AI has come a long way, and ChatGPT from OpenAI is a game-changer. This chatbot can write poems, jokes, and essays that seem human-made.
It's like magic—give it a few words, and it creates love poems in Yelp reviews or song lyrics like Nick Cave.
Before, generative AI made selfies look like Renaissance art and aged faces on social media. Now, it's all about language.
These big language models can talk about anything, from code to molecules to images. They're not limited to language alone.
This shift in AI from visuals to language is captivating people's minds. These models can learn different data types, making leaps in natural language processing and sparking imagination like never before.
According to a recent report by McKinsey & Company, the global market for generative AI is expected to reach $1.5 trillion by 2030.
As this field grows, let's go back to basics: what's generative AI, how did we get here, and how do these models work?
Applications of Generative AI
Generative AI has found applications in various industries, revolutionizing how tasks are performed and content is created. Here's an overview of how Generative AI is used in different fields:
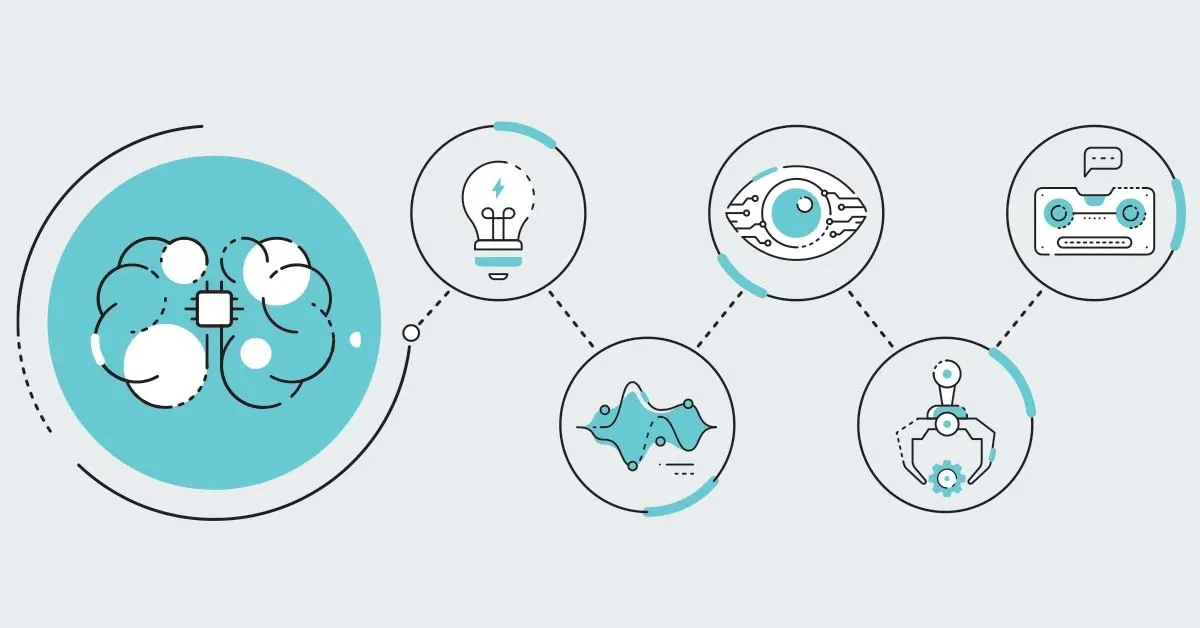
Art and Design
Generative AI has created new and unique visual artworks, such as paintings, sculptures, and digital graphics.
Artists and designers can input specific parameters or styles into the generative model, allowing it to generate original content following those criteria.
It can also assist in the design process by generating different variations and options for a given design or layout, enabling designers to explore new possibilities and accelerate the creative process.
Music
Generative AI has been used to compose melodies, harmonies, and even full-length music pieces.
Generative models can generate new musical compositions that resemble specific genres or artists by analyzing patterns and styles from existing music data.
It can also aid in music production by generating accompaniment tracks, suggesting chord progressions, and even generating song lyrics.
Video Games
Generative AI techniques are widely utilized in the gaming industry.
From procedural content generation for in-game levels, landscapes, and characters to creating realistic and intelligent non-player characters (NPCs), generative models have significantly enhanced video games' content and interactive aspects.
Generative models can also generate realistic dialogue and narratives for game storylines, creating immersive and engaging experiences for players.
Medicine and Healthcare
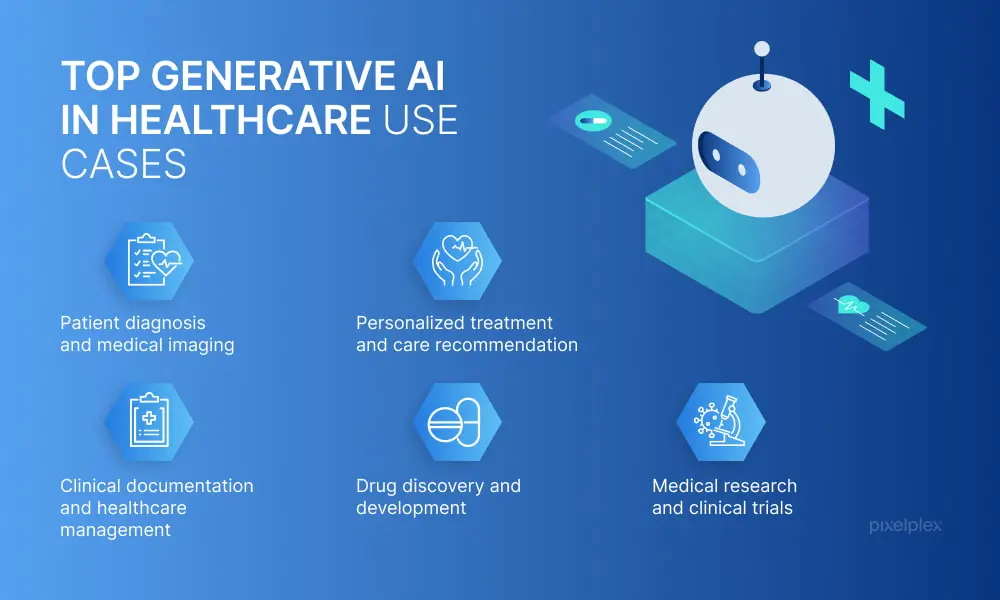
In medicine, Generative AI can assist in drug discovery by generating and screening potential new molecules with desired properties.
By simulating known compounds' chemical structures and properties, generative models can propose new drug candidates for further testing.
It can also be used for medical imaging applications, such as generating high-quality medical images, denoising scans, and even simulating the effects of different treatments or conditions.
These are just a few examples of how Generative AI is employed in different industries.
The technology's ability to generate new, creative content with minimal human intervention opens up numerous possibilities for innovation and problem-solving in fields ranging from entertainment and art to healthcare and beyond.
Its impact continues to expand as researchers and practitioners explore new ways to leverage generative models in practical applications.
Suggested Reading:
Benefits of Generative AI
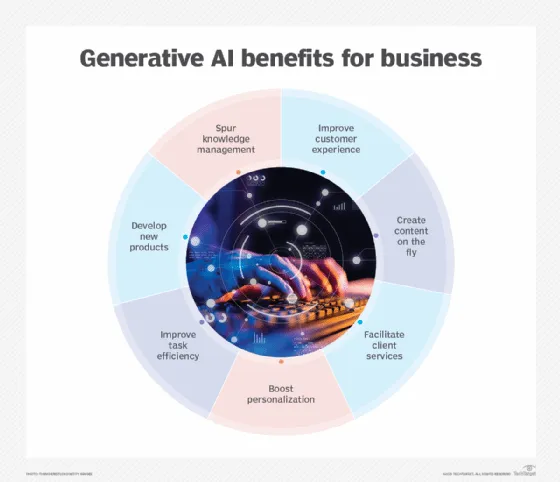
Generative AI offers several benefits by simplifying complex tasks and enhancing creativity and innovation:
Simplifying Complex Tasks
Generative AI can automate and simplify complex tasks that require significant human effort and expertise.
For example, generative models can generate multiple design options in the design field, speeding up the iterative design process and providing designers with a broader range of possibilities to explore.
In fields like drug discovery, generative models can assist in generating new molecules with desired properties, reducing the time and cost required for traditional trial-and-error methods.
Improving Creativity and Innovation
Generative AI can be a source of inspiration and a creative catalyst by generating new and diverse options.
It can help artists, designers, and musicians explore uncharted territories and break free from conventional patterns by generating novel and unique content.
By automating time-consuming and repetitive tasks, generative AI allows creative professionals to focus more on higher-level thinking and innovation, enabling them to push the boundaries of their respective fields.
Implications of Generative AI
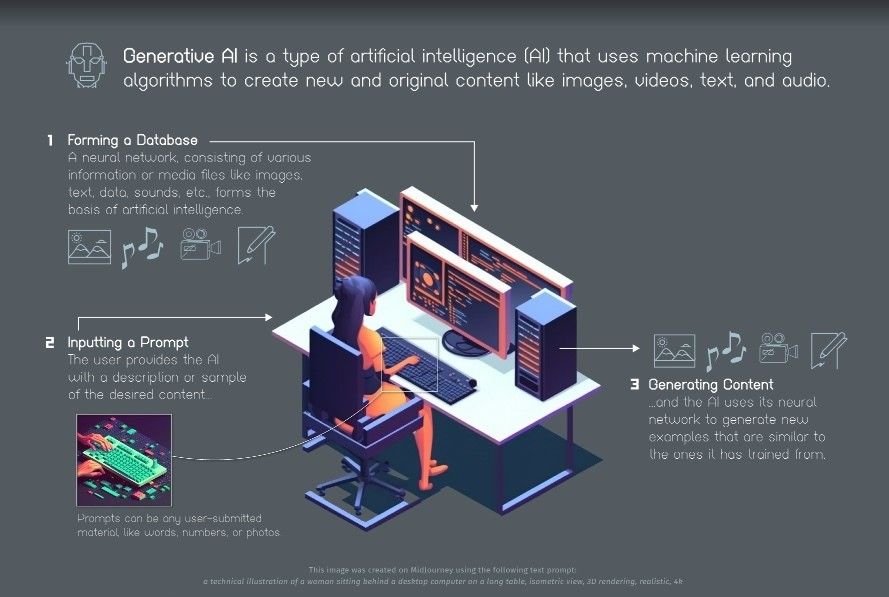
While Generative AI offers significant benefits, it also raises important ethical and societal considerations, along with implications for the workforce:
Ethical and Societal Considerations
Using generative AI in creating deepfake videos raises concerns about the potential for deception and misinformation.
Establishing ethical guidelines and regulations is crucial to prevent the malicious use of generative models.
Generative AI can also raise copyright and intellectual property issues, as it has the potential to create content that closely resembles existing works, leading to questions around ownership and originality.
Impact on Employment and the Workforce
The automation capabilities of generative AI have the potential to impact certain job roles and tasks.
Fields that heavily rely on manual and repetitive tasks, such as graphic design or music composition, may experience changes in job requirements and skill sets.
However, generative AI also creates new opportunities. It can complement human creativity, allowing professionals to explore new possibilities and focus on higher-level tasks that require critical thinking and decision-making.
It is crucial to carefully consider these implications and strike a balance between the benefits and potential risks associated with Generative AI.
We can leverage the technology's capabilities by addressing ethical considerations and preparing for potential workforce changes while minimizing negative impacts and ensuring its responsible use.
How Generative AI Learns and Improves?
Generative AI adopts a model of learning that’s similar to how a human artist improves over time.
It observes, learns, experiments, and refines its approach based on the results.
The foundation of its learning lies in data. The more data it sees, the more it learns.
Picture this: an AI wants to learn to create an image of a cat. It starts by studying thousands of cat images. It notes down common features like whiskers, pointy ears, and a tail. This process is like an artist studying a subject before picking up the brush.
But studying is just part of the process. What happens next is where the 'AI magic' happens. The AI uses its learning to generate a new image - its version of a cat.
Like an artist’s first sketch, the result might be pretty rough. So, the AI uses feedback to improve. How close is its cat to real cat images? The closer it is, the better it has done.
In this feedback stage, the AI is both the student and the teacher. It creates the image, judges how close it is to real ones, and figures out how to improve. It's a continuous, endless cycle of learning and refining that leads to some incredibly realistic results over time.
This learning framework isn't just for images. It applies to everything from writing text to composing music.
Generative AI is like a committed artist, constantly learning, experimenting, and perfecting its craft. The difference is this artist works at lightning speed, never sleeps, and only gets better over time.
By crafting ready-to-deploy AI chatbot solutions for multiple platforms, BotPenguin makes sure that your business reaches wherever your customers are:
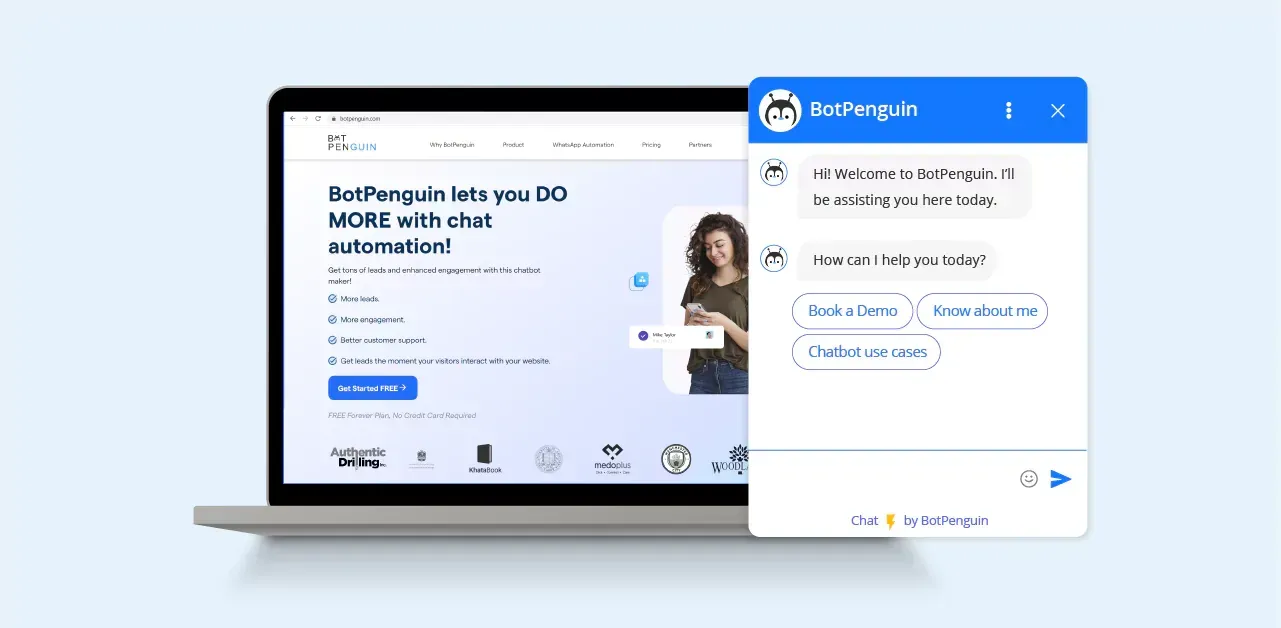
Generative AI and Data Security
While generative AI opens up exciting possibilities, it also raises serious questions about data security.
Since generative AI models require large amounts of data to learn from, they often deal with sensitive information. Security becomes paramount to ensure this data isn't misused or mishandled.
One concern is data privacy. If an AI is learning from personal data, like emails or health records, there's a risk of this information being exposed or shared. This could lead to serious privacy breaches.
Another worry associated with generative AI is its potential for creating deepfakes. These are fake images or videos that look startlingly real.
They're created by AI models that have learned to mimic real-life footage. This technology can be exploited to spread misinformation, fake news, or to carry out fraud.
Moreover, as AI systems become more complex and capable, there's also a risk of these systems being used to launch sophisticated cyber attacks.
An intruder could potentially use a generative model to mimic a user's behavior or to generate convincing phishing emails.
So, how can these risks be mitigated? Transparency in AI learning is crucial. Clear, understandable records of what data AI has access to and how it's using that data can go a long way. Additionally, using encrypted data can add an extra layer of security.
Regular security audits can help identify potential vulnerabilities. Staying vigilant and adopting good data handling practices is also key to ensuring data security in the age of generative AI.
While generative AI gives rise to challenges in data security, with the right precautions, its benefits can be harnessed while risks are kept in check. The dual focus should always be on harnessing the potential of AI while ensuring that data it learns from remains secure.
Suggested Reading:
Reshaping Education: Generative AI in Learning & Development
Debunking Common Myths About Generative AI
Generative AI has rapidly evolved in recent years, sparking both awe and confusion. With its growing influence, it's important to debunk some common myths and misconceptions that surround this technology.
Myth 1: Generative AI will take away human creativity
Truth: AI can undoubtedly create impressive art or music, but it doesn't replace human creativity. Instead, it augments and complements our own creative talents. It's a tool that helps us explore new ideas, identify patterns, and push the boundaries of our imagination.
Myth 2: AI-generated content is always perfect
Truth: Generative AI models can produce incredible content, but it's not always perfect. Since AI learns from data, it can only replicate patterns, themes, and techniques it has observed. Additionally, the quality of its output depends on the quality of data it learns from. Incomprehensible or nonsensical results can still surface.
Myth 3: Generative AI is inherently biased
Truth: AI models aren't inherently biased. However, they can and do learn biases from the data they're trained on. If a model is fed data containing biases, the AI will likely replicate those same biases in its output. Ensuring fair, balanced, and diverse data sets can help minimize this issue.
Myth 4: Generative AI can only create, not learn or adapt
Truth: Generative AI models are continually learning and adapting. They utilize a feedback loop, allowing them to refine their results based on previous outputs. It's this iterative process that enables AI to produce more accurate or visually appealing results over time.
Myth 5: Generative AI is harmful to the economy
Truth: Generative AI has the potential to disrupt certain industries and jobs, but it can also create new possibilities and opportunities. By automating mundane or repetitive tasks, AI frees up human workers to focus on more meaningful and creative work. Embracing AI technology can lead to greater efficiency, new forms of employment, and economic growth.
Debunking these myths is an important step towards fostering a responsible, informed attitude towards generative AI and guiding its use for the betterment of society.
Future of Generative AI
Generative AI is a rapidly evolving field, and its future is full of possibilities. Here are some potential advancements and implications for the technology:
Advancements
As generative AI continues to improve, we can expect more sophisticated models capable of generating more complex and diverse content.
Advancements may be made in creating more efficient and effective training algorithms, allowing for faster and more accurate training of generative models.
Advances in the interpretability of generative models also allow users to understand better and control the content generated.
Potential Breakthroughs
One potential breakthrough could be creating generative models to reason about the content they generate. This could enable models to generate content that is diverse, novel, and more coherent and meaningful.
Another potential breakthrough could be developing generative models that account for user preferences and feedback, enabling more personalized and tailored content creation.
Conclusion
Generative AI is an exciting field that offers significant potential in various industries, from art and design to medicine and healthcare.
Its ability to automate complex tasks and enhance creativity and innovation transforms how we approach problem-solving and content creation.
While the technology has significant benefits, it is essential to consider its ethical and societal implications. Guidelines and regulations must be established to ensure the responsible and ethical use of Generative AI.
We expect continued advancements and potential breakthroughs in Generative AI, leading to new and innovative applications.
As the field evolves, it's essential to remain vigilant about potential risks while leveraging the technology's capabilities to enhance and improve our lives.
Platforms like BotPenguin provide intuitive drag-and-drop chatbot builders for popular platforms like WhatsApp, Facebook Messenger, Website, and more. Our natural language processing is powered by advanced generative models like GPT-3 to enable human-like conversations.
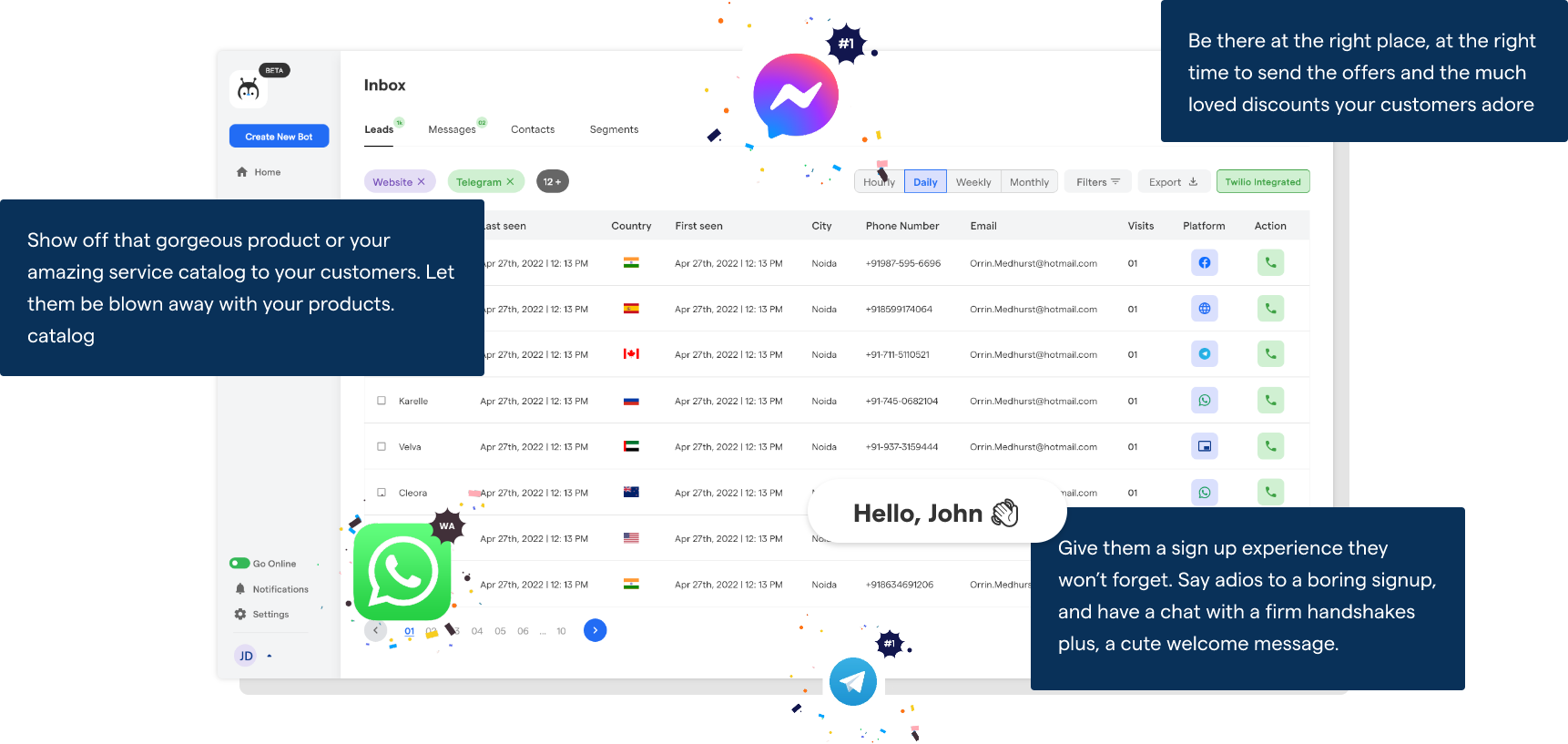
Frequently Asked Questions (FAQs)
What is Generative AI?
Generative AI is an approach to AI that focuses on creating or generating new and original content. It uses deep learning models to produce images, videos, music, or text that is similar to human-created content.
What are the benefits of Generative AI?
Generative AI can simplify complex tasks, automate creative processes, and unlock new creative possibilities. It can help designers, artists, and musicians generate novel and unique content while accelerating the iterative design process.
How is Generative AI being used today?
Generative AI is used in various fields, from art and design to medicine and healthcare. It can assist in drug discovery, create new and unique visual artworks, and even compose music and generate dialogue and narratives for video games.
What are the ethical concerns around Generative AI?
Using generative AI to create deep-fake videos raises questions about potential deception and misinformation. There are also concerns around copyright and intellectual property, as models can generate content that closely resembles existing works.
What are the implications of Generative AI on the workforce?
Generative AI has the potential to automate certain job roles or tasks, impacting industries that rely on manual and repetitive tasks. However, it also creates new opportunities and can complement human creativity, allowing professionals to focus on higher-level tasks.
What does the future hold for Generative AI?
As the technology continues to improve, we can expect more sophisticated generative models capable of generating more diverse and complex content. Advancements in interpretability and user control may also lead to more personalized content creation.