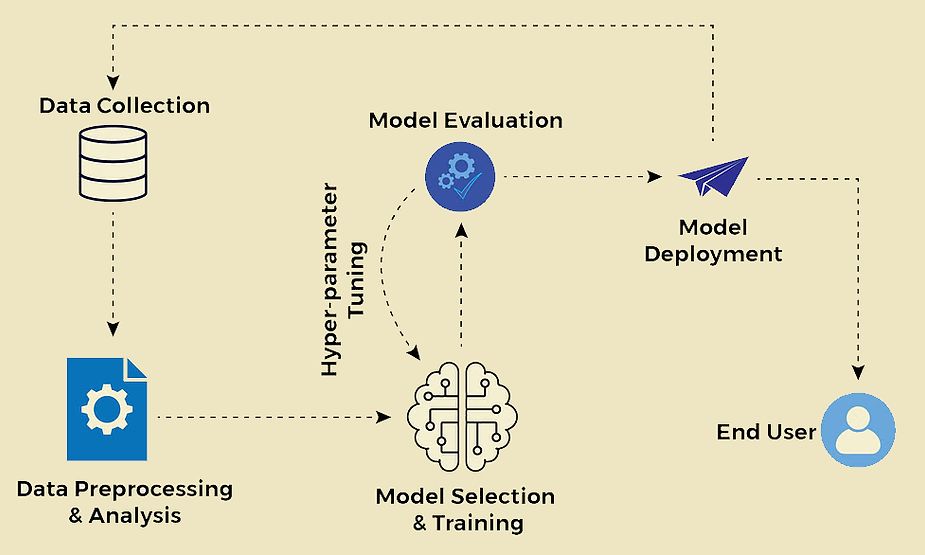
Start with Clear Objectives
Before diving into model development, it's crucial to have a clear understanding of the problem you're trying to solve and the business goals you want to achieve.
Defining key performance indicators (KPIs) and success metrics at the outset helps guide the model engineering process and ensures alignment with organizational objectives.
Focus on Data Quality
High-quality data is the foundation of any successful ML model engineering services. Invest time in thorough data collection, cleaning, and preprocessing to remove inconsistencies, outliers, and noise.
Proper feature engineering and selection can significantly enhance model performance, making it essential to experiment with different techniques to extract the most relevant features from your data.
Choose the Right Model
The choice of model should be guided by the specific requirements of your task, the nature of your data, and the computational resources available.
It's essential to experiment with multiple algorithms, tuning hyperparameters to find the best fit. Additionally, using ensemble methods or hybrid models can often yield better results by combining the strengths of different approaches.
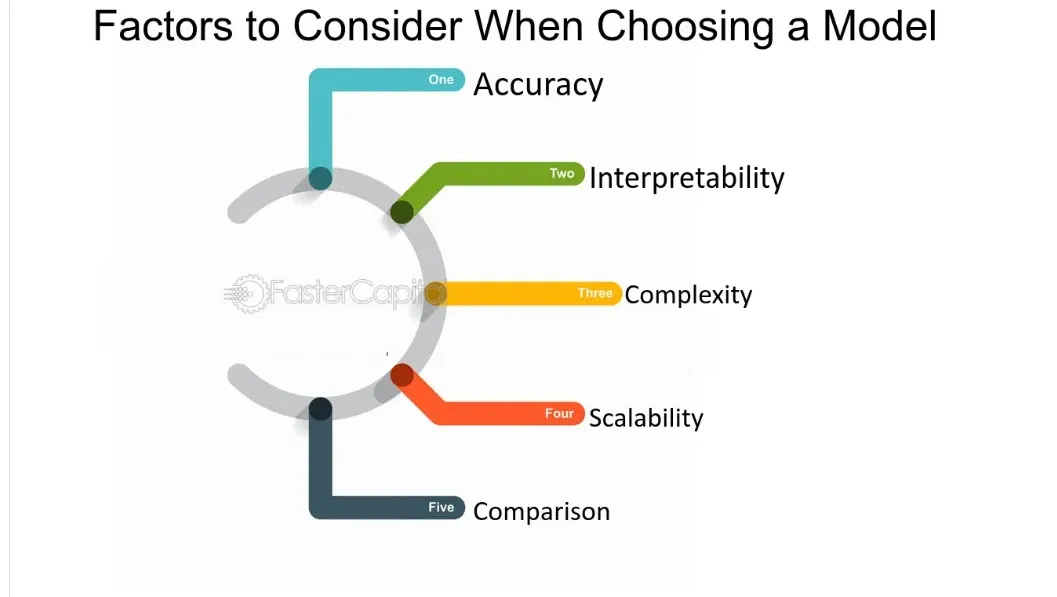
Prioritize Model Interpretability
In many business contexts, it’s important not only to have an accurate model but also to understand and explain its decisions.
Choose models that strike a balance between performance and interpretability, especially when stakeholders need to understand the model's predictions for compliance or decision-making purposes.
Implement Rigorous Testing and Validation
Cross-validation, A/B testing, and other validation techniques are critical to ensuring your model performs well not just on historical data but in real-world scenarios. Continuously monitor model performance post-deployment to detect any drift or degradation over time.
Plan for Scalability and Maintenance
Design your ML model engineering services pipelines with scalability in mind, ensuring that they can handle increasing data volumes and more complex models as your business grows. Additionally, establish protocols for regular model updates and retraining to adapt to changing data patterns and maintain accuracy.
Ensure Ethical and Compliant Practices
Finally, it’s vital to ensure that your models adhere to ethical standards and comply with relevant regulations. This includes being mindful of biases in data, ensuring transparency in model decisions, and safeguarding user privacy.
Conclusion
As businesses ride the wave of the digital age, ML model engineering services become important which helps them stay ahead of the curve. These services aren’t just about crunching numbers—ML model engineering services are about turning data into gold, ensuring your machine learning models are not only smart but also adaptable and reliable.
ML model engineering services are essential in ensuring that ML models are robust, scalable, and effective. Partnering with an ML Model development company provides the expertise needed to navigate these complexities, ensuring models are well-designed and adaptable.
As businesses continue to embrace machine learning, ML model engineering services will be increasingly crucial, driving innovation, efficiency, and sustainable growth. In today’s data-driven world, leveraging these services is not just an advantage but a strategic necessity, positioning businesses for future success.
By following the best practices we’ve explored, you can unlock the full potential of ML model engineering services, making sure your models hit the mark every time. From keeping your data squeaky clean to choosing the perfect algorithm, and from testing like a pro to staying on the right side of ethics, effective ML model engineering services are your ticket to smarter decisions and innovation on autopilot.
So, as you gear up to tackle the future, remember: it’s not just about building any model—it’s about crafting the right model that’s going to keep your business thriving for the long haul.
Frequently Asked Questions (FAQs)
Why are ML Model Engineering Services important?
These services ensure that machine learning models are robust, accurate, and efficient, enabling businesses to make data-driven decisions, automate processes, and enhance operational efficiency.
What challenges do ML Model Engineering Services address?
They address data quality issues, model interpretability, and the need to keep up with rapid technological changes, ensuring models remain accurate and reliable over time.
How do ML Model Engineering Services improve business efficiency?
By automating routine tasks, optimizing processes, and providing accurate data analysis, these services help businesses save time, reduce errors, and enhance overall efficiency.
What role does data quality play in ML Model Engineering Services?
High-quality data is crucial for building accurate and reliable models. Ensuring data is clean, consistent, and complete is a fundamental step in the ML model engineering process.
How can businesses keep up with rapid technological changes in ML?
Partnering with an ML Model development company provides access to the latest advancements, ensuring that models are built using state-of-the-art techniques and remain competitive.